Stanford University’s 2022 Global AI Index found that ESG concerns are holding back AI adoption: concerns over how to be transparent with customers about what the AI will be doing (41%), the impact on corporate reputation (35%), the privacy impacts (41%) and how to avoid other broader ethical issues (29%).
Global approaches to guidance and governance of AI development are fraught with complexities. A combination of voluntary guidelines (e.g. the Australian Artificial Intelligence Ethics Framework, legislative frameworks (the EU’s new Artificial Intelligence Act), industry codes, and adjacent legislation (such as privacy laws, anti-discrimination laws), and yet, there is very little clear, comprehensive, practical guidance afforded to companies answering the one question everyone is interested in: how do I do it?
Into this mish mash steps the Said Business School at Oxford University, developing a comprehensive auditing model, called capAI, to provide “practical guidance on how high-level ethical principles can be translated into verifiable criteria”.
What can go wrong with AI?
Said’s study of 106 ‘big AI fails’ globally found that:
- 50% involved privacy breaches, either a failure to obtain consent or use of data for a non-authorised purpose;
- 30% involved algorithmic bias. But a significant proportion of these involved bias aligning or originating with the users: e.g. on a dating app, nominating a person of the same race. Said commented that ‘when [bias] involves users’ preferences, organisations face a reputationally more contested choice between reinforcing the existing bias in user preferences or choosing to take affirmative action to correct such biased preferences;
- 14% involved algorithmic opacity: why was my loan rejected? Why is AI confident this person will develop cancer?
What you do internally to ensure ethical AI
The capAI model establishes a framework for an organisation to develop its own Internal Review Protocol (IRP). The IRP should follow the key stages of the AI system lifecycle and operates primarily as a management tool for quality assurance and risk management:
The capAI lays out some traps to be avoided:
Design: When things go wrong, data scientists and managers may be tempted to ex-post rationalise AI’s performance or drift use cases on pressures to complete projects quickly, but the reality is more likely that not enough care and attention went into the design phase. First, don’t assume ‘you need to keep up with the Joneses on AI’: ‘[w]hile AI systems may outperform humans in decision-making across many domains, yet, it remains superfluous – even unsuitable – for many purposes.’ But more importantly, 'do the ethics upfront': the design phase ‘must include a definition of both organisational governance, [which] starts with a set of ethical values that steer the behaviour of developers and managers towards the good of society.’
Development: the ‘garbage in garbage out’ problem which bedevils any IT development carries much higher risks with AI because of the scale, scope and autonomy of decision making by AI once ‘let loose’ in your business. The capAI model addresses the risk through sequential ‘prepare’ and ‘train’ steps:
‘The prepare step concerns collecting the ‘right’ or ‘good quality’ data and transforming it with appropriate methods to ensure quality and compliance. Data quality covers criteria such as uniqueness, accuracy, consistency, completeness, timeliness and currency…After all, the statistical learning used by algorithms requires large datasets with appropriate attributes to make the correct inferences.'
‘The training step concerns all the tasks for ensuring the model produces reliable predictions. It includes tasks such as selecting features, training, validating and tuning the model. Tuning ensures that the algorithm is trained to perform its best; it uses all the available information to reduce uncertainty in the outcomes. This is an iterative process, and model versioning is suggested to explain differences in model performance and compare models (suggested to explain differences in model performance and compare models (e.g., through A/B testing).’
Evaluation: The capAI model identifies this stage as involving the biggest difference with traditional software development, because traditional software, apart from ‘glitches’, performs on objective facts as designed and coded, whereas AI is an ‘inference machine’. The model provides for two stages: test and deploy:
‘The test step aims to assess how the AI system performs on unseen data across a set of dimensions, such as technical robustness, and adherence to ethical norms and values. To that end, organisations should instrument AI to measure performance….Quantitative metrics alone are insufficient to assess AI systems. Therefore, developers should act as complementarities in reducing errors and biases, especially regarding input incompleteness.'
‘The deploy step ultimately concerns deploying a tested model into the production environment. To arrive at that point, data scientists first need to define the serving strategy and its impact on users’ privacy and security. Adopting pilots, such as canary deployment, minimise the risks of unforeseen failures.'
