Researchers from Stanford University and the Massachusetts Institute of Technology (Erik Brynjolfsson, Danielle Li and R. Raymond) have published the results of the world’s first large scale empirical study of the impact of workers using generative AI.
The researchers studied the phased deployment of a GPT chat assistant tool supporting over 5,000 customer support agents (‘help desk’ function) in a Fortune 500 software firm that provides business process software.
Why will the impact of AI on work be different from earlier technologies?
The researchers see earlier computer technologies as substitutional for lower paid human workers:
Computers have historically excelled at executing pre-programmed instructions, making them particularly effective at tasks that can be standardized and reduced to explicit rules. Consequently, computerization has had a disproportionate impact on jobs that involve routine tasks, such as data entry, bookkeeping, and assembly line work, and reducing demand for workers performing routine tasks. At the same time, computerization has also increased the productivity of workers who possess complementary skills, such as programming, data analysis, and research. Together, these changes have contributed to increasing wage inequality in the United States and have been linked to a variety of organizational changes.
However, the researchers posit that AI could have a very different impact on the labour market:
In contrast, recent advances in AI, particularly those driven by generative AI, suggest that it is possible for LLMs to perform a variety of non-routine tasks such as software coding, persuasive writing, and graphic design.Since many of these tasks are currently performed by workers who have either been insulated or benefited from prior waves of technology adoption, the expansion of generative AI has the potential to shift the relationship between technology, labor productivity, and inequality.
Of course, this could mean AI will substitute for higher skilled workers insulated from previous technology changes. However - and spoiler alert - the researchers tend to see, based on the results of their study, a more complimentary relationship between AI and human workers.
Use of AI to support help desks
We all experience long queues for a human operator, of operators who cannot seem to answer the most basic questions, and of a ‘round robin’ of call transfers to find someone who can answer our question.
The researchers note that AI is well suited to customer support functions:
From an AI’s perspective, customer-agent conversations can be thought of as a series of pattern-matching problems in which one is looking for an optimal sequence of actions. When confronted with an issue such as I can’t login, an AI/agent must identify which types of underlying problems are most likely to lead a customer to be unable to log in and think about which solutions typically resolve these problems (Can you check that caps lock is not on?). At the same time, they must be attuned to a customer’s emotional response, making sure to use language that increases the likelihood that a customer will respond positively (that wasn’t stupid of you at all! I always forget to check that too!). Because customer service conversations are widely recorded and digitized, pre-trained LLMs can be fine-tuned for customer service using many examples of both successfully and unsuccessfully resolved conversations.
The researchers also noted that there is a high turnover of customer support staff and that newer workers are less productive and require intensive training and supervision - the average supervisor spends at least 20 hours a week training poorer performance agents. AI can, in effect, ‘sit on the agent’s shoulder’ coaching them with better or more accurate responses or suggestions for questions to get further information from the customer.
The researchers considered that the AI could lift the level of service across the help desk staff. On deployment, the AI model starts out being trained on human-generated data in a setting where there is high variability in the abilities of individual agents. Over time, the model identifies patterns that distinguish successful from unsuccessful calls, which means it is implicitly learning the differences that characterize high- versus low-skill workers. For example, the researchers found that top-performing agents identified the need for a problem to have a research answer twice as quickly as the agents, and the AI will learn to prompt all agents to do that.
What the study showed
The phased rollout of the new AI chat tool allowed the study to measure, in effect, the ‘before and after’ of AI. The study examined the conversation text and outcomes associated with 3 million chats by 5,179 agents, of which 1.2 million chats by 1,636 agents were post-AI deployment.
Improved productivity
The study found that:
access to AI increases the number of resolutions per hour (RPH) by 0.47 chats, up 22.2 percent from an average of 2.12. When normalising for the fact that agents have different levels of experience, the deployment of AI increases RPH by 0.30 calls or 13.8 percent.
as would be expected, there is a substantial and immediate increase in productivity in the first month of deployment. But the study found the effects enduring, with the RPH rate growing slightly in the second month and remaining stable up to the end of the study.
the productivity gains were not just because each call was shorter, but it also appears the AI improved the ability of agents to ensure in multi-tasking more than one call. So, while call duration fell by 9%, the number of chats the agents handled per agent increased by a larger 14%.
the biggest increases in productivity were for lower skilled workers. The study divided agents into quintiles using a skill index based on their average call efficiency, resolution rate, and surveyed customer satisfaction in the quarter prior to the adoption of the AI system. The help desk workers in the lowest quintile saw an RPH increase of 35%, while there was not a material productivity gain for the highest skilled workers.
The use of AI also accelerated the productivity of newly employed staff. The study compared new workers who were never given access to the AI to those who were given access on commencing work. Both groups started with an RPH of 2 per hour and those without AI took 8-10 months to reach an RPH of 2.5 while the AI-assisted workers reached that rate after 2 months.
Agents using AI also seemed to become better at resolving customer inquiries on their own without needing to escalate to supervisions, with the escalation rate dropping by 25% post-access to AI.
How is AI changing service delivery?
As the purpose of the chatbot was to augment - rather than replace - the human agent, a logical question is how frequently did the agents follow the suggestions of the AI. Agents were coded as having adhered to a recommendation if they either click to copy the suggested AI text or if they self-input something very similar.
Surprisingly, the study found a high level of non-adherence to the AI recommendations: the average adherence rate is only 38 percent, which the researchers observe is similar to the share of other publicly reported numbers for generative AI tools.
But the study noted two interesting aspects behind this apparently low average figure.
