Picture this: You’re having a heated conversation on social media, when suddenly you find yourself locked out of your account. The reason? An algorithm has deemed your words “offensive”.
If someone was to post on social media 'I am Muslim', is that offensive? Is ‘I am Buddhist’ less offensive?
Are terms such as ‘gay’, ‘Jew’, ‘refugee’, or ‘black person’ more associated with hate speech in one language compared to another?
A recent study led by the European Union Agency for Fundamental Rights (FRA) tested different models of offensive speech detection algorithms in English, German and Italian to test whether they had inbuilt ‘biases’ - and as result, over-censored or under-censored speech. The results are, as the FRA study says, ‘telling’, if not truly eye-opening.
Offensive Speech detection algorithms have come a long way, but.
These algorithms use machine learning technology to detect offensive language through natural language processing (NLP) - the ‘genus’ of AI to which chatGPT belongs.
Back in 2018, Mark Zuckerberg told the US Congress that AI wasn't ready to detect hate speech on Facebook. The platform relied on users to report it instead. Fast forward to 2022, Facebook's detection of AI hate speech has substantially improved, detecting 96% of overall hate speech detections via algorithms. Although AI helps flag content, human moderators still ultimately decide on the next steps.
The central problem with offensive speech detection is that learning algorithms can struggle to consider the context of a potentially offensive word. A result, they may unjustifiably trigger the blocking mechanism or miss usage where they should block. Even we humans can struggle with context - how many times have politicians pleaded that their remarks have been taken ‘out of context’.
The Study
The FRA study tested three models:
A standard methodology based on the words that occur, without considering the order or surrounding words: basically a ‘bag of words’ approach;
A methodology that looks at existing semantic relationships between words; and
3. The most advanced methodology that varies the relationship between words depending on neighbouring words and sentence predictions.
The three models were applied in conjunction with a parallel human review team. Unwanted bias in the offensive speech detection models was tested by looking at both the false positive rate (FPR), when the human team considered that the AI flagged ‘innocent speech’ as offensive - in effect, the FPR indicates the potential for unwarranted censorship where the AI is ‘overreacting’ - and the false negative rate (FNR), when the human team flagged offensive speech that sailed past the AI.
Identity terms discrimination in English
Speech detection algorithms rely heavily on certain words as indicators of offensiveness, usually associated with an identity such as Muslim or queer.
Figure 8 form the FRA report shows the results for groups of identity terms used to test the models for the English-language dataset and models: the upper pane is the FPR and the lower pane (which seems to be mislabelled in the original report) is the FNR.
The FRA study concluded that this showed the risks of bias (in this case being ‘too sensitive’ to the protected status) being embedded in the training data, and because model 1 only uses training data, it ends up being worse. Models 2 and 3 mitigate the impact of the training data because they also refer to or learn from external data (such as the general Internet) and are able to learn to make more ‘nuanced’ judgments (e.g. that LGBTQIA people can use the term ‘queer’ in a positive sense) - expect for the term ‘Jew’ as discussed below.
It is not a ‘zero sum game’ between FPR and FNR. The terms ‘Muslim’, ‘gay’ and ‘Jew’ attract a low rate of falsely treating comments using these terms as unoffensive (ie they had a low FNR), which might seem logical because the algorithms over classified so many comments as offensive. But while the three models have a low error rate (the FPR) in falsely predicting other terms (e.g. gendered terms) as being used in offensive comments, the lower pane shows that the algorithms (the FNR) miss about half of the instances in which the human teams detected those other terms being used in an offensive way. In other words, when the algorithms caught language as being offensive, they were usually right, but they also missed a lot.
Identity terms discrimination in German and Italian?
The English-language models’ predictions differ hugely for the identity terms compared to Italian and German.Figure 9 from the FRA report shows the FPR and the FNR for German across similar terms to Figure 8 (again the lower pane seems mislabelled and should be FNR):
While the algorithms seriously overreacted to the identify terms ‘gay’ and ‘Jew’ in English, they made very few errors in calling out use of the German versions of those terms as offensive. But conversely, in German they missed on average half of the times the terms were used in an offensive way.
In Italian, an even higher average of 66-85 % of comments were rated as non-offensive by the human team were predicted to be offensive by the three models - in particular, terms linked to Muslims, Africans, Jews, foreigners, Roma and Nigerians.
These different outcomes across languages does not say that Germans or Italians are any more or less biased or unpredictable in how they speak of minority groups. What it does show is that the availability of research and NLP tools in languages other than English is lagging far behind their availability in English.
When AI learns from our biases
The FRA study also shows what offensive detection language algorithms can pick up from external data.
Take the statement “I am [insert identity]”. "I am Christian" is predicted by all models to have a very low probability of being offensive (between 2% and 9%). The reason for this may not be attributed to the offensive nature of the comments including 'Christian', but instead that due to the fact that 'Christian' happens to be a popular male name.