Operation: the capAI model identifies this phase as the largest gap in most business compliance processes governing AI. Most practitioners discount the importance of the actual operation of AI systems, but unmanaged AI will deteriorate over time. Again the operational phase is less of a risk with conventional IT systems because, once developed, they are fixed in their characteristics. But machine learning outcomes result from statistical inference rather than ‘ground truth’, and programmers spend less time monitoring, tracking changes and updating the model, as their efforts are put into automated, reproducible pipelines that take care of most of the updates when new data is available. The capAI model builds in two stages in the operational phase, sustain and maintain:
‘Sustain refers to all activities that keep the system working, such as monitoring its performance, and establishing feedback collection mechanisms. As users interact with the AI system, they might use it in ways that were unforeseen by the developers, producing errors that need to be resolved.'
‘Maintain refers to providing updates to keep the system running in good condition or improve it. This step involves defining regular update cycles and establishing problem-to¬-resolution processes.’
The external transparency you can provide on AI ethics
The final component to the capAI auditing system is an external scorecard (ESC). The ESC is a summary or overview document to be made available externally. It is a ‘health check’ to show the application of good practice and conscious management of ethical issues across the AI life cycle.
The ESC derives information from the areas covered by the IRP to develop an overall risk score for the AI system and is then distributable to customers and other stakeholders. Each element is summarised into a brief statement of the most directly relevant information.
Pros and Cons of an ethical auditing approach
The capAI model draws from the broader ESG world of ‘ethics-based auditing’ (EBA) applied to address other thorny issues, such as privacy. The paper engages in a comprehensive discussion of the benefits and risks of EBA. There are a few points worth affording some consideration here.
Clearly, there is a distinct human benefit to anticipating the negative consequences of a technology before they eventuate. By requiring an ethical impact assessment to occur so early in the process of development, a degree of "human suffering" may be relieved by the capAI model.
Holding an organisation deploying AI to account through an internal review and presenting it in a digestible way for all parties and stakeholders through the ESC, means all information is accessible and available. This benefits both stakeholders who can understand the technology they are investing in, and the company themselves who are able to anticipate and protect against potential failings. In this way, EBA facilitates economic growth by building trust in available technologies through procedural transparency and documentation, as well as clear explanations.
But Said also acknowledges that there are risks surrounding EBA, and these all run the risk of eventuating if we put too much pressure on companies to enact procedures that they do not have the resources or capacity to support:
- Ethics shopping: the practice of cherry-picking ethics principles, and thereby justifying pre-existing behaviours. The concept of ethics shopping is in recognition of the large number of ethical principles, codes, guidelines and frameworks that have emerged over recent years, which provide companies with an opportunity to “shop” for the kinds of ethics that are best ‘retrofitted to justify their current behaviours’ rather than meaningfully engaging with their conduct and reflecting on valuable changes to achieve an ethically sound practice.
- Ethics Blue-Washing: bluewashing involves making unsubstantiated claims with regards to the ethical behaviours of an organisation or system. This is a form of misinformation, concentrating on marketing, advertising, and other public relations activities to appear more digitally ethical than one is.
- Ethics Lobbying: the process of exploiting ‘self-governance’ to delay or avoid necessary legislation. In the process of ethical lobbying, private actors use self-regulation to lobby against the introduction of legal norms, to provide an excuse for limited compliance. This is particularly pertinent in the digital industry, where ethics is ‘exploited as…an alternative to legislation’.
Overall thoughts
This paper proposes an exciting prospect: a step-by-step guide to ethical compliance, actionable and comprehendible by users, consumers, and legislators alike. There are clear advantages to this system. It is comprehensive; it pushes past the rhetorical to provide a detailed set of guidelines and a practical list of steps from beginning to end, following all stages of the life cycle of AI; it is ethically guided and thereby accomplishes an admirable goal of driving trust and transparency in AI technologies.
However, the drawbacks of this approach cannot be discounted. A complex process like CapAI requires resourcing and labour, something individual companies may be neither willing nor able to provide. In the development of any new process, for governance purposes or otherwise, we must acknowledge and reflect upon the significant costs imposed upon businesses in implementing these procedures. Whilst capAI is certainly a commendable proposal, we must consider the reality of its application, and a new question is raised: how can a system like capAI be effectively supported in a company? How can SMEs efficiently implement a system like capAI if that is what is needed to open the door to AI in their business? What level of external support can be provided and what would that look like? Can research or industry bodies develop tools or case studies to mitigate the need for companies to build and implement capAI on a standalone basis?