First, the productivity improvements were closely correlated to a worker’s frequency in adopting the AI recommendations. Agents were divided into quintiles based on frequency of adoption. Those in the lowest quintile (i.e. those that ignored the AI the most) see a 10 percent gain in productivity, but agents in the highest quintile see productivity gain close to 25 percent.
Second, over time all workers increase their adherence to the AI recommendations. Initially, more senior workers are less likely to follow AI recommendations: 30 percent for those with over a year of tenure compared to 37 percent for those with under three months of tenure. But over time, the rates of AI adoption increase across the board, with more senior workers accelerating their adoption more quickly so that over time all skill levels converge at similar levels of adoption. As the researchers note, this suggests the more senior workers soon overcome their scepticism that they know better than the AI.
As noted above, the researchers hypothesised that AI should ‘raise all boats’ because it learns from the best agents what works best. The researchers tested this by a textual analysis of the notes made by agents before and after access to AI to compare how an individual agent’s conversations change over time (e.g. within-person similarity over time), as well as how high and low-skill agents’ conversations compare with each other over time (between-person similarity).
The results were:
the within-person textual difference is only 0.3, indicating that, on average, individual agents continue to communicate similarly after the deployment of the AI model. This suggests that AI has helped agents be more productive in delivering more or less the same answers they did before. But drilling down, lower-skill agents more clearly shift their language more after AI model deployment relative to initially higher-skill workers. As the researchers say, ‘[i]f AI assistance merely leads workers to type the same things but faster, then we would not expect this differential change”.
more strikingly, the between-person textual difference between high- and low-skill workers narrowed pre and post access to AI, which suggests that the AI was, as posited by the researchers, transferring knowledge from the higher skilled workers to the lower skilled workers.
Satisfaction levels amongst customers and workers
It is one thing for AI to help push through service requests more quickly, but are customers - and the agents themselves - happier with the service outcomes? That, of course, is the ultimate metric for a business because “customer support interactions are important for maintaining a company’s reputation and building strong customer relationships”. To assess this, the researchers used a sentiment measure tool, SiEBERT, “to capture the affective nature of both agent and customer text.”
The study found “an immediate and persistent improvement in customer sentiment” post-AI.
By contrast, the study did not detect a material improvement in the sentiment of agents in using the AI tool. But interestingly, the study found that the use of AI appeared to substantially reduce the attrition of agents. As the researchers astutely note:
“Increases in worker-level productivity do not always lead workers to be happier with their jobs. If workers become more productive but dislike being managed by an AI assistant, this may lead to greater turnover. If, on the other hand, AI assistance reduces stress, then workers may be more likely to stay.”
The study found that, on average, the likelihood that a worker leaves in the current month goes down by 8.6 percentage points. But more strikingly, amongst workers with less than 6 months employment, the study found the attrition rate fell from about 25 percent to 10 percent.
Conclusions
The researchers conclude that the study shows that “[p]rogress in machine learning opens up a broad set of economic possibilities”, which can be very positive for businesses, employees and customers.
The researchers say that this is mainly driven by the AI system’s ability to embody the best practices of high-skill workers, which traditional, human-based organisational processes in businesses have had difficulty disseminating because these best practices involve tacit knowledge. The researchers conclude that AI assistance leds to substantial improvements in problem resolution and customer satisfaction for newer- and less-skilled workers, but does not help the highest-skilled or most-experienced workers on these measures.
In effect, AI, ‘mines’ the skills and knowledge of the better skilled workers. The researchers say that this raises an equity issue between differently skilled workers and the company. The researchers pose the question (without proving an answer) “whether and how workers should be compensated for the data that they provide to AI systems.”
Read more: Generative AI at work
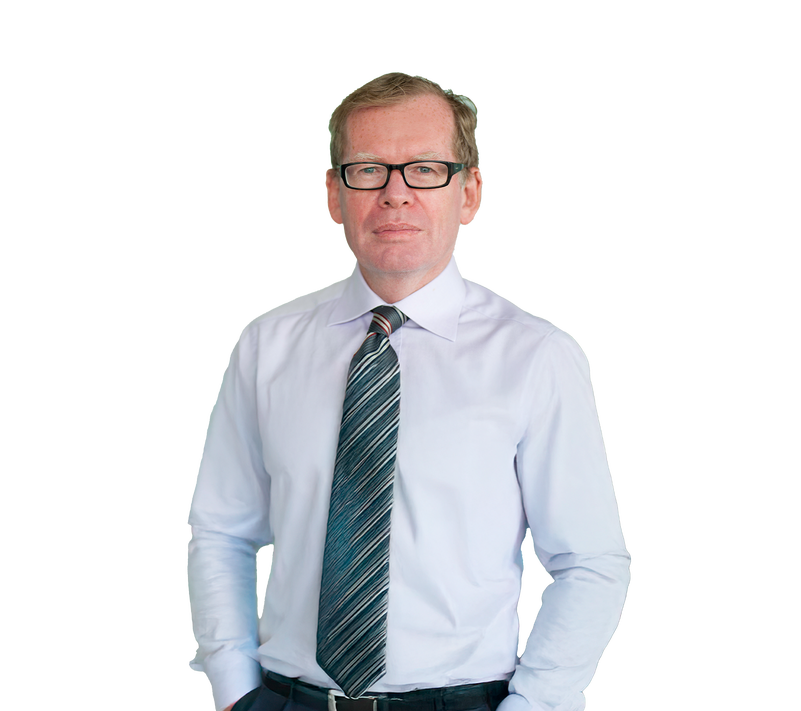
Peter Waters
Consultant