The debate
The US Copyright Office’s review received over 10,000 submissions, with opinions sharply divided:
The stakes are high and the consequences are often described in existential terms. Some warn that requiring AI companies to licence copyrighted works would throttle a transformative technology, because it is not practically possible to obtain licences for the volume and diversity of content necessary to power cutting-edge systems. Others fear that unlicenced training will corrode the creative ecosystem, with artists’ entire bodies of works used against their will to produce content that competes with them in the marketplace.
Does AI training prima facie infringe copyright?
One of the most valuable parts of the report is the gathering of data about the extent to which copying occurs in the process of AI training. Most submitters to the US Copyright Office’s Notice of Inquiry – developers and creators alike – agreed that the process of preparing the vast data pool on which AI is trained unavoidably involves copying of the training materials by developers, usually many times over.
A developer makes electronic copies when they download a work, such as by using stream-ripping software to download millions of video or subtitle files from YouTube. They then go on to make modified versions when filtering and cleaning the data to remove elements unhelpful to training (such as ‘read more’ links). They also copy over those modified versions into a compilation which pulls together the different sources from which data has been harvested (for example, internet data, Wikipedia and databases like Books3 are pooled).
The vast net cast by developers to gather data, inevitably captures copyright works and so reproduction of copyright works is almost unavoidable. Developers also can ‘up-sample’ to configure the sampling process to select examples from some subsets of training data more often than others, resulting in greater representation in the final compiled dataset. As training on higher quality data can improve the accuracy of AI models, copyright works may have a greater importance in training than may be indicated by their representation in the originally downloaded data pool.
The process of training the AI also involves copying of works. Works or substantial portions of works are temporarily reproduced as they are shown to the model in batches. This temporary copying will be repeated over multiple training runs.
Where developers and creators fundamentally disagree is whether the end result of training – the parameters and weights of the model – embodies unauthorised copies of the training data. The developers argue that AI models cannot be reproductions or derivative works because they do not contain training examples. After the temporary copying involved in training, the training data is discarded and what is left is just a large string of numbers that reflect statistical relationships among the training tokens.
However, AI has a puzzling ability to produce outputs in response to a user prompt which are substantially similar to individual training inputs. This is called ‘memorisation’. There are a range of theories about why memorisation occurs: one common theory is that there may be so many images of Darth Vader spread across the internet that the statistical relationship retained by the AI is not a generic representation of all space villains but is specifically of Darth Vader.
The developers argue that memorisation is rare and is a bug, not a feature of AI. However, the Copyright Office thought that if an AI model can produce a substantially similar copy of a training example without that expression being provided externally in the prompt, logically the copyrighted work must exist in some form in the model’s weights.
However, while developers and creators might disagree over the extent of copying involved in AI training, unarguably there are at least some acts of copying which will be a prima facie infringement of copyright. So in the US, the debate quickly comes down to the scope of the fair use defence.
Is AI training a fair use of creator’s content?
‘Fair use’ is a very complex, US-specific concept. Many other countries, including Australia, also have free use exceptions which allow fair use of copyright works without the creator’s consent, but in line with the Berne Convention, these tend to be closed short lists of particular uses specified in the copyright law, for example criticism, review or research.
The Report discusses how the four factors that must be considered in determining if the general US fair use exemption could apply to AI training.
Factor one: Transformativeness
Copyright is designed to protect the economic interests of the creator. If the allegedly infringing work has a further purpose or different character (that is, is transformative of the original copyright work), then it is less likely to substitute for the original work in the marketplace and therefore is less likely to undermine the economic interests of the creator of the original work.
This concept of transformativeness is a matter of degree and not every transformative use will ultimately be considered a fair use. Also relevant is whether the new use serves commercial or non-profit purposes and whether the defendant had lawful access to the work.
The US Supreme Court held in 2023 that physically transforming a work into another medium would not make the use transformative (for example Andy Warhol making a screen print based on a photo portrait of Prince) if the purpose was substantially similar to the original work (commercialising the work for profit (Andy Warhol Foundation v Goldsmith)). On the other hand, a US Court of Appeals found in 2025 that Google scanning books verbatim to create a full-text searchable database to provide an index of the books’ contents did serve a “highly transformative purpose” (Authors Guild v Google).
