The UK’s Centre for Data Ethics and Innovation (CDEI) has released its second annual ‘barometer’ on the state of AI in the UK.
What’s holding us back?
The survey identified the following barriers to AI adoption:
Amongst the ‘usual suspects’ (such as lack of funds and competing demands for those funds), there were three interesting trends.
First, there appears to be a widening gap between adopters and non-adopters of AI. One of the clearest barriers to adoption of AI amongst current non-adopters was the limited benefits of using such technologies in their business. Reflecting the certitude of their view about this, over three quarters of them reported no plans to invest AI in the foreseeable future. The CDEI politely commented that:
“The survey results suggest a lack of intention to introduce data-driven technologies correlates not only with being less likely to see the benefit in such technologies, but also with factors such as: seeing less of a need to improve data collection and management processes; less of a need to improve understanding of related legislation and regulation; less need to invest in the workforce to explore opportunities offered by data-driven technologies; and much less need to establish new collaborations on data-driven and AI projects.”
Second, businesses expressed concern about the adequacy of their internal governance processes to handle ethical issues arising from AI. The level of concern amongst would-be adopters was over a third, but even amongst those currently using AI, one in four expressed similar concerns. A further 70% noted the need for additional legal guidance on what businesses are allowed to do in collecting, using, and sharing data. Even more strikingly, this rose to 78% amongst businesses which already have extensively deployed AI.
Third, AI needs to ingest vast ‘data lakes’ to learn and evolve, but there seem to be problems making this happen on the ground. Amongst AI vendors:
- 58% identified fragmentation of data across public and private data sources used in training their AI;
- 56% identified a lack of skills amongst customers in how to collect and manage data; and
- 50% identified poor or incomplete data, including lack of digitalised historical data and problems of data ownership.
Businesses using AI mainly rely on their own internally collected data (84%), although 60% have one alternative data source to ‘feed’ their AI, mostly from collaborators or partners, followed by open source data and public sector data.
Data concerns amongst businesses using AI are very similar to those of vendors. Nearly 75% had concerns about data fragmentation and nearly half had concerns about poor quality data (remember they are mainly using their own internal data). Just under three fifths said that data challenges were related to the lack of internal skills to deal with data issues – and this is amongst businesses already using AI!
While nearly 90% of businesses thought they had good privacy and data storage practices, less than half thought they had well developed practices to identify bias in AI decision making and less than 20% had the ability to develop synthetic data sets (which is a way of preventing bias creeping in) to balance out where there was not enough natural data.
AI in HR
98% of Fortune 500 companies already use AI in their recruitment processes. But with more remote working as a result of COVID, the use of HR AI has expanded into other areas, such as ‘workplace monitoring tools’ to detect employee activity (e.g. keystroke logging), as well as more advanced applications that claim measurement of employee productivity (e.g. based on their use of email and other software) or wellbeing (e.g. based on inferential biometrics/’emotion detection’).
The CDEI’s industry panel observed that the risks of data-driven technology in the HR are not borne equally among its different actors. While there can be significant legal risks for developers and employers, most direct risks are borne by applicants and employees and they generally wield the least influence in the design and deployment of the systems, meaning design decisions are less informed by those directly experiencing many of the risks.
The opportunities for HR AI identified by the CDEI as being of the greatest perceived benefit but the hardest to achieve would primarily benefit workers (compared to employers or recruiters). However, this presents significant conceptual and technical challenges in the design of HR:
“for example, training a CV scoring system to ‘read’ and value different forms of qualifications and experience of people from diverse backgrounds is likely to require both appropriately labelled data, and appropriate methodologies for labelling such skills and experience in a way that truly reflects applicants’ capabilities. Similarly, use cases that seek to infer employees’ wellbeing or improve staff retention involve quantifying complex and subjective states that may not generalise well across workforces - for example, using sick leave data to understand staff engagement may fail to appropriately account for the impact of chronic illness or disability.”
