The Intergovernmental Panel on Climate Change (IPCC) has been successful in laying down a baseline of scientific facts for global discussions on climate action.
In an apparent attempt to emulate that model, the United Kingdom (UK) and South Korean governments released at the May 2024 Seoul summit on AI safety an interim 130-page independent expert report. The interim report is touted as bringing together "for the first time in history... experts nominated by 30 countries, the European Union (EU), and the United Nations (UN), and other world-leading experts, to provide a shared scientific, evidence-based foundation for discussions and decisions about general-purpose AI safety".
The Chief Scientist at Australia’s national science agency, the CSIRO, describes the report as "brave and insightful". While the panel members "continue to disagree on several questions, minor and major, around general-purpose AI capabilities, risks, and risk mitigations", a clearer picture of the challenges - and the unknowns - we currently face with AI emerges from the interim report.
Current capabilities and limitations of AI
The interim report kicks off with a reminder of how fast, how far, and how startling the development of AI capability has been:
In 2019, the most advanced Large Language Models (LLM), could not reliably produce a coherent paragraph of text and could not always count to ten. At the time of writing, the most powerful LLMs like Claude 3, GPT-4, and Gemini Ultra can engage consistently in multi-turn conversations, write short computer programs, translate between multiple languages, score highly on university entrance exams, and summarise long documents.
Nonetheless, the interim report’s assessment of current AI capability is that today’s general-purpose AI systems show "partial proficiency but are not perfectly reliable". The following table summarises the panel’s analysis through the lens of 'capability-limitation pairs':
Will the current pace of AI capability continue into the future?
The report records a fundamental difference in opinion between the panel members over whether AI capabilities will continue to escalate, even in the near term. Some experts think a slowdown of progress is by far most likely, while other experts believe that extremely rapid progress is possible or likely.
This difference in view comes down to whether continuously increasing computing and data resources used to train new AI models and refining existing algorithms will blow through the limitations set out in the table above.
The experience of AI development over the last five years indicates the existence of a direct relationship between the amount of training data, the size of the computing power thrown at that data, and the model size (the number of parameters) and model performance, called scaling laws:
The amount of computing power needed to train an AI model in 2023 is 10 billion times the compute used to train AI in 2010, and the compute used for training is doubling every 6 months.
In 2017, the original Transformer model was trained on two billion tokens (a token = word) while 2023 models are trained on 3 billion or more tokens, and training data sets are growing 2.5x per annum.
Improved algorithmic efficiency means that in language modelling, the computation required to reach a fixed performance level has halved approximately every eight months on average since 2012.
Scaling can, in effect, be compounded post-training. Post-training algorithms introduced through fine-tuning can significantly improve general-purpose AI model capabilities at low cost: many post-training algorithms improved model performance on a given benchmark by more than 5x result from using the training compute, and in some cases more than 20x.
The AI capability optimists also point out that the scaling laws are not linear: i.e. when AI models achieve large scale; new, advanced capabilities emerge unexpectedly (and inexplicably), which the interim report describes as follows:
There are many documented examples of capabilities that appear when models reach a certain scale, sometimes suddenly, without being explicitly programmed into the model (120, 121, 122*, 123). For example, large language models at a certain scale have gained the ability to perform the addition of large numbers with high accuracy, when prompted to perform the calculation step-by-step. Some researchers sometimes define these as ‘emergent’ capabilities."
As the report notes, many AI developers are betting big on the scaling laws continuing to deliver by the end of 2026. Some general-purpose AI models will be trained using 40x to 100x the computation of the most compute-intensive models currently published, combined with around 3 to 20x more efficient techniques and training methods.
However, the report also records the emerging view that the scaling laws are not the recipe of an AI ‘magic pudding’:
The whole notion of ‘emergent capabilities’ is challenged. More recent studies using more finely calibrated metrics suggest these capabilities appear more gradually and predictably.
There are also examples of ‘inverse scaling’ where language model performance worsens as model size and training compute increase, e.g. when asked to complete a common phrase with a novel ending, larger models are more likely to fail and simply reproduce the memorised phrase instead.
Most tellingly, the scaling laws may simply run out of ‘puff’:
With AI models already trained on a third or more of the Internet, we could simply run out of training data. While running the AI through several turns of the same training data (called epochs) could help, AI can become overfamiliar with data on which it is trained too many times (called overfitting), reducing its ability to respond to a wider range of prompts. Synthetic data (generated by AI for AI) has some promise, but also "could reduce meaningful human oversight and might gradually amplify biases and undesirable behaviours of general-purpose AI models".
While the leading technology companies have the financial resources to "scale the latest training runs by multiples of 100 to 1,000... further scaling past this point may be more difficult to finance".
The increasing energy demands for training general-purpose AI systems could strain energy infrastructure. Globally, computation used for AI is projected to require at least 70 TWh of electricity in 2026, roughly the energy consumed by Austria or Finland.
