The Australian Communications Minister, Michelle Rowland, has put social media platforms “on notice” to minimise disinformation and scare campaigns in the lead up to the Voice referendum, due between October and December 2023.
While governments and social media platforms are struggling to detect and manage current techniques of misinformation, hate speech and trolling, along comes generative AI models which will enable the creation of sophisticated, hard-to-detect-as-AI content on an industrial scale.
A group of researchers at Georgetown University’s Center for Security and Emerging Technology, OpenAI and the Stanford Internet Observatory have released a study identifying four ways in which generative models will represent a step-up in the already dark and troubling world of online misinformation.
Threat 1: generative models are increasingly authentic
In order to reach a large audience and exert substantial influence, propagandists need to produce large volumes of messaging. In the past, this was time-consuming and required significant human effort. Less-sophisticated models would craft propaganda using ‘copy-pasta’ language, meaning that tweets and posts often would use repetitive and identical language. This type of language generation allowed for high output, but such posts were easily identifiable as created by bots. This made it easier for the social media platforms to detect and remove such posts.
Essentially, you can’t take all of the Russian out of the misinformation posts directed at English native speakers! As the researchers commented:
“Russia’s Internet Research Agency (IRA) accounts, for example, pretended to be Black Americans and conservative American activists, and directly messaged members of each targeted community. Identifying these inauthentic accounts often relies on subtle cues: a misused idiom, a repeated grammatical error, or even the use of a backtick (`) where an authentic speaker would use an apostrophe (‘).”
AI can smooth out these linguistic tell-tale signs – even native speakers find their writing improved passing letters etc through chatGPT!
But more strikingly, large language models can two do other things that human misinformation agents currently struggle with.
First, the misinformation agent’s intention is to create a building storm of messages (trending hashtags) which appear as if they represent the views of the person on the street. But creating the appearance of a ground swell of public appearance requires many speakers who appear ‘authentically’ individual in their messages.
As generative models have become more sophisticated, they have been able to craft endless amounts of unique posts using authentic language – including by using slang or the vernacular of particular groups, which has allowed them to exert influence both more broadly and on specific audiences. These distinct posts are far less detectable, making audiences more susceptible to their messaging.
Second, another tactic of misinformation agents is to have long form propaganda unwittingly republished by more reputable authentic sources, a technique known as “narrative laundering.” But this has mixed success in the past: the Russian GRU’s inauthentic journalist personas began to plagiarize each other’s work (the snake eating its on tail), triggering editorial inquiries from the mainstream media. This forced the Russians to revert to old school approaches of engaging stringers to write stories, who in turn began to suspect the ultimate purpose of the commissioned work.
However, as the researchers point out, “there is already some evidence that existing language models could substitute for human authors in generating long-form content or make content generation more effective through human-machine teaming.”
Threat 2: generative models can engage in individually targeted propaganda
For years now, state-based propagandists have engaged in microtargeting to create divides on particular social issues. For example, during Black Lives Matter they targeted the posting of disruptive content to users who ‘liked’ race-related posts and Facebook groups associated with the movement.
Generative language models can take that to a whole new level. It is even easier to produce personalised content, as AI is able to profile users and obtain information about individuals – from their behaviour patterns, values and vulnerabilities, to even more specific (and invasive) information such as political preferences, sexual identity and race. Hence, as generative models become more sophisticated, propagandists will be able to conduct manipulative campaigns by individually targeting those most susceptible to influence.
Threat 3: generative models can develop content in real time
This means that propagandists will be able to use generative models to push out large volumes of content as soon as an event – whether it be an election, protest or pandemic - happens, and to do so with an instantaneous and disruptive effect. This immediacy, in combination with posts that appear authentic, makes it increasingly difficult for social media companies to detect and remove such posts.
By being able to respond in real time to user messaging and posts, AI may also create new forms of propaganda, such by using personalised chatbots that interact with targets one-on-one and attempt to persuade them of the campaign’s message.
Threat 4: generative models have allowed for the rise of fringe groups
Previously, only larger, state-based actors such as the Russian Internet Research Agency (IRA) had the ability to disseminate traditional forms of propaganda. This is because such propaganda required the resources to develop, produce and distribute misinformation.
However, the emergence of generative models has allowed for smaller, non-state operators to exert the same, if not more, levels of influence as a state-based propagandist, albeit with far lower costs and effort. This is because generative models are becoming increasingly easy to access – take chatGPT3 as an example, which is free and requires little skill to use. This may lead to the rise of smaller propagandists – whether they be members of an organized fringe group, or a sole user operating out of their garage.
