The 2023 Bletchley Park AI summit established an international panel of 96 AI experts to provide objective, scientifically based reports on AI safety to inform annual global summits of policy makers, emulating the approach of the International Panel on Climate Change and the COPs.
The first full report, at 300 pages, landed in late January 2025 as pre-reading for the Paris AI Summit which was held in early February. Over the next three weeks we will review the report’s analysis of three core questions: What can general-purpose AI do? What are risks associated with general-purpose AI? And what mitigation techniques are there against these risks?
What AI is good at
The following graph illustrates the accelerating pace at which AI can achieve performance levels which meet or exceed humans.
The report makes the following points:
As AI models have become larger and more sophisticated, AI can now achieve or better human performance within a matter of months.
This makes assessing the current AI capabilities a moving target, illustrated by the release between the report’s finalisation in December 2024 and its publication in January 2025 of Open AI’s o3 model which shows striking advances in abstract reasoning (see below).
AI’s capabilities consistently surpass the expectations of AI experts, including on his own candid admission the panel chair, Professor Yoshua Bengio.
On some tests, AI is beginning to materially outperform human experts. There was a counterview that AI capabilities would plateau at, or marginally above, human performance, which seemed logical because AI learns correlations within the four corners of the human-created knowledge on which it is trained.
AI has mastered working across multiple modalities, both on the input (prompts) and outputs (responses) sides:
Some AI models, including GPT-4o and Gemini 1.5, can process audio in much the same way as text, answering questions about the contents of an audio clip (for example, a spoken conversation). They can create high quality conversations mimicking an individual’s voice with as little as a three second recording of that person speaking.
Some AI models can take video as input and analyse its contents, such as V-JEPA, Gemini 1.5, GPT-4o, and Qwen2-VL, in much the same way as searching text. They can be asked to locate key moments or pieces of information in a video or produce text summaries of what they have ‘seen’ in the video. Conversely, some AI models can also generate realistic, high-definition video from text prompts, for example Sora and Movie Gen.
As AI capabilities exponentially increase, the costs of running AI models at a given capability level has fallen by multiple orders of magnitude. For example, in 2022 it cost users ~US $25 to generate a million words using GPT-3, but by 2023 this fell to almost US $1 using the performance-equivalent Llama 2 7B.
What AI is not so good at
The report identifies the following current limitations of AI:
Current AI models often demonstrate uneven performance, excelling in some domains while struggling in others.
AI models can struggle to cope with novel scenarios, can be overly influenced by superficial similarities, and can be easily thrown off by irrelevant information. While AI models are mathematical whizzes, studies show that inserting spurious data into a prompt which humans would soon identify as a distraction can reduce a model’s accuracy by 17.5%.
When AI systems are given problems that require more steps of reasoning to solve, their error rate increases faster than would be expected if they had a constant error rate per step, which suggests that they are not reliable in solving complex problems. Relatedly, while better than humans at finding the short, quick answer to a problem, AI also materially underperforms humans on problems which take longer times to solve, such as complex multiday engineering solutions.
AI systems have also been shown to sometimes fail at reasoning on seemingly simple tasks. For instance, a model trained on data including the statement ‘Olaf Scholz was the ninth Chancellor of Germany’ will not always be able to answer the question ‘Who was the ninth Chancellor of Germany?’.
AI models perform worse on rare or more difficult variants of tasks that are not seen in the training data.
It is unclear how AI’s impressive performance on benchmarks translates into performance in real-world tasks, where the context, issues and challenges are messier, nuanced, novel and unpredictable. AI systems cannot yet effectively control physical robots or machines to perform many useful tasks such as household work, because the integration of general-purpose AI models with motor control systems remains a challenge. However, rapid advances have been made in AI agents which can autonomously make plans, perform complex tasks and interact with their environment by controlling software and computers, with little human oversight.
As the report sets out, there are two schools of thought on these AI’s limitations say about AI:
Some AI experts say that AI still lacks the deep conceptual understanding and abstract reasoning capabilities of humans. Hence, AI is not good at addressing novel problems not included in their training data because models partly or fully rely on memorising patterns rather than employing robust reasoning or abstract thinking.
Against this view, other AI experts point to studies which demonstrate that large language models (and systems built with them) have performed well on reasoning and mathematics problems whose solutions were not part of their training data, including to reach medal-level performance at the recent International Olympiads for mathematics. Some experts also argue that, unpredictably or inexplicably, when AI models get to a very large size they will begin to display the ability to act in ways that were not explicitly programmed or intended by their developers or users, such as compose music (called emergent capabilities).
Open AI’s new o3 model released this year may shift thinking on this issue. In an update note in the report, the panel chair Prof. Benigo says of the early test results of o3:
These results indicate significantly stronger performance than any previous model on a number of the field’s most challenging tests of programming, abstract reasoning, and scientific reasoning. In some of these tests, o3 outperforms many (but not all) human experts. Additionally, it achieves a breakthrough on a key abstract reasoning test that many experts, including myself, thought was out of reach until recently.
Can AI performance continue accelerating?
The report comments that such is the uncertainty around whether and how the current limitations of AI can be solved that:
In the coming months and years, the capabilities of general-purpose AI systems could advance slowly, rapidly, or extremely rapidly..[b]oth expert opinions and available evidence support each of these trajectories.
In particular, there is disagreement between AI experts over whether the so-called scaling laws will ‘keep on keeping on’. The scaling laws hold that there is a systematic relationship between an AI model’s size and its performance: essentially, more training data + more compute power to process that data + more parameters and honed weights from that training = more capable AI models, in an apparently endless upwards spiral.
