As directed by the National Artificial Intelligence Initiative Act 2020, the US Government’s National Institute of Standards and Technology (NIST) has released an Artificial Intelligence Risk Management Framework (AI RMF). The AI RMF is “intended to be voluntary, rights-preserving, non-sector-specific, and use-case agnostic, providing flexibility to organizations of all sizes and in all sectors and throughout society to implement the approaches in the Framework.” While quite a mouthful, the AI RMF moves beyond other high level guidance to provide a more focused, practical set of recommendations on how to keep your AI ‘on the leash’.
Why is risk management in AI so different to managing other IT risks?
The AI RMF cautions that ‘[w]hile there are myriad standards and best practices to help organizations mitigate the risks of traditional software or information-based systems, the risks posed by AI systems are in many ways unique.’ Traditional software and IT systems are essentially computational tools whereas AI is an ‘inference machine’ trained for decision making, with or without a human.
Compared to traditional software, AI-specific risks that are new or increased include the following:
AI depends on data for training. While use of pre-trained models can improve performance, training data also can increase levels of statistical uncertainty and cause issues with bias management, scientific validity, and reproducibility.
AI continues to learn beyond its original programming. AI systems may become ‘detached from their original and intended context or may become stale or outdated relative to deployment context’.
AI carries substantially elevated privacy risk due to enhanced data aggregation capability of AI - made all the more difficult because ‘big data’ analysis can enable highly targeted decision making by AI without needing to rely on personally identifying information falling within the scope of traditional privacy laws.
There is broad agreement that the characteristics of trustworthy AI systems include: valid and reliable, safe, secure and resilient, accountable and transparent, explainable and interpretable, privacy-enhanced, and fair without harmful bias. But trade-offs are usually involved: e.g. under certain conditions such as data sparsity, privacy-enhancing techniques can result in a loss in accuracy, affecting the commercial efficacy of the AI or even other important values, such as fairness between users.
The sheer scale and complexity of AI (many systems contain billions or even trillions of decision points). In particular, there is an increased opacity of AI systems (‘how or why is it doing that?’) and a higher degree of difficulty in predicting failure modes for emergent properties of large-scale pre-trained models (who would have thought it would do that?).
The AI RMF succinctly captures the difference between AI and all the technologies which have come before:
AI systems are inherently socio-technical in nature, meaning they are influenced by societal dynamics and human behavior. AI risks - and benefits - can emerge from the interplay of technical aspects combined with societal factors related to how a system is used, its interactions with other AI systems, who operates it, and the social context in which it is deployed.
Given this socio-technical character of AI, there are two underlying themes that run through the AI RMF. First, procurement of an AI system cannot be left to an organisation’s standard IT procurement processes nor can ongoing risk management once the AI is introduced into the organisation be left to the Chief Technology Officer and/or its General Counsel. Senior management and the board need to be closely engaged in developing and monitoring an AI risk management framework to ensure that AI executes, and continues to execute, on the values and principles of the organisation. They, in turn, will benefit from drawing on a diversity of views, skills, inputs and demographic backgrounds from across the organisation and often from outside the organisation. We have all learnt the mantra that AI must be trustworthy, and as the AI RMF emphasises, “ultimately, trustworthiness is a social concept that ranges across a spectrum and is only as strong as its weakest characteristics.”
Second, as important as it is to focus on the machine (the algorithms and the data inputs) in an AI risk management framework, don’t forget to worry about the roles the humans will have in the AI lifecycle:
Human Factors tasks and activities are found throughout the dimensions of the AI lifecycle. They include human-centered design practices and methodologies, promoting the active involvement of end users and other interested parties and relevant AI actors, incorporating context-specific norms and values in system design, evaluating and adapting end user experiences, and broad integration of humans and human dynamics in all phases of the AI lifecycle. Human factors professionals provide multidisciplinary skills and perspectives to understand context of use, inform interdisciplinary and demographic diversity, engage in consultative processes, design and evaluate user experience, perform human-centered evaluation and testing, and inform impact assessments.
How does the AI RMF work?