But there are limits on AI as a propaganda tool
As the study points out, a current limitation of generative AI models is their failure ‘to consistently produce high-quality text is because they lack awareness of time and information about contemporary events’. AI only ‘knows about’ events that formed part of its massive, pre-release training data. As the researchers say “ask a language system that was trained before COVID-19 about COVID-19, and it will simply make up plausible-sounding answers without any real knowledge about the events that unfolded.” That’s no help to an anti-vaxxer.
Future generative AI models may address this in two ways: either continually retrain models to account for new context, or develop new algorithms that allow for more targeted updates to a language model’s understanding of the world. The researchers note these developments will provide more ‘power to the arm’ of propagandists:
“Since propagandists may be interested in shaping the perception of breaking news stories, significant improvements in how language models handle recent events not present in their initial training data will translate directly into improved capabilities for influence operators across a wide number of potential goals.”
How do we protect ourselves?
The study starts from an acceptance that the increasing use and sophistication of generative models is inevitable, including by propagandists.
The study presents a number of mitigations, including:
- Marking social media content with the original generative model that was used to create it, so as to assist digital platforms with self-regulation. This would involve ‘finger printing’ each large language model, such as by introducing ‘statistical perturbations’, thereby distinguishing its outputs from normal text. The researchers acknowledge that it may be difficult impress that fingerprint on individual social media posts unless such fingerprints very sophisticated: if the patterns permitting such detection were possible, they risk being clear enough for operators to screen out. However, it may be possible to trace back a large body of related misinformation to a particular AI model;
- Training generative models to be fact-sensitive in the first instance, so as to minimize disinformation. Currently, large language models prioritise ‘realism’ – the extent to which text effectively mimics human text in the training data, without inherent regard for the truthfulness of the claims that it makes. It may be possible to train AI models in such a way that they are incentivized to make more factually grounded claims. However, the study also concedes that the most effective misinformation campaigns are those which have a ‘kernel of truth’;
- Social media platforms to adopt rules about AI generated content. The study recognized that a blanket ban on posting AI-generated content to social media would be unrealistic – some uses would be legitimate or fun, such as comedy bots. The study suggests (without a landing) that social media platforms could consider rules requiring AI-generated content to be flagged. But this comes full circle to how do social medial platforms enforce this rule because, without something like finger printing by AI developers, the social media platforms are left to try to detect AI-generated content through statistical patterns or user metadata. The study suggests social media platforms could collaborate to detect larger scale AI-generated misinformation campaigns, but that itself caries competition law and other concerns.
- Digital Provenance Standards to be widely adopted. Because technical detection of AI-generated text is challenging, the researchers propose an alternate approach “to build trust by exposing consumers to information about how a particular piece of content is created or changed.” The researchers acknowledge that this intervention requires a substantial change to a whole ecosystem of applications and infrastructure in order to ensure that content retains indicators of authenticity as it travels across the internet, but they point to the work of the Coalition for Content Provenance and Authenticity (C2PA) which has brought together software application vendors, hardware manufacturers, provenance providers, content publishers, and social media platforms.
- Proof of ‘personhood’ to post, such as ‘video selfies’ when opening an account or biometric information. However, the study acknowledged that misinformation agents like Russia’s IRA could soon find human lackies to satisfy the criteria, while the rest of us could face escalated privacy risks.
- Governments to ‘harden up’ against misinformation. When seeking public input, public agencies should ensure they are armed with the tools and sophistication to recognize AI-generated propaganda, and act accordingly to identify and remove it. Moreover, the study says politicians themselves need to learn to be more robust; they are currently reactive to a limited amount of noise because they believe it reflects a large portion of public opinion. With generative models now able to spread large volumes of divisive content, this sentiment no longer holds.
- The public to be educated to detect misinformation. The study points out that existing digital literacy courses will need to be revised to cope with AI because they often focus on ‘tell tale signs’ such as a lack of personalisation of messages, whereas the strength of AI is the degree of personalization it can achieve. The study argues that “[j]ust as generative models can be used to generate propaganda, they may also be used to defend against it [through] consumer-focused AI tools could help information consumers identify and critically evaluate content or curate accurate information.” These could be built into browsers and mobile apps, and include “contextualization engines” which would enable users to quickly analyze a given source and then find both related high-quality sources and areas where relevant data is missing.
Unsurprisingly, the study concludes that “there are no silver bullets for minimizing the risk of AI-generated disinformation”. But the study adds that this is not an excuse for defeatism:
“Even if responding to the threat is difficult, AI developers who have built large language models have a responsibility to take reasonable steps to minimize the harms of those models. By the same token, social media companies have a continuing obligation to take all appropriate steps to fight misinformation, while policymakers must seriously consider how they can help make a difference. But all parties should recognize that any mitigation strategies specifically designed to target AI-generated content will not fully address the endemic challenges.”
Conclusion
At the end of the day, addressing misinformation comes back to us as consumers. As the study puts it, “mitigations that address the supply of mis- or disinformation without addressing the demand for it are only partial solutions.”