As the report observes, scaling laws have proven robust so far: the average amount of compute used to train machine learning models currently doubles every six months and training processes now use roughly 10 billion times more compute compared to state-of-the-art model training in 2010.
However, the report identifies concerns the scaling laws may run out of steam:
The costs of building new AI models are enormous, reaching over US$1 billion per model. While the amount of computing power that AI companies can buy with a dollar is increasing at a rate of 1.35x per year, this is outpaced by the total compute required for training, which has increased by approximately 4x per year since 2010.
AI training requires massive amounts of energy, with global consumption similar to that of Austria or Finland by 2026.
Training AI systems across a very large number of AI chips is difficult, which may prevent extremely large training runs. For example, some estimates suggest training runs that are 10,000 to 10 million times larger than GPT-4’s will be impossible due to constraints on how much information can be moved between chips, and limits on the time to process data.
There is likely enough training data for scaling until 2030, but projections are highly uncertain after this point. Training data requirements have grown around 10x every three years. For example, a state-of-the-art model in 2017 was trained with a few billion words, whereas state-of-the-art general-purpose models in 2023 were trained with several trillion. A new potential source is on multimodal data, combining textual, visual, auditory, or biological information: the report says there could be enough multimodal data to support training runs a thousand to ten million times larger than GPT-4’s in terms of compute size. Machine-generated synthetic data also could dramatically alleviate data bottlenecks, but evidence for its utility is mixed.
While recognising these difficulties, the report thought that there were emerging more nuanced or modified approaches to relying solely on the brute force of scaling laws:
Algorithmic improvements: the computational efficiency of AI techniques for training has been increasing by 10x approximately every 2–5 years. For example, the amount of compute required to train a model to achieve a set level of performance at image classification decreased by 44x between 2012 and 2019.
Fine-tuning: involves downstream training of an AI model to optimise it for a specific application or make it more useful. Finetuning generally requires less than 1% of the computational resources to implement than was used for training the model, resulting in an improvement in the model’s capabilities approximately as much as would be expected from 5x more resources in training the base model.
Inference-time scaling: encodes an AI model to resolve a problem in a more structured, step-by-step way, called ‘chain of thought’. Requiring an AI model to, as it were, ‘think out aloud’ appears to result in more accuracy and better performance. However, inference scaling may be more revoluntary. The early tests of the o3 model suggest that this approach may also substantially improve AI’s cognitive abilities, breaking through the barrier of comparative human performance that many thought was out of reach. The drawback of interference-time scaling is that the AI model consumes substantially more computing power in deployment as it ‘cogitates’ on the response to each prompt from a user.
The DeepSeek model was released as the report was being published and is not addressed at any length in the report. DeepSeek’s ‘mixture-of-experts’ approach would seem to upend the scaling laws by producing an AI model with capabilities equivalent to or better than models trained using much more computing resources. However, this approach has been described as ‘more evolutionary than revolutionary’. See more here.
DeepSeek splits its AI model into submodels each specialising in a specific task or data type, with a load bearing architecture to dynamically shift tasks from overworked to underworked submodels. This means that only a fraction of its over 600 billion parameters are engaged at any one time in processing each chunk of input text (token), requiring less compute. Inference-time scaling is also used to ratchet up or down the compute being applied based on the complexity of the task.
Conclusion
The panel concludes that today’s data intensive, AI development and training methodologies are likely to remain feasible until 2030, although the pace of the resulting increase in AI performance capabilities is highly uncertain. The panel believes that beyond 2030, more fundamental, as yet unknown breakthroughs will be needed. However, it is unclear whether from the chairman’s postscript on o3 and DeepSeek the breakthroughs may have come much earlier than anticipated.
Read more: International AI Safety Report 2025
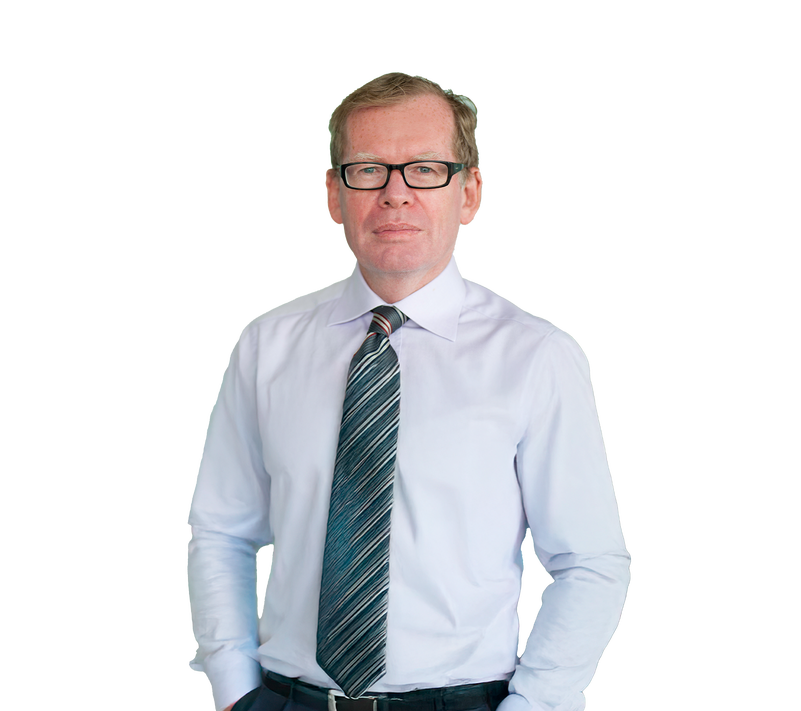
Peter Waters
Consultant