AI and cybersecurity are individually two of the most newsworthy topics this year, given the rapid popularisation of generative AI on one hand, and the landscape of increased cyber-attacks and data breaches on the other. Individually they are ubiquitous, but it is rare to see them grouped together.
A joint report from Georgetown University’s Centre for Security and Emerging Technology and the Alan Turing Institute does just that, evaluating an increased interest in the application of AI to enhance cyber defence.
What is autonomous cyber capability?
Autonomous cyber defence uses AI to protect network and systems by being able to detect malicious activity and react at the speed of digital attacks.
Autonomous cyber defence may include defensive countermeasures built around identifying and detecting breaches, but it can also go beyond threat detection to implement steps like system hardening and using decoys to respond to an attack.
What makes the cyber defence autonomous is the AI’s capacity to make high-stakes decisions without requiring explicit prior human approval or authorisation. As soon as human approval is required (a ‘human in the loop’), a cyber defence loses its speed advantage over cyber-attacks. The US Naval Research Laboratory describes the widening mismatch between cyber-attacks and cyber defence as follows:
"Adversaries are able to launch cyberattacks that are dynamic, fast-paced, and high-volume, while cyber responses are human-speed. In current cyber defense systems, most system adaptation and recovery processes are ad-hoc, manual, and slow. Keeping pace with existing and emerging cybersecurity threats is a challenging task, especially without the benefit of relying on the human expertise of system administrators and cyber warriors".
But as we consider below, there could be legal risks arising from removing human oversight.
Australia has its own simulated learning environment (called a ‘gym’) for cybersecurity called CybORG, and globally it has been used in a series of cyber defence competitions and challenges commencing in 2021. The joint report comparison of global gyms finds Australia’s CybORG worthy of “special attention” as it is open source, its design is defence-focused (which is important, as we explain below) and it allows for relatively complex simulations.
The technical challenges of autonomous cyber defence
The joint report frankly acknowledges that the current AI technology is not sufficiently mature and further scaling, testing and training is required for operational autonomous cyber defence agents. The joint report recommends training these agents through reinforcement learning (RL), a well-known machine learning paradigm through which reward drives desired behaviour (used, for example, by OpenAI in building chatGPT).
But the joint report acknowledges there are significant challenges.
The primary challenge is “selecting tasks and building training environments that are complex enough to be useful, while small enough in terms of the number of actions and observations to be manageable”. The joint report describes the nub of this challenge as follows:
“Every configurable setting on every computer, router, and device is a potential action. Moreover, every bit of data flowing in a network or sitting on a computer is potentially important to observe. For example, ten computers that each have ten pieces of software that each have ten possible security settings to configure leads to one thousand possible actions…The number of actions and observations grows exponentially and quickly becomes unmanageable."
The joint report posits that building and training a single system is likely to be infeasible. It instead suggests an approach that utilises a series of separate interacting agents trained individually on a narrower set of tasks:
“For example, one agent may only think of computers as black boxes that can be infected or clean, and it may only be able to perform a few actions to isolate or remediate them. Another agent may be working on those computers, observing all the processes that are running and user behaviors. It could decide whether to kill some of those processes or lock out the users, and it could tell the first agent whether or not the computer is infected.”
Second, the ability to remember the nature and signature of previous attacks is critical to effective cyber defence:
“From a defensive perspective, discovering malware signatures or attacker tactics is only helpful if they can be remembered. A capable autonomous cyber defense agent cannot be an amnesiac.”
However, cyber defence agents that are deployed to the networks they are defending must be small enough that they will not exhaust the computational resources of those networks and devices. As a result, state of the art cyber defence has comparatively limited capacity to store parameters.
The joint report proposes an approach in which information is stored outside of the neural network and accessed by the cyber defence agents when needed, which is the method adopted by the Canadian CyGil gym.
