Last week we looked at the European Union’s’ Agency for Fundamental Rights (FRA) evidence-based study into bias in algorithms detecting offensive speech. This week we review the FRA study’s analysis of the risks of feedback loops in machine learning, with predictive policing as the case study.
Predictive policing is already on the ground
Predictive policing involves the application of statistical techniques – particularly quantitative techniques – to predict likely targets for police intervention and to prevent crime or solve past crimes. The use of machine learning can detect statistical relationships that the 'typical coppa' armed with a spreadsheet might not identify.
Police forces around the world are already deploying predictive policing platforms. For example, Precobs, which is deployed in Austria, Germany, and Switzerland, assesses the likelihood that certain areas will experience burglaries (so called ‘place-based predictive policing’) based on the theory of near-repeat phenomena, which identifies burglaries that are likely to be followed by crimes in the vicinity. It uses geographical data, combined with police statistics on burglary locations, time of occurrence, items stolen and modus operandi, to deduce patterns corresponding to professional serial burglars and predicts likely near-repeat burglaries.
More controversially, other predictive policing programs can attempt to identify potential criminality based on personal circumstances or other identifying criteria of individuals or groups (so-called ‘person-based predictive policing’). In 2019, the Netherlands introduced SyRI, a system designed to help the government identify individuals at risk of engaging in fraud in the areas of social security, tax and labour law. The Dutch Government withdrew the system after a court found that it violated the right to privacy under the European Convention on Human Rights. Philip Alston, United Nations Special Rapporteur on extreme poverty and human rights, in a letter lodged with the court said:
“Whole neighborhoods are deemed suspect and are made subject to special scrutiny, which is the digital equivalent of fraud inspectors knocking on every door in a certain area and looking at every person’s records in an attempt to identify cases of fraud, while no such scrutiny is applied to those living in better off areas.”
AI is beginning to be used in Australian police forces. The New South Wales Police Force already uses AI to analyse CCTV Data. Recently Queensland trialed an AI tool to help police identify high risk domestic violence offenders.
Introduction to Feedback loops
The great benefit of AI is that it ‘learns on the job’. The AI produces a prediction based initially on its training data, decisions are made and the observed results are added to the training data for the next round of training.
But therein also lies the risk. If a bias is embedded as an inherent part of the initial input data, this can be exaggerated when decisions made by that system are used as inputs that determine future decisions. This creates a feedback loop. These loops can be difficult to identify in systems characterized by a lack of transparency and accountability.
This risk in predictive policing AI is fairly simply explained. Predictive policing algorithms like Precobs use crime and other police data statistics to determine the frequency of police patrols in these locations. This means that more police resources are dedicated to geographic areas that reportedly experience higher volume of incidents. This increased level of patrols inevitably leads to an increase in crime identified. This new information then forms part of new crime statistic feed into the AIs, which are used as the AI inputs, causing the system to over-emphasize the risk of crime in those areas even further.
While these risks of predictive policing have been recognized before, the FRA study seeks to quantify just how pronounced the feedback loop can be. The study simulated two neighbourhoods in which the true crime rate was the same, but the initial allocation of police happened to be 20% in one neighbourhood and 80% in the other. The study compared how the allocation of police resources would play out over time using a simple probabilistic model and an AI model. As illustrated in the diagram below, despite the true distribution of crime being uniform, the initial historical bias was maintained under the probabilistic model. By contrast, the AI predictions with the same parameters gradually contributed to the generation of a feedback loop that assigned, after 40 weeks, 100% of the police resources to district 2.
The FRA concluded that machine learning can amplify small biases in the test data, creating a ‘runaway feedback loop’.
The FRA study highlights how this becomes particularly problematic when areas over-emphasized by predictive policing algorithms are home to ethnic minorities. Due to the perceived objectivity of AI data, there is a danger that these models will be used to justify other discriminatory over-policing policies. This outcome is, in a sense, an AI version of the ‘broken windows’ theory of policing introduced in New York City and other large US cities which focused on minor crime in an effort to reduce the general climate of social disorder in which major crime could occur – but many considered that this approach became self-perpetuating and focused policing on historically disadvantaged groups, such as African Americans youth.