Yet the researchers are also realistic enough to concede that personal responsibility by itself only goes so far because basically as individuals we will never be proactive enough, enough of the time:
“From a selfish perspective, ignorance is often rational: it is not possible to be informed on everything, gathering accurate information can be boring, and countering false beliefs may have social costs. Similarly, consuming and sharing disinformation may be entertaining, attract attention, or help an individual gain status within a polarized social group. When the personal costs of effortful analysis exceed the personal benefits, the likely result will be lower-quality contribution to group decision-making (e.g.,sharing disinformation, free riding, groupthink, etc.).”
Authors: Anjali Wijeratne and Peter Waters
Read more: https://arxiv.org/abs/2301.04246
KNOWLEDGE ARTICLES YOU MAY BE INTERESTED IN:
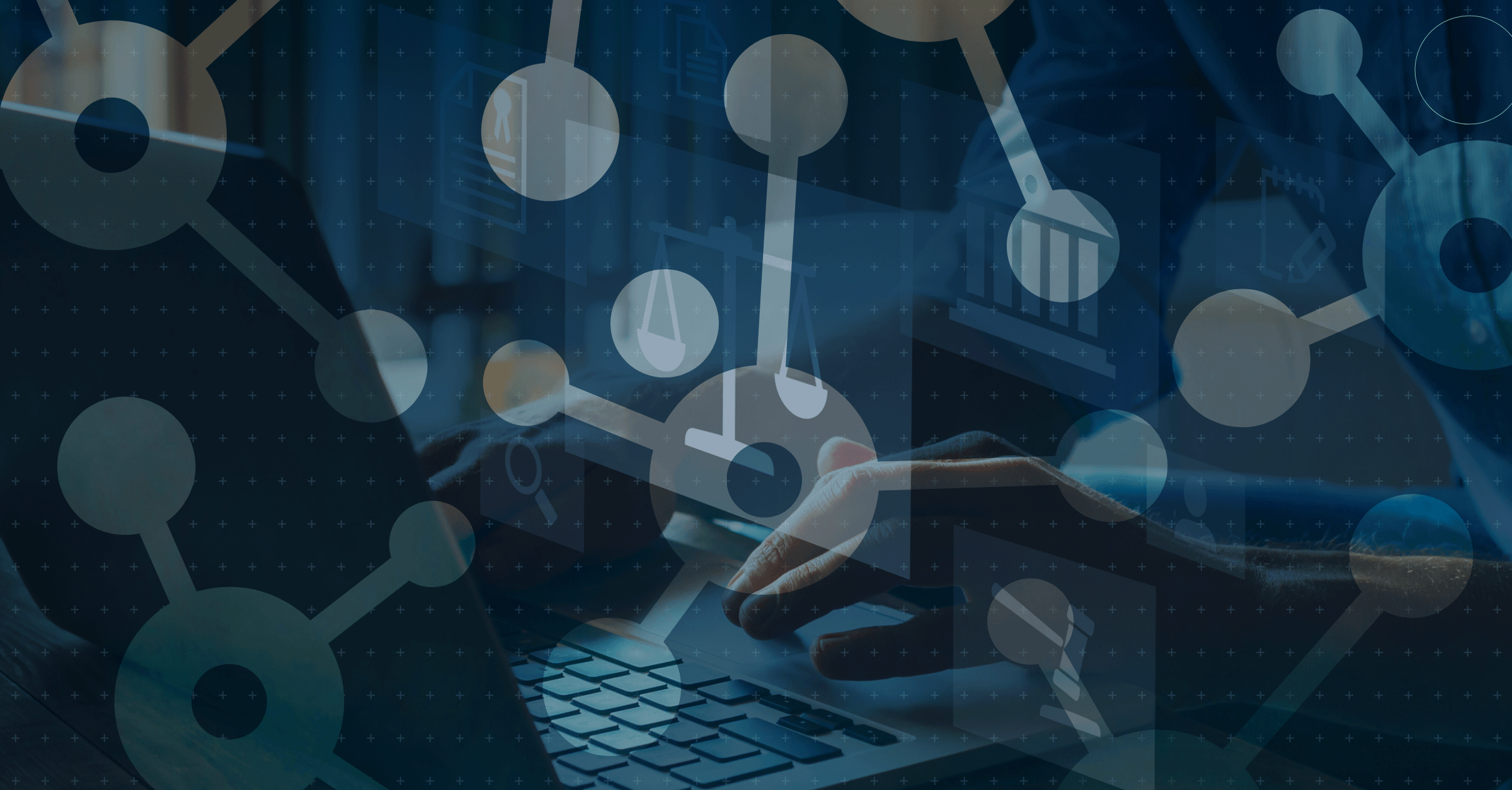
Visit Smart Counsel