The scope of AI in Health (AIH) is huge. It currently includes improved diagnostic precision in radiology via image analysis and the use of robotics to improve the precision of surgery as well as for early lung cancer diagnosis and treatment. AIH is also improving clinician diagnostic precision and patient care outcomes as well as enhancing healthcare delivery, safety and efficiency.
The pattern recognition capabilities of AI allow clinicians to make more informed, personalised treatment recommendations in the management of complex chronic diseases. AI algorithms can process vast amounts of patient data rapidly and with a high degree of accuracy.
The proliferation of wearable technology, smart devices and smartphones equipped with an array of sensors offers an unprecedented opportunity to monitor patient physiology, signs of mental health deterioration and possible adherence to treatment for other medical conditions outside of clinical settings. AI can leverage this data to provide real-time monitoring and personalised recommendations and interventions.
Late last year, Harvard Medical School organised the Responsible AI for Social and Ethical Healthcare (RAISE) conference of 70 health and technology leaders. In the New England Journal of Medicine AI, the RAISE Consortium reported a consensus among conference participants that AIH was strongly beneficial – closer to China’s attitude to AI than to the EU’s focus on AI risk:
“At a moment when the complexity of modern medicine has surpassed the capacity of the human mind, only AIH will be able to perform many tasks. AIH thus seems to offer unparalleled potential for further medical progress, including for precision medicine — the right therapy, for the right patient, at the right time….Some participants reported that this emphasis on benefits contrasted somewhat with a greater European regulatory emphasis on risks.”
Around the same time, an expert panel convened by US federal government health agencies released guiding principles to address the impact of algorithm bias in health and health care.
The view from the health profession
RAISE conference participants were strong of the view that AI should not be considered an additional entity in the traditional clinician-patient dyad – “AI should be seen as a tool supporting the clinician or an aid to patient self-management rather than as a new entity".
That said, the conference participants worried that medical practitioners may still ‘carry the liability can’ when using AIH: “if AIH is to be widely adopted, technology companies must accept some portion of the legal liability if an AIH system leads to harm and it is at fault".
The conference also put a shot across the bow of regulators:
“…according to broad consensus at the conference, professionals and patient advocates will determine best clinical practices and convey them to regulators, rather than vice versa.”
When it came to patient data, the conference participants thought that, because “it is so important to train AIH models with broad and diverse data”, we should lean towards opt-out models for patient information, albeit with clearly explained rights of patients to refuse to say no. The conference participants acknowledged patient concerns over the use of their data but thought that patient trust for opt-out approaches could be built up by measures such as:
- requiring that AI vendors reveal the sources of their training data (e.g. in a nutritional label), in ways accessible to the patients themselves.
- bringing patients, patient advocates, and clinicians into the process of designing the technology at every stage, particularly in setting guardrails.
- developing a well-regulated data economy that tracks provenance and benefits across society, ensuring that all are included in the data pipeline, including marginalised groups.
- clinicians embracing, and positively encouraging, patient self-use of AI to better inform themselves and even “to avoid visiting a provider by using an AI-powered alternative, shifting some time-held practices". One of the real-life stories presented at the conference was of a cancer patient plugging his medical information into a chatbot and learning that his oncologist had misidentified his tumour subtype and thus prescribed the wrong therapy.
The RAISE conference participants also expressed concern that, while AIH developers need to recover their substantial investment, the level and structure of charges for AIH could distort its use:
“Experience shows that payment schemes can influence real-world uptake of medical innovations. Earlier AI tools often met with limited demand, particularly if they were pay-per-use, leading to underuse; there is also a risk, however, that payment models can provide incentives that lead to harmful overuse, either of the AI tool or products recommended by it, regardless of whether the government pays directly for health care.”
They thought that investor “reimbursement should be tied to problems solved and care improvements". Further, they considered that just as drug companies are being pushed to provide medicines for free or low cost to low and middle-income countries, so too AIH developers should recognise the “ethical imperative to focus on the public good” by making AIH available for free or low cost to the disadvantaged.
A new set of guiding principles for AIH
Spurred by the US Congress, the Agency for Healthcare Research and Quality and the National Institute for Minority Health and Health Disparities convened a diverse panel of experts to consider how to systemically address bias in AIH, against the background of some striking early cases:
- a biased algorithm that used race to estimate kidney function resulted in higher estimates for Black patients compared with White patients, leading to delays in organ transplant referral for Black patients.
- a commercial algorithm that risk-stratified patients to determine eligibility for chronic disease management programs effectively required Black individuals to be sicker than White individuals to qualify for such services.
The panel produced 5 principles (the 5 AIH principles) – and while they are not surprising, their significance is that they have been set out in US Government-endorsed ‘tablets of stone’, which means they are likely to become ‘holy writ’ for the medical profession in the US, and probably globally.
