The British National Health Service (NHS) and the Ada Lovelace Institute have recently announced the world’s first methodical assessment tool for AI used in healthcare, called an algorithmic impact assessment (AIA).
The problem being addressed
Use of AI in healthcare has seen numerous benefits from improving the accuracy of pathology diagnoses, to automating radiology scans by “learning” certain images over time. Already AI systems have saved clinicians time and resources that can be repurposed towards other tasks, enabled hospitals to save money and provided larger collections of data from a range of sources that may assist research efforts and the analysis of many illnesses.
However, alongside these many benefits, come some risks. What a machine “learns” may not always be completely accurate and will often require the monitoring and input of clinicians. There have been concerns around the overreliance or misplaced faith in the accuracy metrics of AI systems, as well as these systems making decisions that require human judgment.
Other factors that may have an impact on the effectiveness of an AI system include human subjectivities, such as biased or racist attitudes which can become implemented into AI systems reinforcing oppressive systems.
More mundane factors also can distort the effectiveness of AI in a health setting. One study on the development of an AI retinal scanning tool used in Thailand found that the success of the AI tool depended on various socio-environmental factors such as whether the hospital had stable internet and good lighting conditions.
What is an AIA tool?
One way to mitigate these risks is to conduct an AIA prior to the design and development of an AI system. An AIA is a tool used to assess the possible societal impacts of an AI system before the system is in use (although ongoing monitoring of the system is advised).
The NHS has recently announced that it is launching a world first trial into AIAs in healthcare using the model developed by the Ada Lovelace Institute, an independent British research institute specialising in AI and data. Companies who wish to access data held by the NHS AI Lab’s National Medical Imaging Platform (NMIP) for any AI system they are developing will be required to conduct an AIA. The NMIP collects medical-imaging data from across the NHS and make it available to companies and research groups to develop and test AI models. The NMIP Data Access Committee (DAC) is used as a forum for holding developers accountable throughout the AIA process and will also ultimately decide who is able to access the NMIP.
How does the Lovelace AIA work?
The Ada Lovelace Institute’s report setting out its recommended methodology (the Report) recognises at the outset that AIAs are heavily context specific and have different concerns depending on their objectives and the data used, and that therefore it is difficult to achieve unity between them. However, the Report says that the starting point is to define a common set of goals that communicate the purpose for which all AIAs are conducted:
- Accountability: The proposed AIA process remains accountable through the engagement of not only external decision makers, but by engaging external stakeholders, such as clinicians, members of the DAC and general members of the public. During the process, patients and clinicians are provided the opportunity to provide their specific expertise and insight into the decision. The Report noted that this would involve a substantial shift in mindset, and power relationships, in the health sector which is traditionally dominated by health professionals:
“Most AIA processes are controlled and determined by decision-makers in the algorithmic process, with less emphasis on the consultation of outside perspectives, including the experiences of those most impacted by the algorithmic deployment. As a result, AIAs are at risk of adopting an incomplete or incoherent view of potential impacts, divorced from these lived experiences.”
- Reflection and reflexivity: The proposed AIA process aims to prompt reflection from AI developers, as well as elicit discussions with potentially affected individuals as to how the design and development of an AI system may result in certain benefits, as well as certain harms to society. By encouraging this reflection and open dialogue, the proposed AIA process ensures that applicants continue to think “reflexively” - that is, examine and respond to their own practices, motives and beliefs – while they undertake the research and development of the AI system. By involving a wide range of perspectives, the process also allows for a critical and thorough AIA which aims to identify and remove individual biases. The Report probably sees a lack of reflection as the biggest shortcoming of current health AI development, yet the most important building block to the success of health AI.
- Standardisation: The proposed AIA process uses a standardised approach and language that is easily understandable to a wide range of stakeholders, allowing applicants and other individuals involved in the process to fully engage with the task. The AIA provides a standard template document, which allows decision makers to more easily compare different applications.
- Independent scrutiny: The proposed AIA process also allows external stakeholders to assess the AIA and call out any potential issues with the process itself via a participatory workshop. The independent scrutiny provided by these external stakeholders allows for greater accountability as well as providing a wider forum for judgment and deliberation of the process.
- Transparency: The proposed AIA process focuses on being transparent through both internal as well as external visibility. This is achieved by applicants documenting their full AIA process as well as making their results publicly available (such as on the NMIP website), providing regulators as well as members of the public valuable insight into the UK healthcare context. This involves much more than telling other stakeholders what the medical AI is designed to do:
“This differs to making transparent details about the AI system and its logic – what has been referred to as ‘first-order transparency’. This AIA aims to improve transparency via both internal and external visibility, by prompting applicant teams to document the AIA process and findings, which are then published centrally for members of the public to view.”
The Report says that these goals will be accomplished using two principal approaches within the AIA process: documentation and participation. The principle of documentation includes maintaining thorough recordkeeping throughout the AIA process, permitting applicants to more easily consider their internal process and practices and engage in reflexivity throughout the process.