The CDEI also expressed concern over the so-called ‘gamification’ of certain work types. This involves performance monitoring technology which directly feeds back into workers’ environments and task allocations: for example, some warehouse contexts incentivise workers to beat picking times and use that data to optimise future efficiency targets (usually upwards), and similar mechanisms are common in gig economy app platforms, where worker ratings or more desirable work assignments are distributed based on performance.
AI in logistics
The CDEI observed that the applications of data-driven technology across this sector are some of the broadest and most advanced in any sector of the economy, but this had mainly occurred within individual supply chains, such those of major supermarkets. Substantial additional benefits of AI – chief amongst which were climate change benefits – require system-wide solutions for all transport businesses across the rail, road, air and shipping networks. For example, there is a growing market of Mobility as a Service (MaaS) providers whereby different transport systems are linked up to enable journey and delivery planning over the system as a whole.
Yet transport sector wide integrated AI also carries its own challenges:
- Many of the major benefits offered by technology in the transport and logistics sector are spread diffusely across many others (e.g. improving air quality), meaning incentives may not be aligned between developers and users of technology, and bodies responsible for regulatory or environmental outcomes.
- Given the critical nature of transport, integrating individual systems together creates more ‘attack surfaces’ for cybersecurity risks.
- Many transport and logistic contexts such as road transport and rail system management are safety-critical. Decisions need to be able to ensure the right level of human control when systems malfunction or don’t understand, but this then creates challenges in switching decision-making over to slower human processes, in some instances making it impractical for a human operator to meaningfully intervene in time.
- The risk of data monopolies, where a single actor within a system holds an unparalleled market advantage due to the data they hold and are able to systematically collect. The CDEI panellists highlighted that in rail, contractual arrangements have sometimes resulted in public bodies missing out on the benefits that analysis of their datasets has provided contractors (e.g. around optimising scheduling).
AI in education
To date, most of the focus in ‘EdTech’ has been on ‘teacher-facing’ technologies which seek to alleviate administrative and pedagogical pressures on educators and on ‘system-facing’ technologies which can augment administrators’ and planners’ decision-making capabilities, for example in delivering effective inspection.
However, the CDEI saw the biggest opportunities lie in ‘learner-facing’ systems which offer opportunities to increase levels of personalisation in learning pathways. The OECD has given the following examples:
“Providing all students with a more inclusive access to education has been a persisting challenge for most countries, even more so in less affluent countries... AI systems have already shown their effectiveness to help students with disabilities…. For example, wearables using AI can help visually impaired students to read books and recognise faces, and thus to learn and socialise within their communities….Powered by AI, technologies such as augmented and virtual reality (AR/VR) and robotics support the learning and engagement of students with health impairments and mental health issues.”
Although the CDEI did not put it this way, the biggest challenge for ‘learner-facing’ systems is that many of us put our success down to a single good teacher – how can that very human, personal experience be replicated by a machine?
The CDEI industry panel criticised much of current EdTech as being focused around replacing aspects of teaching, rather than augmenting and scaling the capabilities of teachers. EdTech tended to automate the easiest (but not necessarily the most effective) approaches to teaching, with the risk of automating poor pedagogic practices as they are integrated into education environments.
The CDEI panel also expressed concern about the rigid use of predictive analytics in AI, such as estimating a learner’s attention or engagement levels, given that consequences of these decisions on learners’ lives can be very significant, both individually and cumulatively. Beyond the ‘standard’ concerns about bias, EdTech would need to grapple with broader issues of ‘fairness’ and opportunity in education – how to leave room for that instinctive sense in a teacher that a failing student has it within themselves to succeed with the right encouragement.
Conclusion
The CDEI’s barometer has three big take-outs:
- not all opportunities presented by data-driven technology are equal, but we need to trust that, when exposed to AI decision making, we will be treated equally;
- the highest benefits of AI are also the hardest to achieve, and are the most unlikely to be realised without Government regulation and policy intervention; and
governance challenges in understanding how high-level principles apply in specific sectoral or application contexts means businesses are less willing to make full use of data and data-driven technologies due to the risk of non-compliance or potential reputational damage from doing harm.
