AI has the potential to transform legal services: one CEO of an AI company has offered US$1 million to a litigator with an upcoming case in front of the United States Supreme Court prepared to wear AirPods and let its robot lawyer argue the case by repeating exactly what it says.
However, a recent study by Stanford University researchers has found that hallucinations about the law are “alarmingly prevalent” in AI responses, occurring 69% of the time with ChatGPT 3.5 and 88% with Llama 2. While hallucination is a well-known risk of large language models (LLMs), they are particularly consequential when dealing with legal issues because “adherence to the source text is paramount, [and] unfaithful or imprecise interpretations of law can lead to nonsensical—or worse, harmful and inaccurate—legal advice or decisions.
How does AI legally hallucinate?
The Stanford researchers catalogued three ways in which LLMs can hallucinate about legal issues:
an LLM might hallucinate by producing a response that is either unfaithful to or in conflict with the input prompt (called a closed-domain hallucination). This is a major concern in tasks requiring a high degree of accuracy between the response and a long-form input, such as inaccuracies in summarizing a specific judicial opinion, synthesizing client intake information, drafting legal documents, or extracting key points from an opposing counsel’s brief.
an LLM might hallucinate by producing a response that either contradicts or does not directly derive from its training data (called open-domain hallucination). The way AI should work is that the output of an LLM should be logically derivable from the content of its training data (whether that data itself is right or wrong). For example, an LLM trained solely on common law texts provides an answer on how an issue would be addressed in a civil law jurisdiction. As the Stanford study observes, “this kind of hallucination poses a special challenge to those aiming to fine-tune the kind of general purpose foundation modelswith proprietary, in-house work product,[f]or example, firms might have a catalogue of internal research memos, style guides, and so forth, that they want to ensure is reflected in their bespoke LLM’s output”
an LLM can hallucinate by producing a response that lacks fidelity to the facts of the world, irrespective of how the LLM is trained or prompted, which is another type of open-domain hallucination. For example, the reported cases of lawyers filing briefs with made-up cases in them. The Stanford study considered that “this is perhaps the most alarming type of hallucination, as it can undermine the accuracy required in any legal context where a correct statement of the law is necessary.”
The Stanford study makes the good point that not every AI hallucination is a bad thing - one person’s hallucination is another person’s creative argument or novel insight. Strict interpretation constitutionalists might say that the new Chief Justice of the Australian High Court, Justice Stephen Gageler, was “hallucinating ” when as a junior counsel he saw an implied right freedom of political speech within the Australian Constitution, which had hitherto been read as largely devoid of human rights. As the Stanford study remarks:
..insofar as creativity is valued, certain legal tasks—such as persuasive argumentation—might actually benefit from some lack of strict fidelity to the training corpus; after all, a model that simply parrots exactly the text that it has been trained on could itself be undesirable. Defining the contours of an unwanted hallucination in this context requires value judgements about the balance between fidelity and spontaneity.
Designing the legal exams which the AI sat
The researchers randomly picked a ‘control’ group of 5000 decided cases across the three levels of the US federal judiciary (Supreme Court, circuit courts of appeal and district courts) about which to ask questions of three LLMs, ChatGPT 35, PaLM 2 and Llama 2. The questions fell into three categories of ascending difficulty:
low complexity tasks: these basic questions were focused on whether the AI can distinguish real cases from non-existent cases. Prompts used in the test included: given the name and citation of a case, ascertain whether the case actually exists, which court decided it and who wrote the majority judgment? These responses required no knowledge of the legal reasoning in the cases and should be fairly simple and factual: but AI has been reported as having Justice Ruth Bader Ginsburg dissenting in the US Supreme Court’s marriage equality decision in Obergefell.
moderate complexity tasks: to answer the queries in this category, an LLM had to know something about a decided case’s substantive content. Prompts used in the test included: given a case name and its citation, state whether the court affirmed or reversed the lower court, what precedents did the court rely on, and confirm whether and in what year it was overruled?
high complexity tasks: these questions both presuppose legal reasoning skills (unlike the low complexity tasks) and answers that are not readily available in existing legal databases like WestLaw or Lexis (unlike the moderate complexity tasks). These tasks all required an LLM to synthesize core legal information out of unstructured legal prose—information that is frequently the topic of deeper legal research. Prompts used in the tests included: given a case name and its citation, state its factual background and what was the legal issue; and compared with another case and its citation, state whether they agree or disagree with each other?