The principle of participation enables a wider range of perspectives throughout the AIA process, providing alternative sources of knowledge to the development of the system, including individuals own lived experiences, as well as a more independent and unbiased review of the impacts of an AI system. Crucially this depends on an enabled, informed external forum of stakeholders:
“The definition that is most helpful here [is] a depiction of the social relationship between an ‘actor’ and a ‘forum’, where being accountable describes an obligation of the actor to explain and justify conduct to a forum. An actor in this context might be a key decision-maker within an applicant team, such as a technology developer and project principal investigator. The forum must have the capacity to deliberate on the actor’s actions, ask questions, pass judgement and enforce sanctions if necessary.”
The Report assembles the above building blocks into a seven-step process that applicants must follow to access NMIP data:
Conclusion
As important as it is to appreciate the numerous benefits of AI, it is equally important to acknowledge its risks, and the potentially harmful impact it could have on society. Balancing benefits and risks lies at the heart of most medical advances, but there are well developed, trusted and supervised processes for this balancing exercise in drug development and medical devices. Without a rigorous, trusted process in place for medical AI in which the public can have trust, there is a fear that the risks of AI will dictate limits on its use in the healthcare industry. AIAs allow for – or perhaps force - developers to plan and consider the benefits and risks in the early stages of the development of AI systems, allowing for a less unpredictable and more pragmatic approach to this new frontier.
Read more: Algorithmic impact assessment: a case study in healthcare
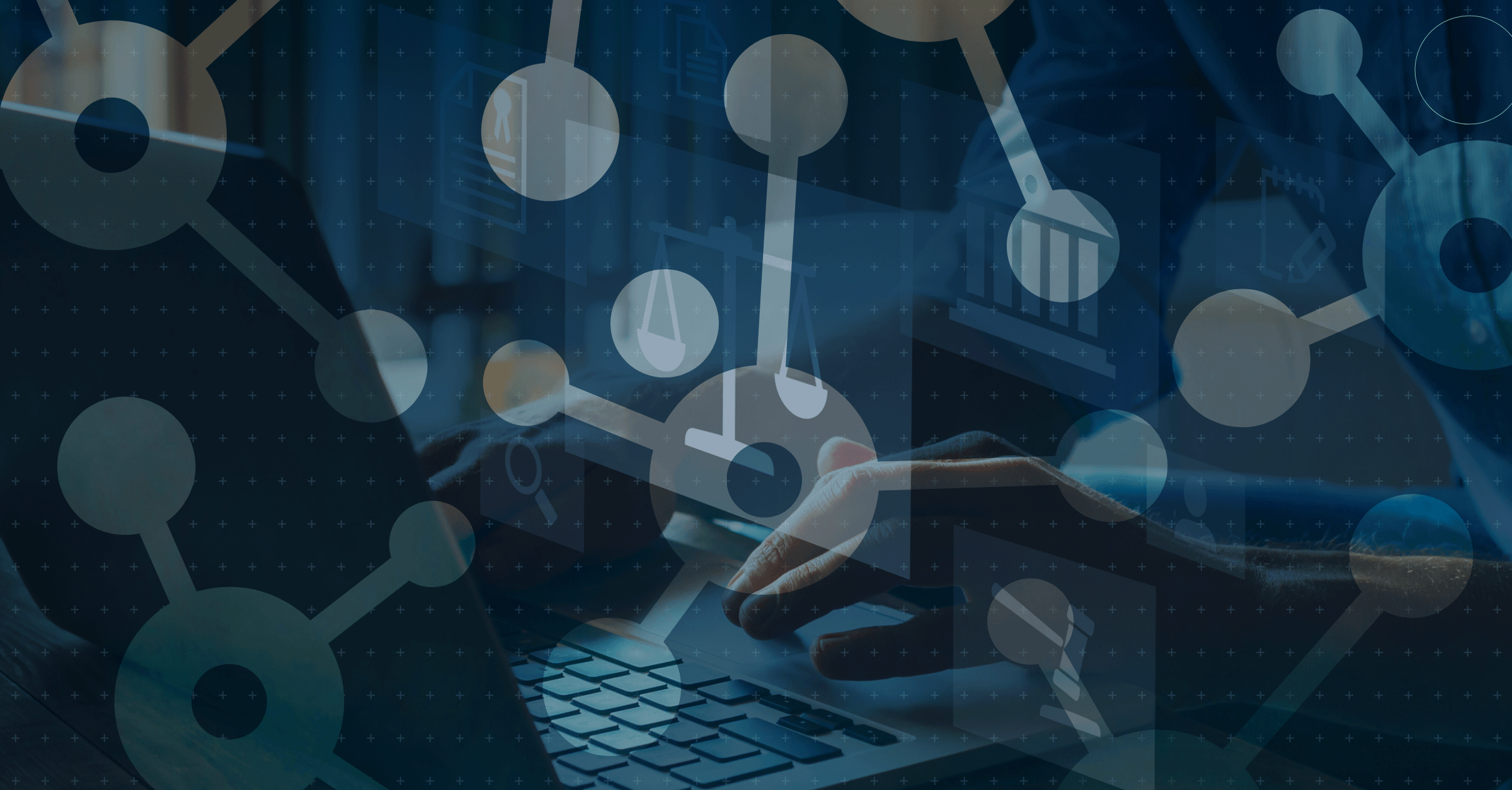
Visit Smart Counsel