Third, under any RL approach, it is important to carefully and clearly define the goals, which will often require a prioritisation of goals which are in tension with each other. This can be a particular challenge in managing cyber-attacks because of the endless variety of potential attack scenarios and the challenge for the agent in assessing the degree of seriousness each time when deciding when and how to trade off limiting data loss and maximising up-time. Algorithms also can be very ‘literal’ in their reactions if the goals are too broadly defined: for example, if the agent’s goal is simply to “keep malware off all systems,” it may achieve this goal by precipitously turning all of the systems off.
The Frankestein factor
Many of the current cyber gyms are primarily intended for creating offensive agents, as they are required in order to test and build the capability of defensive agents. In fact, the joint report says that “[i]t is unclear at this stage if it is even feasible to build defensive agents without also building their offensive counterparts.”
Obviously, offensive agents could cause significant harm if they are leaked or stolen. Ominously, the joint report notes that it is presently unclear, in a contest between intelligent autonomous cyber attackers used to ‘beget’ autonomous cyber defenders and those defender counterparts, who would win.
As more complex offensive agents are developed, the joint report highlights the need for stringent protective measures to prevent them being stolen or disclosed without authorisation, and subsequently deployed or reverse engineered.
Unpacking the legal challenge
Building autonomous cyber defence agents poses a range of policy challenges in the creation stage, as well as for long-term use and management once the agents are fielded.
One major challenge is that the reinforcement learning agents used to train these tools requires “detailed data about how networks, computers, and devices are set up, managed, and attacked” to design realistic defences. The sharing of data is a major policy challenge as most of the data is held by private companies who regard the data as proprietary material (and presumably a security risk if disclosed), holding it in confidence and protecting its privacy. Moreover, in the national security domain, data on threats, incidents, responses and weaknesses is sensitive and controlled, with strict data protection restrictions surrounding its use.
The joint paper proposes that to advance this space, it is necessary to craft new regulations or establish new norms, but there are clearly challenges to be overcome in that sharing this data without adequate safeguards could create increased security risks even where the intention is to do precisely the opposite.
What happens when these agents fail to detect or prevent an attack? The possibilities for failure are wide-ranging, and include both overreacting to imagined threats and failing to identify or respond to attacks that they should be prepared for. Agents may also cause harm by operating without authorisation beyond the boundaries of the networks which they are defending.
For regulated entities, there are obvious risks in relying on autonomous cyber defence to discharge, or help discharge, regulatory obligations such as those imposed on certain financial institutions under prudential standards such as CPS 234, 231 and 230 issued by the Australian Prudential Regulatory Authority.
If customers procuring these defence agents secure robust contractual liability regimes, that may go some way towards protecting against more clear-cut risk allocation issues. However, the harms arising from cybersecurity failures are difficult to adequately mitigate through contractual means, given not only exposure to regulatory penalties and to customer claims but most materially, the potential for substantial reputational harm
Seizing the higher ground: can AI provide a tactical advantage against cyber-attacks?
As cyber-attacks escalate beyond the speed, frequency and scale that humans can address, cyber-security will increasingly become a ‘battle of the machines’.
The joint report notes that, while there have been plenty of lab tests and competitions, the authors are not aware of an autonomous cyber defence system yet being deployed in the real world. However, while there is no guarantee that autonomous cyber defence will succeed, the joint report argues that the autonomous cyber defence space appears to be at a stage where support is needed, and that it is promising enough to be worthy of that support.
But the joint report has a final caution for the national security apparatus, which have been armed with substantial, if not extraordinary, powers of oversight, control and management of IT systems and technology supply chains. The joint report warns policymakers to “avoid interfering with knowledge or data sharing in ways that could stagnate progress or stifle a fledging field, while protecting national interests, inhibiting advances by hostile entities and maintaining national security advantages”. This is no doubt a difficult balancing act to achieve, but potentially with great benefits if successful.
