As AI requires vast lakes of data for training, there is an assumption that leadership in AI R&D requires huge and escalating computing capacity.
The big economies are involved in a computing ‘arms race’. The US is spending $500 million building an exascale computer which will double the capacity of its biggest existing computer (exascale =1,000,000,000,000,000,000 operations per second). The European Union has commissioned the European High Performance Computing Joint Undertaking (EuroHPC JU) with an exascale computer in Germany. China alone accounts for 12% of global super computer capacity.
The US National Security Commission concluded that—due to the computing and data needs of cutting-edge AI systems—“the development of AI in the United States is concentrated in fewer organizations in fewer geographic regions pursuing fewer research pathways”.
What hope then for smaller economies which cannot afford investment in massive computing capacity?
What hope then for smaller economies which cannot afford investment in massive computing capacity? A recent Australian Government paper commented that:
“Australia has capability in AI-related areas like computer vision and robotics, and the social and governance aspects of AI, but its core fundamental capacity in LLMs and related areas is relatively weak. While the Australian Government announced investments of $100 million in AI-related initiatives (e.g including a national AI centre), creating generative AI technologies has especially high barriers to access, due to its considerable compute and data requirements.”
But a recent study by Georgetown University’s Center for Security and Emerging Technology (CSET) suggests a more nuanced picture for success in AI R&D.
CSET undertook its study because there is no comprehensive data available on compute use among AI researchers. CSET surveyed AI researchers identified through leading AI journals and LinkedIn entries. The responses came from a wide range of public and private sector researchers: of the 410 responses, 67 percent reported working in academia, and 29 percent in industry. Among respondents who reported working in industry, 70 per cent reported working for a company with more than 500 employees, while 30 percent reported working for a company with 500 or fewer employees.
Compute is not the primary constraint for many AI researchers
Respondents were asked to identify their projects over the last 5 years that made the biggest contribution to research in their field and the projects that consumed the most computer resources. Over two thirds reported that the two projects were the same. But when asked what made the project successful, 90 percent rated “specialized knowledge, talent, or skills,” 52 percent rated “large amounts of compute” and 51% ranked access to “unique data”.
To double check on the relative significance of factors, researchers were asked to imagine if the budget for their current or most recent AI project doubled, what would their first priority be to spend the extra money on? Roughly half (52 percent) said that they would spend more on hiring more talent, while only about a fifth of researchers would make purchasing more or higher-quality compute their first priority, and a similar share would prioritise collecting or cleaning data..
As a third cross check, respondents were asked the reasons they had to refuse, abandon or revise AI projects over the last 2 years. Researchers report rejecting and abandoning projects due to a lack of data or researcher availability more often than due to a lack of compute resources. But when it came to the reason for revising projects, over three quarters of researchers identified problems with access to sufficient computing power.
Compute less important going forward to AI progress
Respondents were asked, looking back over the last 10 years, which of five factors - data, compute, algorithms, number of researchers, and level of support for AI projects – was the most important contributor to AI progress. They were then asked the same question going forward over the next 10 years.
Looking backwards, 59 percent of respondents thought AI progress was primarily driven by computing power. But looking forward, only 40 percent considered computing power would be the main driver, with the focus shifting to algorithms.
Although in looking at these results, CSET cautions against the risk of a degree of self-interest and self-importance amongst researchers:
“Predictions that the importance of algorithms will rise while the importance of compute falls could be a reflection of the researchers’ own interests rather than a developing trend. Researchers tend to view progress that comes from the brute force approach of simply using more compute as less interesting and valuable than new approaches that require their knowledge and creativity. That more compute often outperforms more ingenuity has been a “bitter lesson” that has perhaps still not been entirely internalized by the research community.”
Public vs Private sector computing
One of the feared outcomes of the need for massive computing capacity to support AI is that Big Tech will be better positioned than the public sector – even than some nations – to build that computing capacity.
However, the CSET study shows a less stark picture:
- while AI academic report that spend on computing in their projects is much lower than commercial researchers report, the hours of computing capacity per project is very similar between the public and private sectors;
- when AI academics were asked if they had thought about moving to the private sector and why, only 35% ranked access to better computing resources as significant deciding factor, a long behind the 70% who identified better remuneration.
- AI academics do not express any greater concern than commercial AI researchers about the level of access they will have to computing resources they need for the future. Rather, current heavy users of computing resources – whether academic or commercial – are concerned they will have to share more access in the future as the ‘popularity’ of AI research expands.
Start-ups
If AI R&D depended on access to large scale computing, start-ups might be expected to be concerned about the asymmetrical advantages which Big Tech have. However, access to talent was still the top priority for start-up respondents. Interestingly, start-ups also attributed project success much more to access to data than computing power.
Where Government’s should be investing their AI research funds
The CSET report notes that the US Government’s decisions to invest massive sums in computing capacity is driven by the well-meaning assumption that provisioning large amounts of computing resources to a wider number of researchers could “democratize” AI research by allowing poorly resourced researchers to compete with better-resourced ones. However, the CRET cautions that this policy could prove counterproductive:
“Such a strategy could actually backfire, resulting in differences in compute usage becoming even further stratified. Across a wide number of indicators, we found that the researchers who were most eager for greater amounts of compute were the same ones who already used more compute than their peers. … this in turn suggests that if more compute were made available across the board to researchers, it might primarily benefit high compute users, without becoming a major resource for researchers currently using less compute.”
