Spurred by President Biden’s AI Executive Order, US federal agencies are churning out proposals, guidelines and rules on AI governance. Last week we reviewed the OMB’s memo on AI governance in the bureaucracy . This week it’s the National Telecommunications and Information Administration (NTIA) report on AI accountability, disclosure and auditing requirements - including the proposed AI version of nutrition labels on the side of your cereal box.
Viewing accountability through the other end of the telescope
The NTIA makes the obvious point that “AI accountability policies and mechanisms can play a key part in getting the best out of this technology.” But the NTIA also makes the less recognised point that the accountability of AI developers depends on downstream developers and users being able to test AI developers:
To be clear, trust and assurance are not products that AI actors generate. Rather, trustworthiness involves a dynamic between parties; it is in part a function of how well those who use or are affected by AI systems can interrogate those systems and make determinations about them, either themselves or through proxies.
The NTIA argues that, viewed this way around, AI accountability mechanisms crucially depend on the ready availability of information about:
the creation, collection, and distribution of information about AI systems and system outputs; and
AI system evaluation.
In the absence of this information - in a standardised form to allow comparison between different models - there are information asymmetries and gaps up and down the AI supply chain:
downstream deployers lack the information they need to appropriately in different contexts (e.g. medical vs CRM use cases);
upstream developers may lack information about deployment contexts and therefore make inaccurate claims about their products. Their harm mitigation efforts are also hampered because they may be unaware of serious adverse developments (the OECD is developing a global adverse incidents database);
individual users may not even be aware that an AI system is at work, much less how it works.
The NTIA argues that to get flowing the information required or AI accountability requires two types of measures:
the disclosures that AI developers are required to push out to users; and
the information stakeholders can pull from AI systems about how the system works.
What should developers disclose?
The NTIA identified a ‘family’ of push information artifacts that developers could make available:
Datasheets (also referred to as data cards, dataset sheets, data statements) to provide salient information about the data on which the AI model was trained, including the “motivation, composition, collection process, [and] recommended uses” of the dataset.
Model cards to provide information about the performance and context of the AI model, including “[o]n-label (intended) and off-label (not intended, but predictable) use cases.”
System cards to show how an entire AI system, often composed of a series of models working together, performs step-by-step a task, for example to compute a ranking or make a prediction.
These can be synthesised into a single ‘nutrition’ label, like as follows (originally proposed by Twilio):
Sitting behind these push artifacts usually will be technical papers and the NTIA says these should be available on a pull basis, subject to potential audience restrictions for IP and security issues. However, the NTIA noted that there currently is a high degree of variability in the availability, scope and accessibility (i.e. extent of opaque technical jargon) in these papers:
These differences frustrate meaningful comparison of different models or systems. The differences also make it difficult to compare the adequacy of the artifacts themselves and distinguish obfuscation from unknowns. For example, one might wonder whether a disclosure’s emphasis on system architecture at the expense of training data, or fine-tuning at the expense of testing and validation, is due to executive decisions or to system characteristics. Like dense privacy disclosures, idiosyncratic technical artifacts put a heavy burden on consumers and users. The lack of standardization may be hindering the realization of these artifacts’ potential effectiveness both to inform stakeholders and to encourage reflection by AI actors.
Improving AI model evaluation
The NTIA’s proposals on developer pre-release evaluation of AI models is on more familiar ground, although the NTIA has some interesting insights of its own.
First, it is important to distinguish between two very different purposes of evaluation, because different tools (and different levels of regulation) may be required to ensure evaluations are effective in meeting each purpose:
claim validation: Is the AI system performing as claimed with the stated limitations? This is the more straightforward purpose to evaluate because “it is more amenable to binary findings, and there are often clear enforcement mechanisms and remedies to combat false claims in the commercial context under [competition and consumer protection laws].
societal values: this evaluation examines the AI system according to a set of social criteria independent of an AI actor’s claims, such as discrimination. This is a more difficult exercise: identifying what values are to be tested, which itself can be a values-laden exercise; deciding who should be involved in the evaluation, such as potentially harmed or marginalised groups; and evaluating outputs against the selected criteria, which again can require qualitative judgments.
Second, the NTIA stressed “the need for accelerated international standards work.” But at the same time, the NTIA acknowledged the challenges to standards setting of “the relative immaturity of the AI standards ecosystem, its relative non-normativity, and the dominance of industry in relation to other stakeholders.” As a result:
Traditional, formal standards-setting processes may not yield standards for AI assurance practices sufficiently rapidly, transparently, inclusively, and comprehensively on their own, and may lag behind technical developments.