How AI scored on these exams
The study found that the tested LLMs hallucinate at high levels across the simple, moderate and complex tasks, as depicted below. Other studies have found that that GPT 3.5 hallucinated about 14.3% of the time on general Q&A queries. But on this study’s tests, GPT 3.5 and the other LLMs performed on legal Q&A much worse than this baseline rate — hallucinating at least 57% of the time on simple tasks climbing to at least 75% of the time in more complex tasks.
On many binary questions - such as whether two cases agree or disagree with each other - the LLMs performed little better than random guessing. As the study notes, “[t]his suggests that LLMs are not yet able to perform the kind of legal reasoning that attorneys perform when they assess the precedential relationship between cases— a core purpose of legal research.”
Other findings of the study were:
hallucinations are lowest in response to prompts about decided cases at the higher levels of the judiciary, such as the US Supreme Court.
hallucination rates varied by geographic location of the courts in the US, with the figure below showing lower hallucination rates in lighter colours and higher rates in darker colours. As the study notes, this conforms with lore amongst US lawyers that the Ninth Circuit (California) and the Second Circuit (New York) have more influence in the US legal system, although the tested LLMs appear to rate the District of Columbia Circuit (which handles important administrative law cases) as less consequential than human lawyers!
hallucinations are most common among the Supreme Court’s oldest and newest cases, and least common among the ‘in-between’ cases from the post-war Warren Court (1953-1969). The study concludes that “[t]his result suggests another important limitation on LLMs’ legal knowledge that users should be aware of: LLMs’ peak performance may lag several years behind the current state of the doctrine, and LLMs may fail to internalize case law that is very old but still applicable and relevant law.”
the prominence of an individual case is negatively correlated with hallucination - which means that the more prominent a case is (i.e. how often it is cited in other decisions) the more likely the AI will not hallucinate about it.
across the board, the tested LLMs tend to overstate the true prevalence of individual judges at a higher magnitude than they understate them. This might be expected of more famous or oft-cited judges because they appear more frequently in the training data, but some of the LLMs had ‘quirky' favourites amongst lesser known or dissenting judges.
Is AI too sycophantic and over confident?
Looking beyond the challenge of hallucination, the Stanford study also considered other challenges in using AI as a legal research tool. Junior lawyers faced with a puzzling legal research task vaguely framed by partner as they hurry by your desk will recognise how the Stanford study described the process of legal research:
When a researcher is learning about a topic, they are not only unsure about the answer, they are also often unsure about the question they are asking as well. Worse, they might not even be aware of any defects in their query; research by its nature ventures into the realm of unknown unknowns. This is especially true for unsophisticated pro se litigants (self-litigants), or those without much legal training to begin with. Relying on an LLM for legal research, they might inadvertently submit a question premised on non-factual legal information or folk wisdom about the law.
To test this risk, the researchers put prompts to the tested LLMs which deliberately incorporated false assumptions: they asked the LLMs to (1) provide information about a judge’s dissenting opinion in an appellate case in which they did not in fact dissent and (2) furnish the year that a US Supreme Court case that has never been overruled was overruled.
As the following graph shows, the LLMs did not detect the false premise in the prompt, but in fact ‘ran with it’ and provided a hallucinated response as if the prompt was factually correct. Llama 2 appears to perform much better, but this was often because it wrongly responded that the case or the judge did not exist (when in fact they did exist but the prompt changed what happened in the case).
The study characterised this as “a kind of model sycophancy.. [being] the tendency of an LLM to agree with a user’s preferences or beliefs, even when the LLM would reject the belief as wrong without the user’s prompting.”
The Stanford researchers also wanted to assess the ability of AI to “know what it knows” or put another way to “know when it does not know”, because:
[i]deally, a well-calibrated model would be confident in its factual responses, and not confident in its hallucinated onesresearchers would be able to adjust their expectations accordingly and could theoretically learn to trust the LLM when it is confident, and learn to be more skeptical when it is not. Even more importantly, if an LLM knew when it was likely to be hallucinating, the hallucination problem could be in principle solvable through some form of reinforcement learning from human feedback (RLHF) or fine-tuning, with unconfident answers simply being suppressed.
The study’s methodology was to extract a confidence score for each LLM answer that it obtained and compare it to the empirical hallucination rate that was observed.
The study concluded that:
..our LLMs systematically overestimate their confidence relative to their actual rate of hallucinationNot only may [users] receive a hallucinated response, but they may receive one that the LLM is overconfident in and liable to repeat again.
So what does this mean for use of AI in legal practice?
AI developers have been working on reducing hallucinations rates - ChatGPT 4 is said to have a lower hallucination rate than GPT-3.5 (2.3% vs. 27.3%).