There are supply constraints in the physical infrastructure. AI chip manufacturing depends on a complex, hard-to-scale supply chain, featuring sophisticated lithography, advanced packaging, specialised photoresists, and unique chemicals, and a new chip manufacturing plant can take three to five years to set up. Even if these supply bottlenecks can be solved, individual GPU performance also may slow due to physical limits on transistor size.
But the real driver of the pessimistic view that AI capability will slow is that the current ‘deep learning’ approach is a dead end:
current deep learning systems are thought by some to lack causal reasoning abilities, abstraction from limited data, common sense reasoning, and flexible predictive world models. This would suggest that achieving human-level performance in general-purpose AI systems requires significant conceptual breakthroughs and that the current type of progress, driven by incremental improvements, is insufficient to reach this goal."
A primary advocate of this view is Facebook’s Chief AI scientist, Professor Yann LeCun:
It’s astonishing how [LLMs] work if you train them at scale, but it’s very limited. We see today that those systems hallucinate, they don't really understand the real world. They require enormous amounts of data to reach a level of intelligence that is not that great in the end. And they can't really reason. They can't plan anything other than things they’ve been trained on. So, they're not a road towards what people call 'Artificial General Intelligence (AGI)'. I hate the term. They're useful, there's no question. But they are not a path towards human-level intelligence."
The interim report observes that if this view, that progress depends on new breakthroughs is correct, then advances in AI capability could soon slow to a crawl:
Fundamental conceptual breakthroughs resulting in significant leaps in general-purpose AI capabilities are rare and unpredictable. Even if novel techniques were invented, existing general-purpose AI systems’ infrastructure and developer convention could provide barriers to applying them at scale. So, if a major conceptual breakthrough is required, it could take many years to achieve."
However, to straddle these opposing views, the report argues that:
These opposing views are not necessarily incompatible: although current state-of-the-art deep learning systems broadly have weaker reasoning abilities than most humans, we are seeing progress from one generation of a general-purpose AI model to the next, and many AI researchers are exploring ways to adapt general-purpose AI models to unlock or improve ‘system 2’ reasoning (analytic, rule-based, and controlled reasoning) and ‘autonomous agent’ abilities. Progress on these capabilities, if it occurs, could have important implications for AI risk management in the coming years."
Next instalments
Next week we will look at the interim report’s assessment of risks, and the week after we look at its views on technology mitigants to those risks.
As a taster, the report says that while nothing about the future of AI is inevitable, "no existing techniques currently provide quantitative guarantees about the safety of advanced general-purpose AI models or systems".
But the Irish member of the panel, Ciarn Seoighe, remarked in a dissenting view that “the language used [in the interim report] could create the impression that the outlook for humanity is bleak no matter what steps are taken and that consequently, the report's impact on policymakers could be undermined".
Stay tuned!
Read more: International Scientific Report on the Safety of Advanced AI
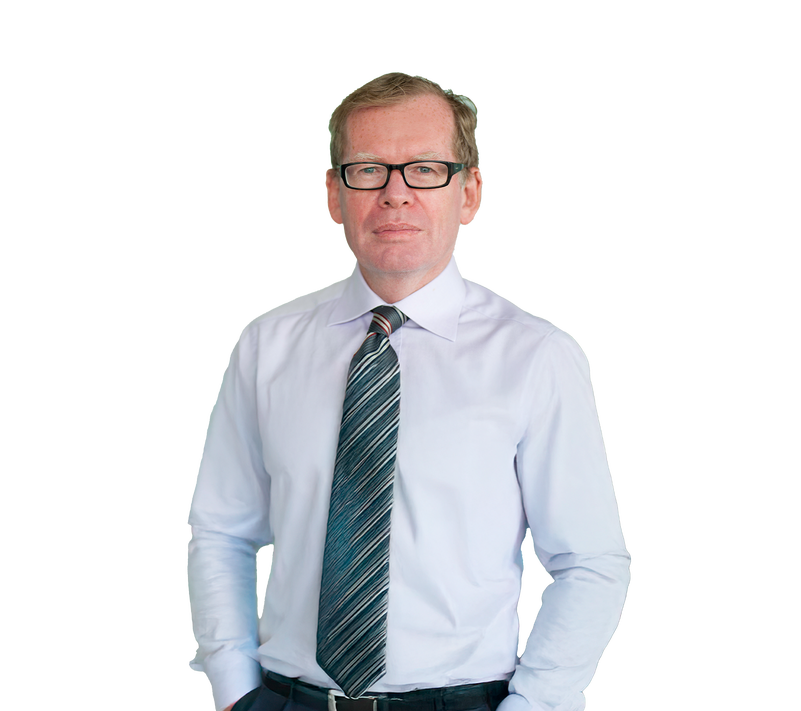
Peter Waters
Consultant