The US Copyright Office rejected two commonly made arguments that AI training is inherently transformative:
Developers argue that while the purpose of the original copyrighted works is for expressive purposes, copying in AI training is for the very different purpose of deconstructing existing works to model mathematically how language works and then using that statistical analysis to generate new digital artefacts. However, the Copyright Office considered that the very purpose of training is for the model to learn how to mimic human expression:
“Language models are trained on examples that are hundreds of thousands of tokens in length, absorbing not just the meaning and parts of speech of words, but how they are selected and arranged at the sentence, paragraph and document level – the essence of linguistic expression. Image models are trained on curated datasets of aesthetic images because those images lead to aesthetic outputs.”
Developers also argue that AI training is like humans learning in a library. The US Copyright Office observed that humans do not swallow whole or literally the copyrighted works they study:
“Humans retain only imperfect impressions of the works they have experienced, filtered through their own unique personalities, histories, memories and worldviews. Generative AI training involves the creation of perfect copies with the ability to analyse works nearly instantaneously.”
The US Copyright Office noted that it is these human limitations that underlie the structure of the exclusive rights comprised in copyright.
Instead, the US Copyright Office considered that transformative use is a question of fact and degree – which is likely to differ between models and at what point the training occurs in the AI supply chain. The development of the generative AI model will often be transformative because the training process converts a massive collection of training examples into a statistical model that can generate a wide range of outputs across a diverse array of new situations: for example a model trained on data including the Humphrey Bogart/Lauren Bacall movie the Big Sleep (filmed over 75 years ago with a convoluted, mystifying plot) can help you write a succinct, to-the-point email to your boss.
On the other hand, the US Copyright Office said that there are AI models whose purpose is much closer to the purpose of the training materials. For example, a foundation image model might be further trained on images from a popular animated series and deployed to generate images of characters from that series. These models would be so close to the training input that they would be derivative works and covered by the copyright of the original works. It follows that these could not amount to transformative fair use of the original works.
Many AI models would fall between these two poles, “sharing the purpose and character of the underlying copyrighted works without producing substantially similar content”. These models at best would be moderately transformative. The US Copyright Office does not venture a view about whether that would be enough transformation for fair use, but notes that restrictions on outputs (for example guardrails such as rejecting requests for excerpts of copyright works) may assist.
Factor two: Nature of the copyright works
The US courts have considered that the use of more creative or expressive works (such as novels, movies, art or music) – which are at the heart of copyright – is less likely to be fair use than use of factual or functional works (such as computer code). Whether or not the work has previously been published is another consideration under this factor, which is directed at the core of protection intended by copyright.
Developers are in a race to make their AI models ever more human-like and the best place to learn to mimic human emotions is in works of human creativity. The Copyright Office concludes that this second factor will usually tell against developers successfully making out fair use but won’t be decisive of itself.
Factor three: How much is copied
This is both a quantitative and qualitative assessment. Downloading works, curating them into a training dataset and training on that dataset generally involve using all or substantially all of those works. However, the US courts have also held that mass copying of entire works is justified when it enables transformative uses.
In Google Books, Google’s index only made sense if it indexed the entire content. While the justification was not as strong, the US Copyright Office thought a case could be made that fair use allowed developers to copy whole works for AI training, at least where there are guardrails in place to prevent the generation of infringing content in user prompts:
Given the scale of the data required to achieve the performance of large language models, “the use of entire works appears to be practically necessary for some forms of training”.
Some US courts and commentators will discount the making of complete literal copies as an intermediate step where the ultimate user can only access snippets of the original work. The Copyright Office noted that, even if memorisation is more commonplace than the developers say, “[m]ost outputs from generative AI systems do not contain any protected expression from their training data and models can be deployed in ways that entirely obscure outputs from users or result in non-expressive outputs”.
Factor four: Impact on the market for the original work
This factor considers not only harm to the market for, and value of, the original work, but also harm to the market for derivative works. In one of its most unambiguous findings, the Copyright Office considered that the copying involved in AI training “threatens significant potential harm to the market for or value of the original copyrighted works”:
Loss of sales: For example creators could lose out from the use of pirated collections of copyright works to build a training library; from the emerging market for creation of works to train AI; and where training enables a model to output verbatim or substantially similar copies of the works trained on, from substitution of sales of the human-created work by the AI-created facsimile.
