Nearly half of Australians believe AI will harm them – one of the highest distrust levels in the developed world (see our previous article - What does it take for Australia to win in a post-COVID world?). The ACCC has launched a string of court cases about algorithms (Google, Trivago and HealthEngine), built around a broad theory of ‘unfairness’. Is this the right tool to address public trust of AI?
Not according to the UK’s Centre for Data Ethics and Innovation, which last week released a landmark review of bias in AI – really, it's about how to ensure the same question of fairness.
The CDEI starts with the truism that “algorithms, like all technology, should work for people, and not against them.” More telling is CDEI’s warning that, with COVID’s rapid acceleration of digital transformation, we only have a small window of opportunity to get this right before AI takes off.
CDEI makes four big points.
First, don’t view AI through a simplistic lens of ‘human decision-making fairer: AI decision-making riskier’. Human decision-making has always been flawed, shaped by individual or societal biases that are often unconscious. Therefore, “the issue is not simply whether an algorithm is biased, but whether the overall decision-making processes are biased…[l]ooking at algorithms in isolation cannot fully address this.” In fact, good use of data can enable organisations to shine a light on existing practices and identify what is driving bias.
Second, algorithms have different but related vulnerabilities to human decision-making processes: “[t]hey can be more able to explain themselves statistically, but less able to explain themselves in human terms…[t]hey are more consistent than humans but are less able to take nuanced contextual factors into account.”
And most importantly, there is the sheer scale and breadth of both individual and collective harms potentially generated by AI trained on data that embeds historic inequalities and patterns of behaviour and resource.
The key challenge is how to build fairness into an algorithm. As CDEI comments:
“If we want model development to include a definition of fairness, we must tell the relevant model what that definition is, and then measure it. There is, however, no single mathematical definition of fairness that can apply to all contexts. Ultimately, humans must choose which notions of fairness an algorithm will work to, taking wider notions and considerations into account, and recognising that there will always be aspects of fairness outside of any statistical definition.”
One way of ensuring the AI is ‘built fair’ is to have diversity in the workforce designing and approving the AI. The CDEI gives the example of two possible ways of measuring gender fairness in credit approval AIs: the probability of men and women getting a loan approval; or the probability of men and women on the same income getting a loan approval. As only 79% of UK IT professionals are men, the second criteria of fairness may be adopted without much thought, but that would ignore the entrenched income disparities between men and women.
Designing AI to be fair also requires "understanding of, and empathy for, the expectations of those who are affected by decisions, which can often only be achieved through the right engagement with groups.”
Once AI is implemented, AI fairness also requires checking of actual outcomes. The CDEI found that many government and business users of AI were under the mistaken legal view that they could not collect data about ‘protected status’ (e.g. gender and race) to work out if their AI was discriminating on those basis:
“..discrimination as part of the decision-making process, protected characteristic attributes should not be considered by an algorithm. But, in order to assess the overall outcome (and hence assess the risk of indirect discrimination), data on protected characteristics is required. if data being analysed reflects historical or subconscious bias, then imposed blindness will not prevent models from finding other, perhaps more obscure, relationships.”
Third, Governments bear a special duty of care in using AI because “when the state is making life-affecting decisions about individuals, that individual often can’t go elsewhere.” CDEI recommends a mandatory transparency obligation on all public sector organisations using algorithms that have a significant influence on significant decisions affecting individuals to proactively publish information on how the decision to use an algorithm was made, the type of algorithm, how it is used in the overall decision-making process, and steps taken to ensure fair treatment of individuals.
Fourth, given the escalating speed and scale at which AI is adopted, existing regulatory approaches are too slow to respond to the new ways algorithms are already impacting people's lives. This requires a shift to pre-emptive action by government and corporate decision-makers, from the earliest point in the technology innovation cycle and right through its deployment. This ‘anticipatory governance “aims to foresee potential issues with new technology, and intervene before they occur, minimising the need for advisory or adaptive approaches, responding to new technologies after their deployment.”
The CDEI also cautions that “[b]ias mitigation cannot be treated as a purely technical issue; it requires careful consideration of the wider policy, operational and legal contexts.” The CDEI says boards need to come to grips with the following issues:
- understanding the capabilities and limits of those tools.
- considering carefully whether individuals will be fairly treated by the decision-making process that the tool forms part of.