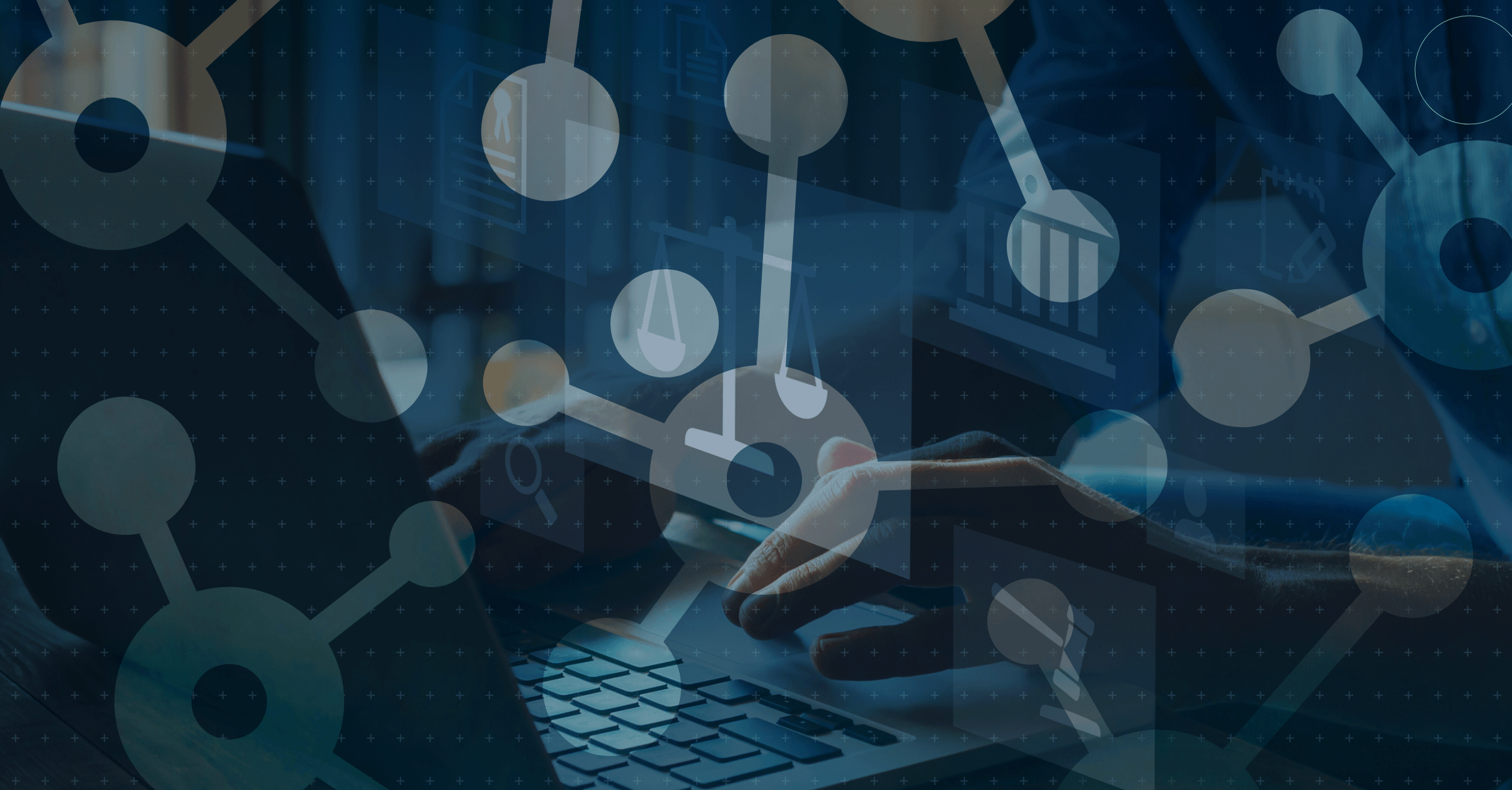
Visit Smart Counsel