Market dilution: Developers argued that while it is possible for generative AI to create works of the same type that compete in the overall market with the originals, substitution should be more narrowly limited to the actual original copyrighted work, otherwise innovation would be stifled. However, the US Copyright Office thought that, while still unchartered territory, market harm could result from AI models generating material stylistically similar to copyrighted works in their training data:
“The speed and scale at which AI systems generate content pose a serious risk of diluting markets for works of the same kind as in their training data. That means more competition for sales of an author’s works and more difficulty for audiences in finding them. If thousands of AI-generated romance novels are put on the market, fewer of the human-authored romance novels that the AI was trained on are likely to be sold.”
Loss of licensing opportunities: The availability of licensing options is likely to weigh against fair use under this fourth factor. The Copyright Office observed that emerging deals between creators and developers to licence content for training demonstrates the potential new opportunities AI can provide creators to commercialise their work.
Conclusion
On US case law, the first factor (transformative use) and the fourth factor (market impact) are the most important. On the Copyright Office’s analysis, the first factor leans in favour of finding that AI training is fair use while the fourth factor leans in favour of finding it is not.
While the US Copyright Office says the final balancing act is up to the courts and is likely to vary on a case-by-case basis, reading between the lines, the Office tries to find a way forward for developers and creators.
First, developers and creators need to recognise that “there are strong claims to public benefits on both sides, many applications of generative AI promise great benefits for the public, as does the production of expressive works”.
Second, while transformative use may give developers latitude to use copyrighted works for training, developers could be better at developing and using training methods and guardrails which mitigate their AI models producing outputs which are substantially similar to the copyrighted works used in training.
Third, having found that some uses of copyright works to train AI models require licensing, the US Copyright Office considers options for licensing solutions in the final section of its report. It finds that a voluntary licensing market is developing and is likely to be influenced by the results of pending cases.
However, the feasibility of voluntary licensing varies widely across the AI market, so if creators want to earn a return from use of their works in AI training, they need to recognise the lack of licensing infrastructure which makes the licensing of content at the scale challenging for developers. For example, content providers could form collecting organisations to negotiate content class-wide deals with the major developers.
The US Copyright Office warns creators that if the barriers to licensing prove insurmountable for developers’ uses of some types of works, there will be no functioning market to be adversely impacted by copying for AI training and fair use will need to be the mechanism by which AI models get (free) access to the content required for training.
Other jurisdictions such as Australia, have in place existing statutory licensing and collecting society mechanisms which have been used to address mass copying with earlier generations of technology, such as photocopying.
Following the dismissal of the head of the US Copyright Office, it is unclear whether this nuanced attempt to find a way forward for both developers and copyright owners will be dead on arrival.
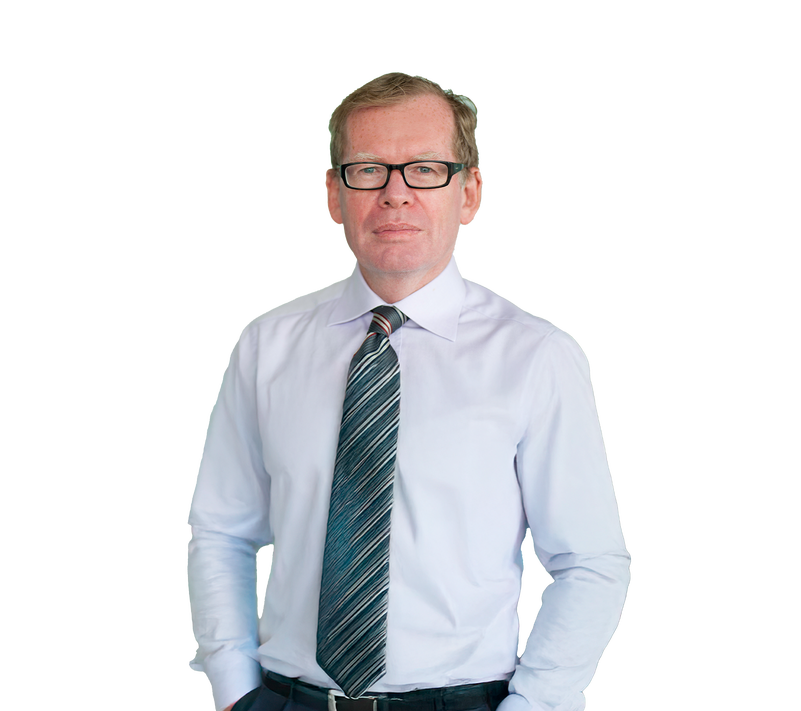
Peter Waters
Consultant