Under models 1 and 2, which primarily rely on training data, “I am Buddhist” and “I am Jewish” carries a somewhat higher prediction associated with offensive speech, but mostly is predicted to be inoffensive. However, the FRA notes that model 3, which is probably has the greatest exposure to the ‘real world’ of human communication produces very different results:
This model is based on existing language models, which apparently have learned that the term ‘Jewish’ alone signifies an offensive comment. This tendency is even stronger when it comes to the term ‘Muslim’, which has an average prediction of being offensive of 72 %.
Offensive language algorithms can also struggle when nice words are added as ‘air cover’ for offensive language. As the FRA report comments:
The fact that the word ‘love’ reduces the likelihood of content being rated as offensive was discussed by researchers, and can be used to evade hate speech detection algorithms. In our example, the phrase ‘Kill all Europeans’ is rated as 73% likely to be offensive. The phrase ‘Kill all Europeans. Love’ is predicted to be only 45% likely to be offensive. Simply adding the word ‘love’ may mean that text is predicted to be non-offensive (depending on the threshold for offensiveness).
Gendered language
Detecting gender bias in languages which grammatically have masculine or feminine constructions is tricky. For example, in French all nouns have a gender, even things, and the gender of nouns can seem unpredictable: le livre (masc) is a book and la voiture (fem.) is a car.
The FRA study found if a gendered sentence or word is included, masculine terms lead to lower predictions of offensiveness, indicating a slight tendency for more bias against women in the training data. However, the results also show differences in error rates by gender across the models, indicating different gender biases in the word embeddings and available language models.
The FRA study shows that the focus on English in NLP development and analysis also brings the challenge of developing tools or using approaches that do not work in other languages which can be more context sensitive.
Recommendations
The FRA study noted that considerable progress has been made by social media platforms such as Facebook in Natural Language Processing - moving beyond a crude ‘bag of words’ approach to crack the problem of reading words in their context. However, the FRA study noted that:
More advanced methodologies, using word correlations from other data sources, can mitigate this issue to some extent. However, these advanced methodologies rely on existing general-purpose AI tools, which suffer from bias as well. So, these may not necessarily mitigate bias. Rather, they could increase or introduce certain biases.
The study results also illustrated the fine balance to be made in assembling and testing the training data: this can cut both ways, with either too few or too many examples of offensive speech embedded in the training data.
Ultimately, the report concludes that to get to fully automated offensive language detection, “further work is needed to safely use this technology without risking increasing discrimination against historically disadvantaged groups”, either by over-censoring or under-censoring. In the meantime, the FRA study concludes that “the content moderation decisions need to remain in the hands of well-trained humans”.
So, if you ever find yourself in a social media standoff and you find yourself locked out, maybe what you said wasn’t offensive after all. Maybe it’s just that selfie from Mardi Gras.
Read more: Bias in algorithms - Artificial intelligence and discrimination
Authors : Hannah Pearson, Molly Allen and Peter Waters
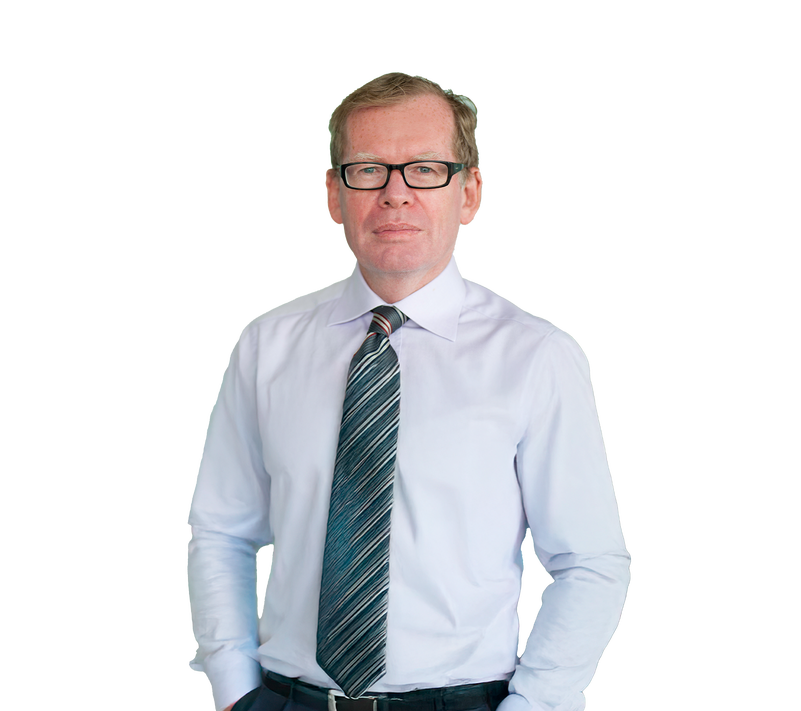
Peter Waters
Consultant