- making a conscious decision on appropriate levels of human involvement in the decision-making process.
- putting structures in place to gather data and monitor outcomes for fairness.
- understanding their legal obligations and having carried out appropriate impact assessments.
Given the current profile of many boards, this is likely to be a challenge – but not one that cannot be avoided for much longer.
Read more: CDEI publishes review into bias in algorithmic decision-making
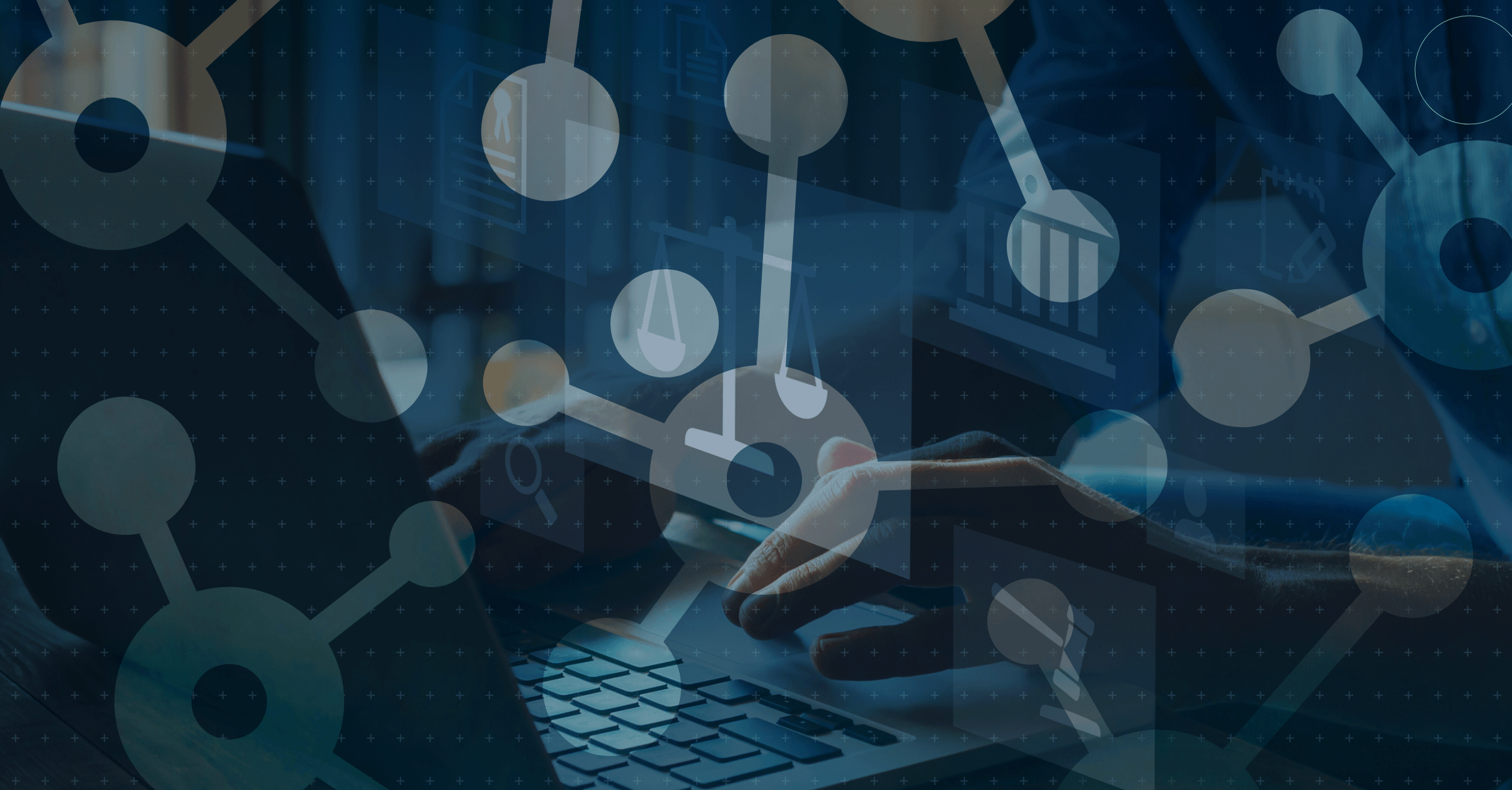
Visit Smart Counsel