Read more: AI Barometer 2021
KNOWLEDGE ARTICLES YOU MAY BE INTERESTED IN:
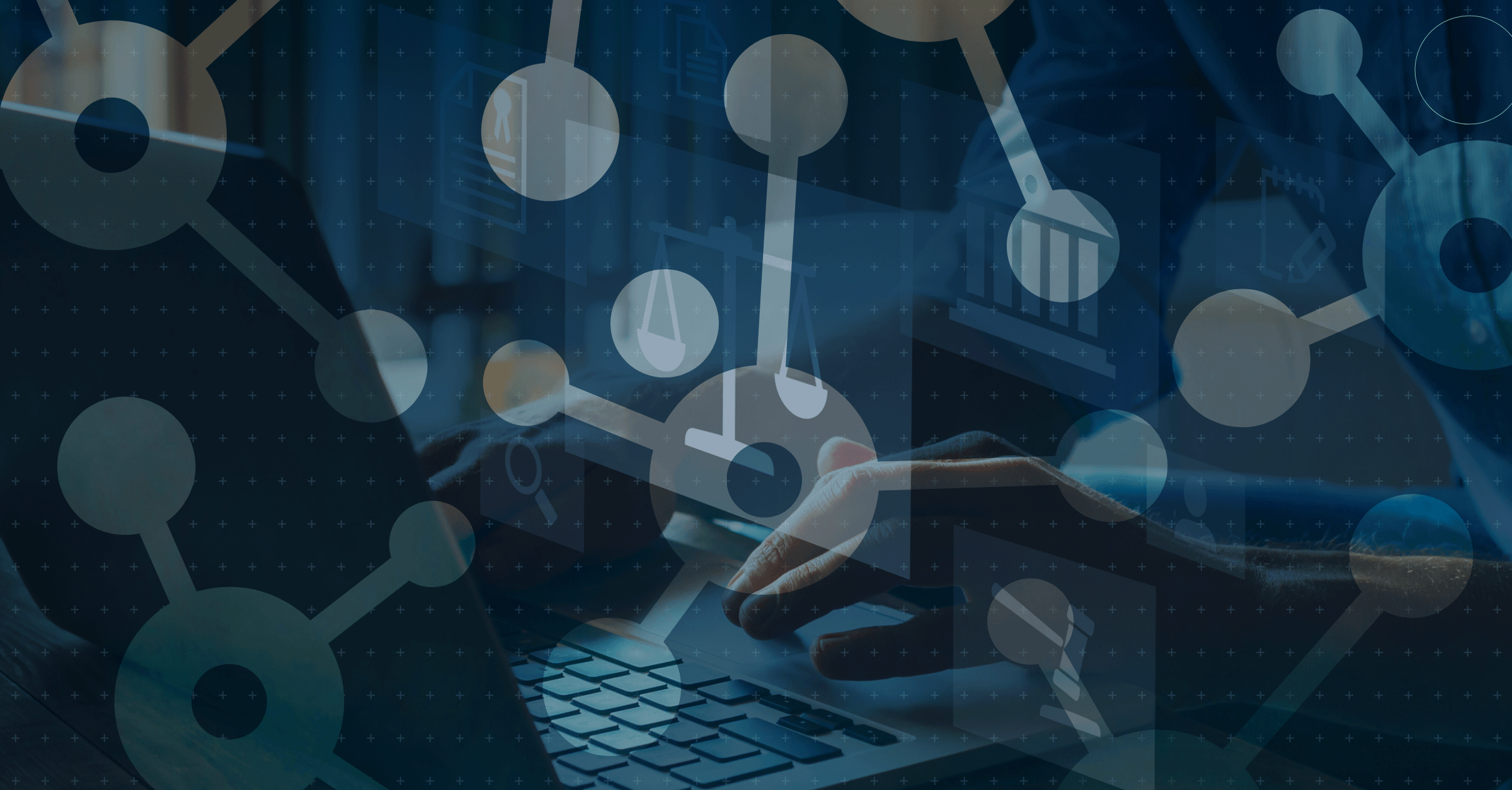
Visit Smart Counsel