But as hallucination is likely to continue to be a risk, and as specialist areas like the law depend on accuracy, users like law firms and inhouse legal departments will need to adopt mitigations. These could include:
more systemically apply that ‘stock-in-trade’ of lawyers, scepticism, to the use of AI in legal practice. This applies to users’ willingness to accept the responses from AI but the Stanford researchers also suggest that in its design, legal AI itself could have an embedded degree of scepticism in processing user prompts: more AI responses along the lines of “do you really mean to ask the question in that way?”
framing prompts in a structured way which is more exacting for the AI. There is an emerging ‘science’ of ‘prompt engineering’. Prompts can be framed to require the AI to explain its ‘chain of reasoning’, to provide references and to give and explain its level of confidence in its answer. Cleverer prompts also can provide the AI with some logic guidance or ‘clues’ to the answer, which itself will reduce the risk of hallucination.
the law firm or inhouse legal department should consider ‘injecting’ a general LLM with higher quality current legal data, such as through fine-tuning or a plug-in. This could, for example, be a data source of legal text books. This, of course, may require copyright licensing.
reinforcing to legal researchers that they need to stick by that old adage of legal research - always go back to the primary source!
The Stanford study also has two warnings about the broader transformative impact which use of AI may have on the law itself.
First, there is the risk of producing a ‘kind of legal monoculture’. As the study findings show, by reducing legal knowledge to mathematically based parameters that predict probabilities based on past cases, AI can reinforce the predominance of particular courts, judges or cases. But more fundamentally, AI could ‘dictate’ a more rigid conformity in future legal cases to past decisions. As Justice Kirby of the Australian High Court often said, the ‘genius’ of the common law is as much about its ability to respond creatively as it is about sticking with precedent:
A system based upon the common law, of its nature, requires a creative judiciary. If the judges of the common law did not so act where plain justice demands action, the law would fail to adapt and change to modern society.
Second, AI may fail to live up to the hope on the part of some that it will ‘democratise’ access to law. As the Stanford study comments:
Although data-rich and moneyed players certainly stand at an advantage when it comes to building hallucination-free legal LLMs for their own private use, it is not clear that even infinite resources can entirely solve the hallucination problem we diagnose. We therefore echo concerns that the proliferation of LLMs may ultimately exacerbate, rather than eradicate, existing inequalities in access to legal services.
Read more: Large Legal Fictions: Profiling Legal Hallucinations in Large Language Models
KNOWLEDGE ARTICLES YOU MAY BE INTERESTED IN:
Australia’s Cyber Security Strategy for 2023-2030 is here
Can new AI help to level up the scales of justice?
Federal Court rejects Optus's privilege claim over Deloitte report
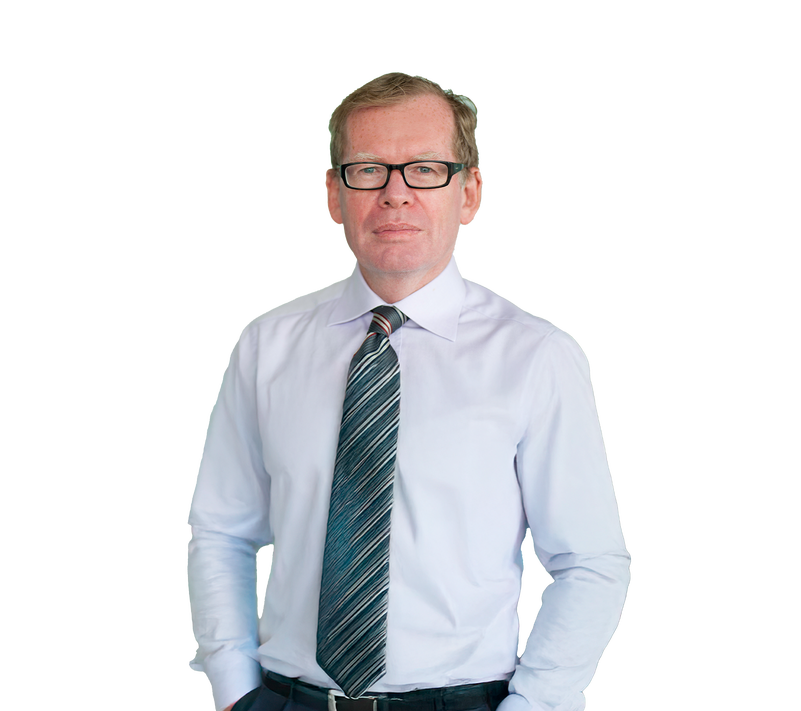
Peter Waters
Consultant