Perhaps more cynically, politicians love ‘ribbon cutting’ events, which makes investing Government funds in shiny computer hardware more attractive than investing in less tangible projects such as growing talent or in access to better data sets to more accurately and ethically train AI.
Conclusion
The CSET study suggests that there is still room for mid-sized and smaller economies to make headway in AI innovation with a more nuanced, mixed set of policy initiatives. The study concludes that:
“compute cannot be viewed as an all-purpose lever for promoting AI progress.. talent is more important than compute for fostering AI research, so policymakers should evaluate how compute-focused interventions can be coupled with policies to foster AI talent in order to effectively promote AI research progress.”
The punchline is that “the main resource [in AI R&D] is human”
Read more: The Main Resource is the Human: A Survey of AI Researchers on the Importance of Compute
KNOWLEDGE ARTICLES YOU MAY BE INTERESTED IN:
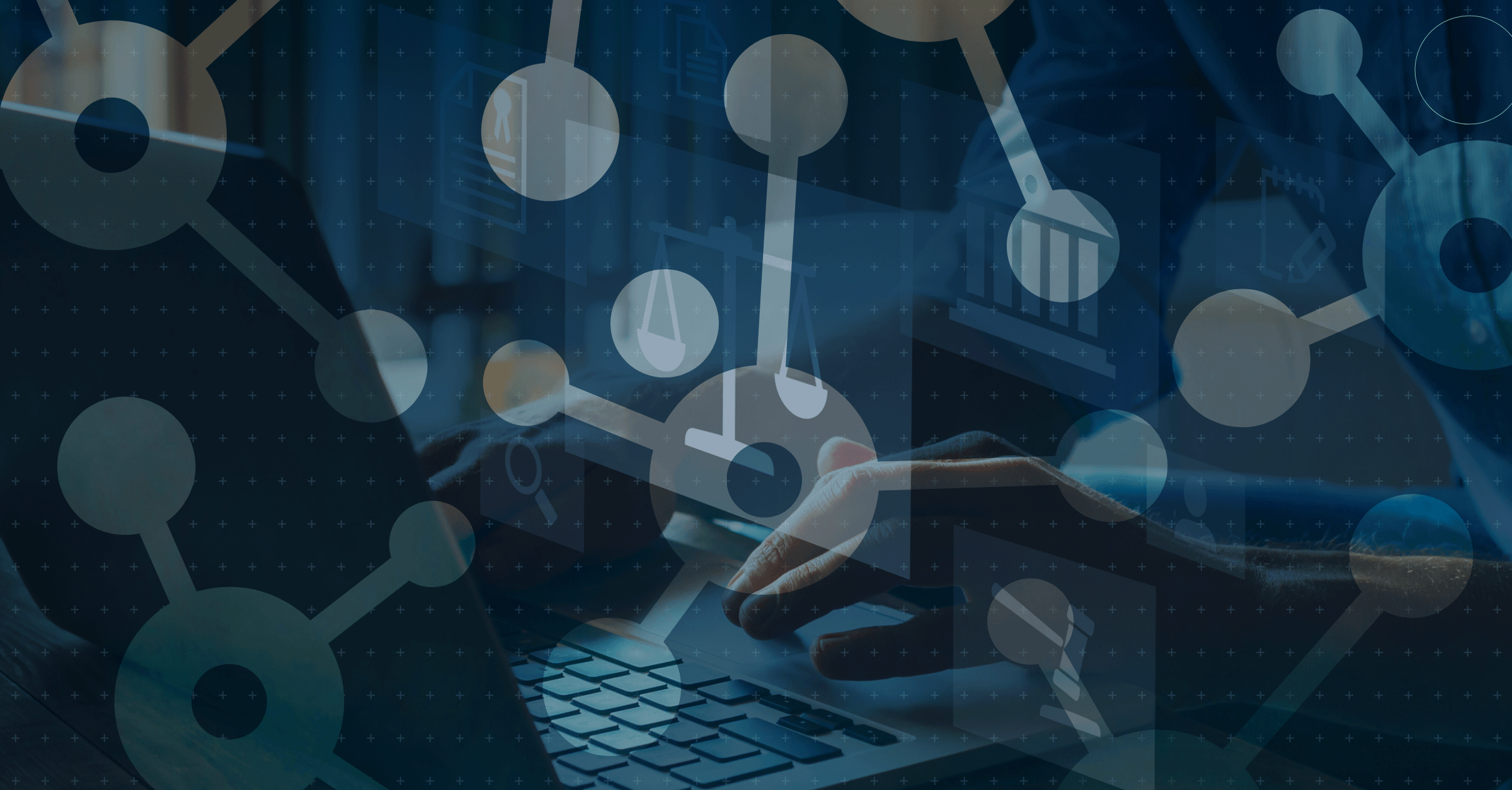
Visit Smart Counsel