As per the last two week’s articles, there is a growing view that the traditional ‘individual harms and rights’ approach to regulation, such as privacy laws, and which underpins new AI laws currently being rolled out, such as the EU’s AI Act, fails to fully address the larger risks of AI. This week we review a paper from the Canadian Institute for Advanced research (CIFAR), which goes one step further and proposes a practical tool - “regulatory impacts analysis” (RIA) - to assist policy makers chart the wider risks to society, including to long-held assumptions underlying current regulatory models.
What the harms paradigm misses
The CIFAR paper describes the tack taken by policy makers in Europe and North America in regulating AI as follows (emphasis added):
As AI begins to play a growing role in the economy, regulators appear to have taken a leaf out of their traditional playbook. They focus on the harms potentially posed by AI and adopt regulatory approaches originally developed in conventional product safety and risk mitigation [which the CIFAR paper calls a ‘harms paradigm’]. Prominent examples include the EU AI Act, which is a quintessential risk categorization and mitigation regime. The Act categorizes AI systems based on their risk levels and imposes corresponding safety requirements. Canada’s Artificial Intelligence and Data Act (AIDA), part of Bill C-27, similarly proposes regulating AI by classifying systems according to their level of impact, imposing the most stringent conditions on high-impact AI systems. The harms paradigm is also dominant in the United States, typified by the National Institute of Standards and Technology (NIST) AI Risk Management Framework, which proposes principles and procedures for AI enterprise risk management.
The paper notes that, to be fair, “[r]egulators are not alone in subscribing to the harms paradigm [and the] discourse among technologists building AI systems and social scientists studying the impacts of AI also reinforces the harms paradigm”.
Like the Stanford and Kennedy School papers, the CIFAR paper makes the obvious but telling point that AI, as a general-purpose technology, has the potential to fundamentally transform society and that framing AI regulation solely in terms of risk mitigation obscures that bigger picture. But the CIFAR paper goes further to say that “[t]he focus on harms and risks of AI as a technology arguably obscures the impact of AI on regulation itself ” (emphasis added).
Disrupting regulation
The CIFAR identifies how AI can disrupt regulation in two dimensions: first, the targets of regulation, i.e. the entities to which regulation applies (or aims to apply); and second the tools of regulation, i.e. the mechanisms used to govern the targets of regulation.
The paper gives the example of the impact of AI on healthcare regulation, which in its current form imposes a range of educational and licensing requirements on indiviudal humans or entities run by humans, including doctors, nurses, and other healthcare providers. The thrust of this regulation is to require healthcare professionals to undergo training and, to varying degrees, demonstrate competence on an ongoing basis. Now, medically-focused or trained AI, such as Google’s Med-PaLM, can perform a variety of biomedical tasks, including mammography and dermatology image interpretation, radiology report generation and summarization, and genomic variant calling. The CIFAR says that this may shift the ‘targets of regulation’:
Tools like this arguably shift the regulatory focus from doctors and conventional healthcare processes to software engineers and the development of AI products and services, raising a host of new questions. For instance, which actors should be required to undergo educational and professional training - human healthcare specialists or AI developers building healthcare applications, or both? Which entity is liable for defective AI generated medical advice? How can responsibility be shared between these different actors?
There are also challenges to the ‘tools of regulation’. The CIFAR paper gives the example of trying to shoehorn medical AI in Canada’s existing risk-based four categories of medical devices (from ranging from low-risk devices like wheelchairs to high-risk devices like defibrillators):
The problem, however, is that AI systems are not necessarily standalone devices or products. They are dynamic tools that are highly sensitive to the contexts in which they are deployed. Seen in this light, how can a regulatory regime designed for evaluating traditional, narrow-purpose medical devices be applied to general-purpose medical AIs? Tracing the causal chain between an adverse outcome and the AI, alongside other human and organizational factors that contribute to that outcome is not trivial. Allocating liability among different actors is equally challenging.
Regulatory impact assessment
The CIFAR paper says that there is no accepted methodology for evaluating the impact of AI on regulatory regimes or systems, and so it proposes a framework as the beginnings of such a tool. This RIA tool can be summarised as follows:
Step 1: identify shift in regulatory targets:
Currently:
who are the primary targets of regulation in your domain? Who else are you responsible for regulating?
Do most regulatory requirements currently apply to these people and/or organizations?
Which actors are not currently regulated but should be regulated?
How will AI technologies and applications change your answers to these questions? Are these changes already taking place? If so, what are the most significant changes to date? In what timeframe do you anticipate further changes to take place?