Guiding Principle 1: Promote Health and Health Care Equity During All Phases of the Health Care Algorithm Life Cycle
While targeting obvious direct and indirect discrimination in AIH, such as race, this principle takes a much broader view of the equity requirements of AIH:
“an unbiased algorithm is one that ensures patients who receive the same algorithm score or classification have the same basic needs….Health equity is defined ‘as the attainment of the highest level of health for all people. Achieving health equity requires valuing everyone equally with focused and ongoing societal efforts to address avoidable inequalities, historical and contemporary injustices—which includes systemic racism—and the elimination of health and healthcare disparities.’”
This principle requires proactive measures to address the well-known problem of ensuring training data is sufficiently diverse to validate algorithms for fairness in performance across populations. During the operational deployment of an AIH model, ongoing measurement and comparison of outcomes between advantaged and historically marginalised populations or individuals with lower income should be assessed routinely by healthcare systems, algorithm vendors, and the research community and supported by research sponsors (e.g. funders, scientific journals).
This principle also candidly acknowledges the challenges that the inherent characteristics of AI can present unique challenges in using AI models in the health sector:
“Compared to traditional statistical techniques in which statisticians have more manual control over the analyses, artificial intelligence models can be more opaque and more difficult to interpret. They risk being overfitted to the data at hand, threatening generalizability. Artificial intelligence models sometimes lack common sense and are more difficult to audit. Thus, rigorous methods and processes are essential for algorithm development.”
Guiding Principle 2: Ensure Health Care Algorithms and Their Use Are Transparent and Explainable
Explainability, of course, is an all-too-common catch-cry in AI governance, but this principle tries to add a little more meaning to AIH. Explainability should include the following:
- accompanying evidence or reasons for outcomes and approaches or processes.
- explanations that are understandable to individual users.
- explanations that correctly reflect the system’s process for generating the output.
- information that ensures that a system only operates under the conditions for which it was designed, and outputs are only used when the system achieves sufficient confidence in its results.
Transparency should be implemented at multiple layers:
- by developers making publicly available profiles of the data used to train the algorithm, describing the distribution of key aspects of the population in the data set (e.g. race and ethnicity, gender, socioeconomic status, and age);
- by an independent review of the algorithms;
- by health and medical regulators requiring algorithm information labels or model cards sufficient to assess design, validity, and the presence of bias;
- by medical professionals disclosing to patients the purpose of the algorithm and its impacts, whether there are identified biases, and mitigations used to address biases.
With respect to transparency, AI technologies should be as explainable as possible. Striking a balance between full algorithmic explainability which is difficult (even at the cost of some accuracy) and enhanced accuracy (possibly at the expense of explainability) is an important aspect of the technology.
Guiding Principle 3: Authentically Engage Patients and Communities During All Phases of the Health Care Algorithm Life Cycle, and Earn Trustworthiness
This principle says that it is an “ethical imperative to engage with patients and communities around health care algorithms and earn their trust, as these tools can provide great benefit or harm.”
This principle also requires AIH to be built around ‘data sovereignty’ – both of the jurisdiction in which they are deployed and of individual patients. This principle notes that data sovereignty is particularly important to indigenous populations. While not explicitly getting into the opt-in/opt-out debate, this principle seems to take a more conservative approach to the use of clinical data in training AIH than the RAISE conference.
Guiding Principle 4: Explicitly Identify Health Care Algorithmic Fairness Issues and Trade-offs
This principle frankly acknowledges that there is no single, accepted definition of fairness in delivery of their health services, and qualitative trade-offs already are often required in the minds of clinicians in their everyday work. However, the problem with AIH is that different values are often “mathematically mutually incompatible” within AIH programming and training. For example:
“[I]f a particular algorithm use involves choosing a cut-off point for action during model development and implementation, should that cut-off be chosen to maximize sensitivity of the tool to identify someone who might benefit from an intervention, or should it be chosen to maximize specificity of the tool so inappropriate patients are not exposed to unnecessary risk from the intervention?”
This principle takes the following approach:
- fairness in AI should be considered through the lens of distributive justice: i.e. that health outcomes are fairly allocated across the different groups in society.
- trade-offs should be made explicit.
- while technical methods of fairness (such as reweighting people from under-represented groups) can assist, they often “do not translate clearly or intuitively to ethical, legal, social, and economic conceptions of fairness.” Therefore, a broader group of stakeholders and experts needs to collaborate in designing AI for fairness.
Guiding Principle 5: Establish Accountability for Equity and Fairness in Outcomes From Health Care Algorithms
While making the point more subtly than the RAISE conference, this principle says that “[t]here should be redress for persons and communities who have been harmed by biased algorithms”, including legal accountability for AIH developers.
Yet this principle also says that any legal or regulatory framework of accountability and liability for AIH should have incentives to:
- ensure that equity and fairness checks are built into each phase of the algorithm life cycle for both technical bias and human bias that lead to inequities in model performance, clinical outcomes, and resource allocation. Unfair algorithms should be deactivated, removed, or discontinued. Without saying, this seems to suggest some regulatory system of certification for AIH.