The NTIA had two suggestions:
standards may need to start as non-prescriptive (i.e. more ‘should’ than ‘shall’ language) as standards setting bodies, in effect, learn on the job as AI continues to develop. The NTIA said it was “cognizant of the critique that non-prescriptive stances have sometimes impeded efforts to ensure that standards respect human rights”, but that this could be mitigated by ensuring involvement of a broad range of stakeholders along the journey “as non-prescriptive guidance comes to develop normative content and/or binding force.”
governments should take a more proactive role in standards setting, such as by funding the research required to underpin standards and providing guidance on how societal values should be taken into account.
Third, the NTIA supported the development of a common certification scheme, along the line of energy ratings on electrical appliances. However, the NTIA leaned against a mandatory pre-release licensing or certification scheme for several reasons:
given the prospect of AI becoming embedded ubiquitously in products and processes, it would be impractical to mandate certification for all AI systems.
the costs and complexity of certification could be a barrier to entry of new AI developers and entrench existing incumbents’ market power.
Fourth, certification - voluntary or mandatory - won’t be credible without evaluation by third parties who are independent of the developer and who apply common audit standards. But the NTIA thought that the baseline requirement, and the starting point in any evaluation, should still be self-evaluation by the developer. Self evaluation “benefits from its access to relevant material” and “will tend to improve management of AI risks by measuring practices against ‘established protocols designed to support an AI system’s trustworthiness’”.
The NTIA basically supports a hybrid model in which regulation or standards required developers to lift their game on self evaluation, such as by requiring ‘red teaming’ and then in the case of riskier models, independent evaluation then sat over the self evaluation.
Fifth, the NTIA says that “accountability [should be conceived] as a chain of inputs linked to consequences”:
Consequences for responsible parties, building on information sharing and independent evaluations, will require the application and/or development of levers - such as regulation, market pressures, and/or legal liability - to hold AI entities accountable for imposing unacceptable risks or making unfounded claims.
The main consequence is legal liability. While the NTIA did not offer an opinion on how liability should be allocated along the AI supply chain, it encouraged regulators, such as competition and consumer protection authorities, to be more ambitious in their efforts to test and clarify the law.
Interestingly, the NTIA canvassed the use of new AI-specific safe harbors to incentivise developer behaviour, such as an immunity for sharing information with other developers about adverse events.
Read more: AI Accountability Policy Report
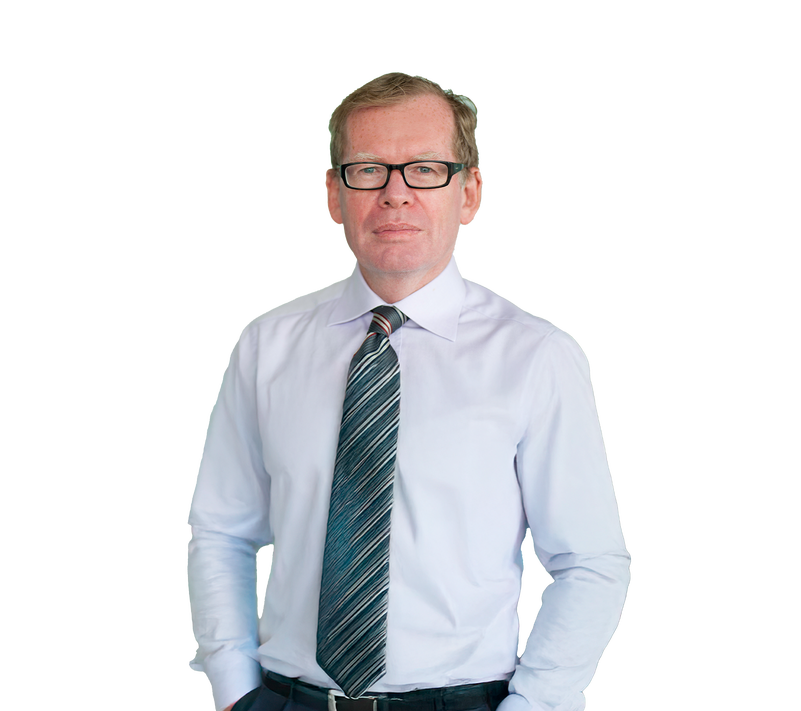
Peter Waters
Consultant