As depicted in the diagram below, the “Core” of the AI RMF describes four specific functions to help organizations address the risks of AI systems in practice.
Many of the task categories in each Core function will be familiar to anyone who has designed and administered a risk framework, such as clearly documenting roles and responsibilities. However, there are also sharper AI-related tasks that hark back to the two underlying themes discussed above.
GOVERN is a ‘cross-cutting function’ - which in ‘management speak’ means that each of the other three functions need a governance element within them, and accountability and direction ‘tracks’ back to the central governance function. This function is designed to connect “technical aspects of AI system design and development to organizational values and principles”.
The key ‘pointedly AI’ tasks in the GOVERN function are:
Executive leadership of the organization must take responsibility for decisions about risks associated with AI system development and deployment - this requires a detailed, inquisitorial and continuing role by the board and senior management. The board and executives will need AI-specific training to ensure they are up to these responsibilities.
Organisations need to develop their own risk assessment of AI in the context of their business. Different participants across the AI lifecycle can have different risk perspectives: e.g. risk metrics used by the organization developing the AI system may not align with the risk metrics or methodologies uses by the organization deploying or operating the system. Also, the organization developing the AI system may not be transparent about the risk metrics or methodologies it used. This is a particular issue with the new foundational AI models which are intended to be useable across many different sectors of the economy, which will have very different risk profiles.
At the operational level, decision-making related to mapping, measuring, and managing AI risks throughout the lifecycle must be informed by a diverse team: e.g., diversity of demographics, disciplines, experience, expertise, and backgrounds.
In turn, organizational policies and practices should be in place to collect, consider, prioritize, and integrate feedback from those external to the team that developed or deployed the AI system regarding the potential individual and societal impacts related to AI risks.
The MAP function establishes the context to frame risks related to an AI system. This is more complex than toting up a fixed-in-time laundry list of risks, instead looking more like a constantly revolving Rubik’s cube, for four reasons: (a) AI participants in charge of one part of the process often do not have full visibility or control over other parts; (b) AI participants have different risk appetites, which can be especially problematic when an organisation is acquiring AI from outside; and (c) AI is a ‘moving feast’ in the operational environment and (d) the interdependencies between these activities, and among the relevant AI participants, can make it difficult to reliably anticipate impacts of AI systems. As a result, the best intentions within one dimension of the AI lifecycle can be undermined via interactions with decisions and conditions in other, later activities.
The key ‘pointedly AI’ tasks in the MAP function are:
The organization’s mission and relevant goals for AI technology must be understood and documented - and as importantly, the risk tolerances against the mission and goals need to be mapped because of the material risk of the AI doing something that will be unanticipated;
Information about the AI system’s knowledge limit, and of current AI generally, must be clearly understood. While generative AI’s abilities are extraordinary, AI is still not good at understanding ‘context’. As the AI RMF says:
Many of the data-driven approaches that AI systems rely on attempt to convert or represent individual and social observational and decision-making practices into measurable quantities. Representing complex human phenomena with mathematical models can come at the cost of removing necessary context. This loss of context may in turn make it difficult to understand individual and societal impacts that are key to AI risk management efforts.
Or as Professor Yann LeCun puts it more colourfully, AI has less common sense than a domestic cat .
Processes for human oversight must be defined, assessed, and documented. Mapping the AI limits helps delineate where the humans need to step back in and exercise good old fashioned human judgment and discretion. But the AI RMF also cautions that having ‘humans in the loop’ of decision making (e.g. AI provides a recommendation to a final human decision maker) is not necessarily the easy answer it seems: “the AI part of the human-AI interaction can amplify human biases, leading to more biased decisions than the AI or human alone.”
Again, the MAP function is not a one-off exercise but has to continue to ‘shadow’ the AI. In particular, organisations using AI should expect that the boundaries between automated decision making and humans “softening the edges” of that automated decision making will move over time as the MAP function identifies unanticipated problems or conversely, strengths of the AI.
The MEASURE function “employs quantitative, qualitative, or mixed-method tools, techniques, and methodologies to analyse, assess, benchmark, and monitor AI risk and related impacts.”