- promote innovation and continuous improvement, which also suggests some ‘give’ in legal liability for AI developers and medical professionals.
Ethical principles play a pivotal role for all stakeholders engaged in the responsible advancement, deployment, and assessment of AI technologies for healthcare. This inclusive group encompasses physicians, system developers, healthcare system administrators, health authority policymakers, as well as local and national governments.
Conclusion
The RAISE conference and the 5 AIH principles show both belief about AIH that “this is the future” and an equally strong desire to avoid past mistakes in applying new technology and policies to health care.
Both also stress the urgency in resolving the ethical and governance framework for AIH as the technology is moving at “blistering speed”. As the Raise Consortium put it none too subtly:
“Conclusions reached today may no longer be relevant even 1 year from now. Lives may be saved, however, that would not have been saved even just last year. So, while the conversation around how best to use AIH for public and patient good needs to continue, it also needs to begin now.”
Read more: Attribution-NonCommercial-NoDerivs 4.0 International
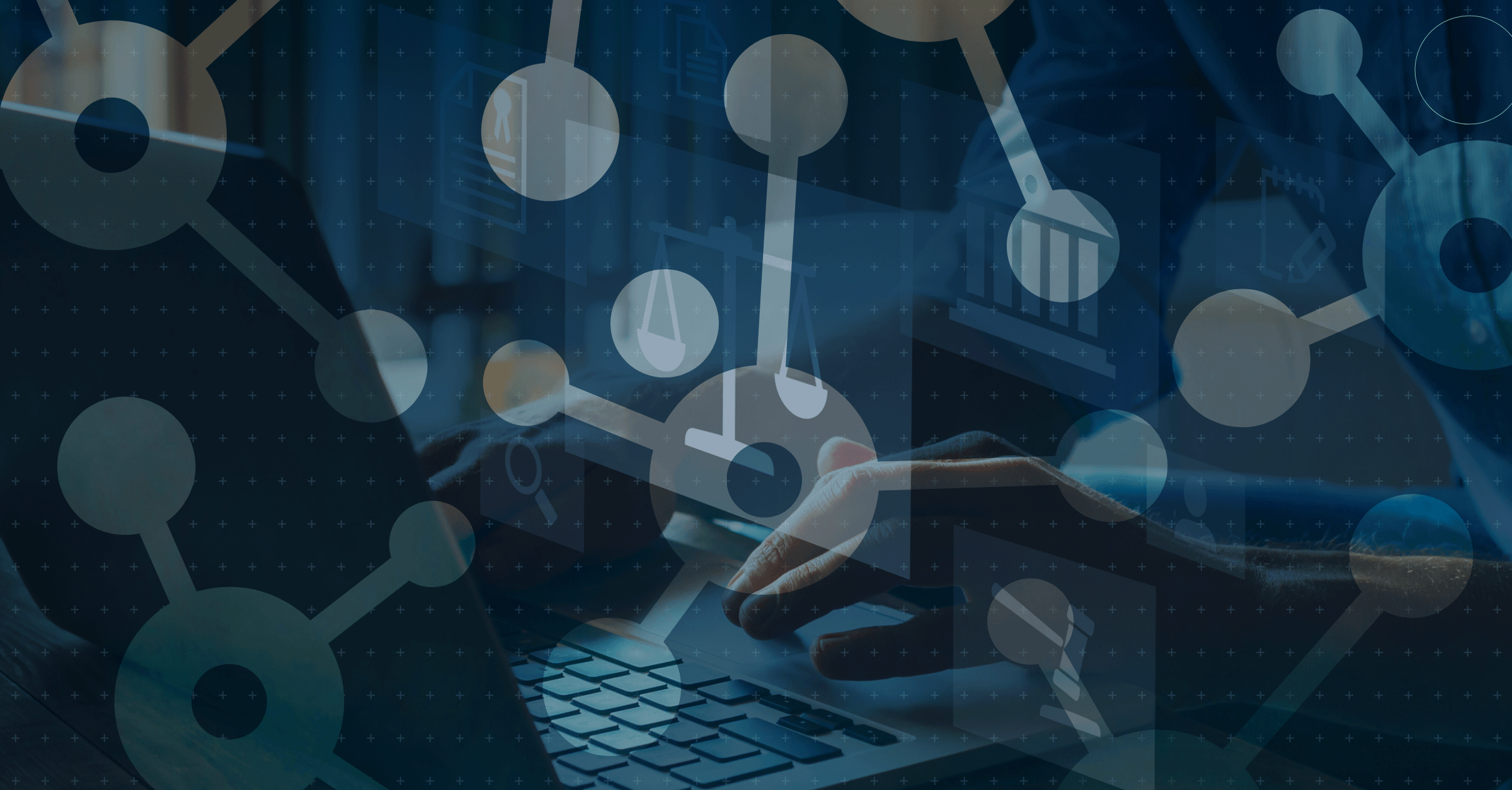
Visit Smart Counsel