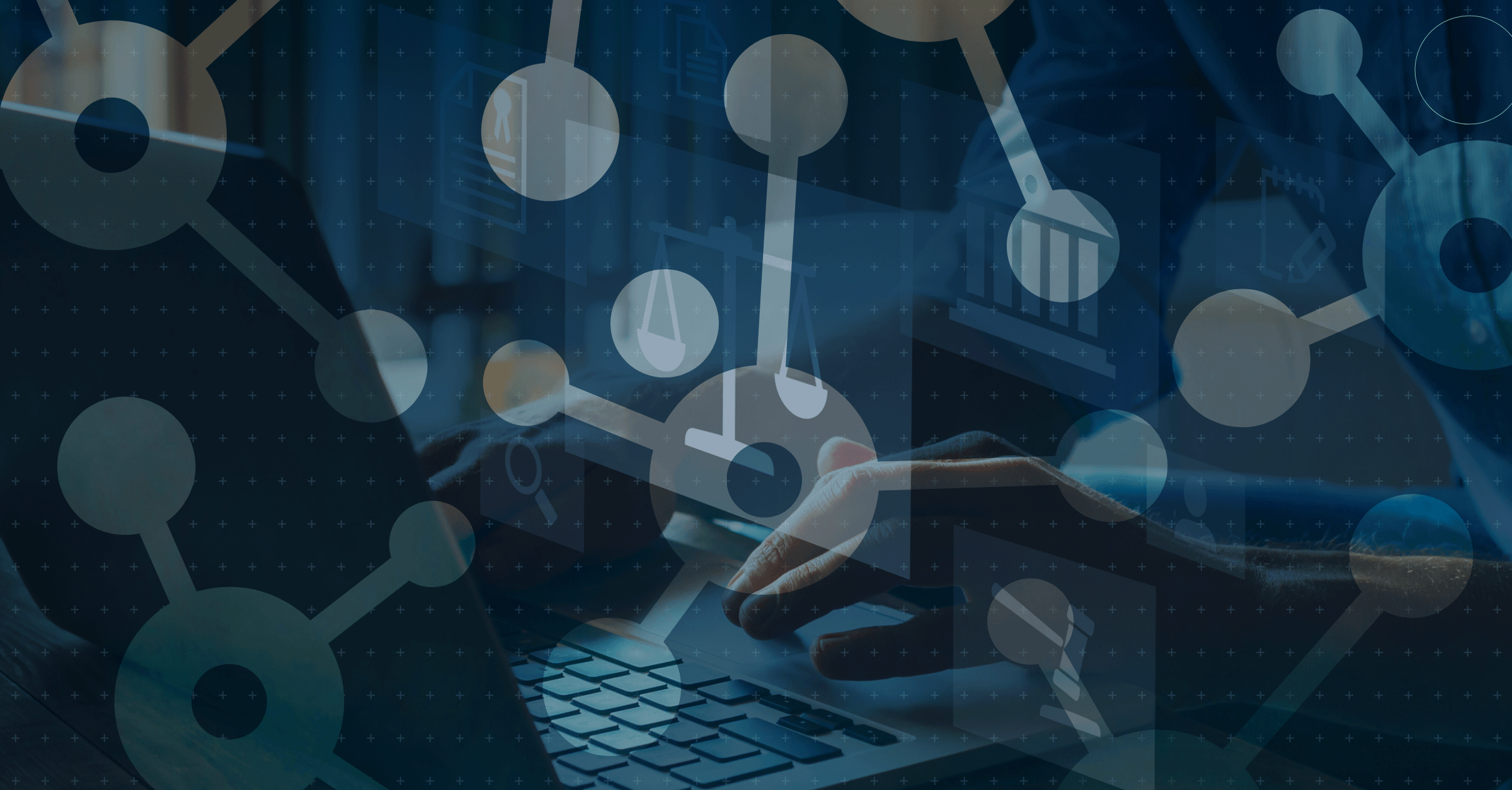
Visit Smart Counsel