The key ‘pointedly AI’ tasks in the MEASURE function are:
The AI system is evaluated regularly for safety risks. Safety metrics reflect system reliability and robustness, real-time monitoring, and response times for AI system failures and whether the AI continuous learning has resulted in 'drift' from its original purpose and the organisations principles.
Measurable performance improvements or declines based on consultations with relevant AI participants and users, including affected communities, and field data about context, relevant risks and trustworthiness characteristics are identified and documented.
The problem with the MEASURE function, as the AI RMF candidly acknowledges, is that there is a current lack of robust and verifiable measurement methods for risk and trustworthiness. As a result, there can be an inability to document AI-based practices to the standard expected of traditionally engineered software for all but the simplest of cases.
Also, MEASURE is a tool, not a substitute for AI trustworthiness:
Transparency is often necessary for actionable redress related to AI system outputs that are incorrect or otherwise lead to negative impacts... A transparent system is not necessarily an accurate, privacy-enhanced, secure, or fair system. However, it is difficult to determine whether an opaque system possesses such characteristics, and to do so over time as complex systems evolve.
Lastly, the MANAGE function entails allocating risk resources to risks MAPPED and MEASURED on a regular basis and as defined by the GOVERN function.
The key ‘pointedly AI’ tasks in the MANAGE function are:
Procedures to be followed to respond to and recover from a previously unknown risk when it is identified.
Mechanisms to be in place and applied to supersede, disengage, or deactivate AI systems that demonstrate performance or outcomes inconsistent with intended use - including what are the fallback non-AI systems.
Measurable activities required for continual improvements to be integrated into AI system updates and include regular engagement with interested parties, including internal and external users.
Availability of a clear, easy to use appeal process (to humans) when users disagree with automated decision making - some laws go further and require users have an easy opt out from fully automated decision making.
AI RMFv1
NIST plans that the AI RMF will continue to evolve and deepen with feedback from organisations using it to build and benchmark their AI risk frameworks. NIST has produced an accompanying Play Book as a practical implementation tool with a (quaintly amateur) video explainer .
Read more: Artificial Intelligence Risk Management Framework (AI RMF 1.0)
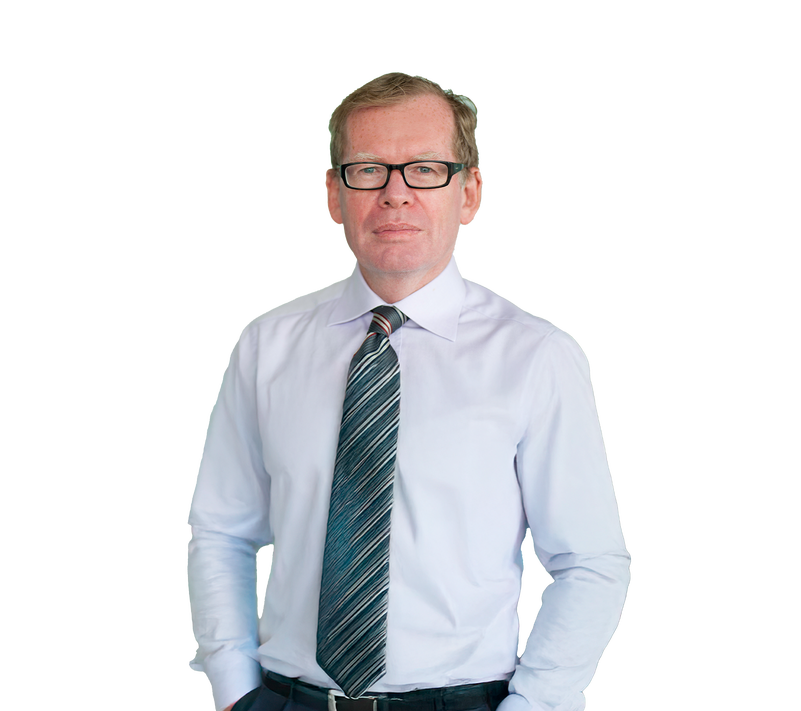
Peter Waters
Consultant