Step 2: scrutinise your regulatory tools:
Currently:
what are the primary tools, mechanisms, and methods of regulation in your domain?
How do you currently administer and apply these regulatory tools, mechanisms, and methods?
Which regulatory tools do you currently refrain from using, and why?
Again, how will AI technologies and applications change your answers to these questions, in what time fame etc?
Step 3: next steps:
The above Q+A will assist a regulator understand the ‘delta’ between its current regulatory approach and a sector transforming with AI:
regulatory targets in your domain that AI will render less important, and regulatory targets in your domain that AI will render more important.
regulatory tools that AI will render less effective in your domain, and (b) new regulatory tools that should be developed in light of the use of AI in your domain.
That being the case, then the regulator can better work out the new tools and resources it requires and the timeframe in which to aim to achieve them.
The CIFAR paper gives the example of applying its Regulatory Impacts Analysis to regulation of nuclear power plants. Currently, nuclear regulators tend to focus inwards on a small defined set of industry participants that operate nuclear facilities and activities, including engineers and other personnel in these organizations. However, applying the recommended RIA framework, a nuclear regulator could see that they need to look more outwards to a new set of regulatory targets because AI technologies conducting offensive cyber operations could potentially expand the range of malicious actors who seek to gain access to, or exploit, nuclear materials and technologies.
Conclusion
As Australia’s AI expert group gets underway, the trifecta of the Kennedy School, Stanford HAI and CIFAR papers makes the argument for applying a broader lens to the definition of safe and responsible AI. But probably the most interesting perspective from the CIFAR paper is that regulation itself can be disrupted by AI. The ‘utility’ character of AI is well understood to enable it to be applied across all sectors of the economy and society, challenging all sectoral regulators to develop skills in AI. But what less understood is that, as the CIFAR paper points out, this ‘utility’ character also means that AI can upend assumptions on which current sector-based regulation is built. No longer can sectoral regulators rely on there being a limited, fairly readily identifiable group of participants in the sector as the targets of their regulatory efforts, nor can they rely so heavily on regulatory tools which test and monitor competency in the specialist skills required to operate or practice in the sector.
The key takeout from the CIFAR paper is that, given the social and economic disruption that AI can wrought, it would be nave to think that AI will not disrupt regulation itself, and continuing to try to shoehorn AI into existing regulatory models won’t cut it.
The CIFAR paper is authored by Jamie Amarat Sandh, Noam Kolt and Gillian K. Hadfield.
Read more: Regulatory Transformation in the age of AI
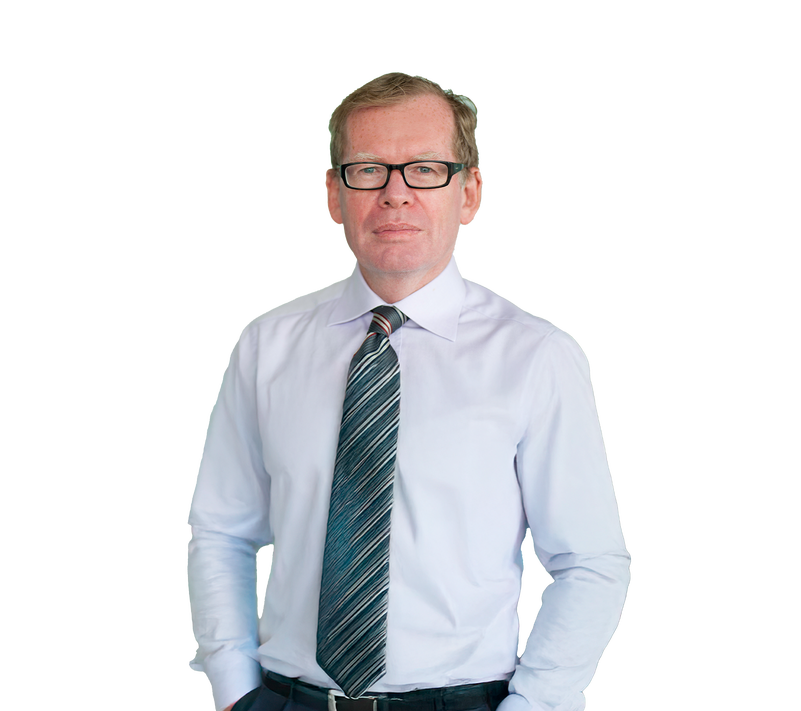
Peter Waters
Consultant