This is more than about the risks of the existing bias of human cops making its way into AI ‘on the beat’. Bias can make its way into predictive policing AI from the public through crime reporting. The rate of crime reporting (e.g., dial a Crimestoppers service) also can drive feedback loops in allocation of police resources, largely divorced from the levels of actual crime. When the difference in crime reporting rates between neighbourhoods is large (more than doubled) and the true crime distribution is closer to uniform, the neighbourhood with lower ‘true crime’ ends up with the largest portion of police patrols.
A study by the Georgetown University Poverty Center found showed grownups in general perceived Black girls as not as innocent and less deserving of protection than white girls. Yale University recently recognized a 9-year-old Black girl whose neighbour called the police reporting suspicious activities as she worked to eradicate invasive insects from her hometown.
This could be called the ‘nosey-parker’ or ‘twitching blinds’ effect.
Mitigation techniques for Feedback loops
The FRA study acknowledged that ‘policing is much more complex and police consider many more aspects before sending patrols into certain neighbourhoods...Police work with people and are present on the streets, which is why a purely computer-based simple simulation cannot reflect reality and all its complexities.’ However, the study thought that the risks of feedback loops illustrated by its simulations required safeguards to be implemented to ensure human rights were not transgressed by predictive policing algorithms.
The FRA study suggests a threefold approach:
-
Increasing the amount of objective data used as initial inputs. The FRA study says this is key to building community trust in the fairness of predictive policing AI, but it also acknowledges there is some circularity here: FRA research showed that the lack of trust in the police is one reason for people not reporting burglary in the first place, either because of a general lack of trust (7 %) or because they expect the police would not do anything about it (25 %).
-
Using technical solutions that address machine learning's tendency to focus too much on extreme patterns in training data (a pattern called “overfitting”). A countervailing practice called “regulation” involves applying a mathematical restriction on the algorithm to screen out more extreme predictions. However, the FRA study acknowledges this itself is a tricky exercise: its value should be scrutinized regularly to ensure feedback loops are prevented but also ensuring the produced predictions remain useable.
- Using 'down sampling': downsampling involves randomly removing observations from a class of data (usually the majority class) to prevent its signal from dominating the learning algorithm. Downsampling can be used to counteract the low reportability of specific crimes. Real world situations will be more accurately reflected if widely accepted qualitative factors are used to complement quantitative data inputs.
Conclusion
We now know that AI tools are far from neutral, and not necessarily less discriminatory. But as the FRA study reminds us, the goal of an entirely ‘neutral' AI is unrealistic. Algorithms are developed and used by humans and where bias is present in human decision-making, it will be transferred to algorithms. Therefore, if a business or organisation develops or uses AI, actively detecting and mitigating bias has to be ‘standard procedure’.
While bias may lead to discrimination, it is also necessary for the proper functioning of machine learning systems. The development of ‘neutral’ training data with respect to certain characteristics, such as gender and ethnic origin, raises the question of the extent to which such predictions should actually be neutral: if you don’t teach an algorithm what bias looks like, it will miss the bias altogether or not recognise it for what it is.
Additionally, bias in an AI machine learning system might be necessary for the purposes of positive discrimination. For example, it may increase the allocation of opportunities to historically underrepresented groups. What is important to consider, when determining whether mitigating techniques identified in the EUFRA’s are necessary are the potential outcomes of such algorithmic bias.
The FRA study rounds off with a ‘mandate’ for digital transformation:
“It is high time to dispel the myth that human rights block us from going forward. More human rights mean more trustworthy technology. More trustworthy technology is a more attractive technology. In the long run it will also be the more successful technology.”
Read more: Bias in algorithms - Artificial intelligence and discrimination
Authors: Clare Veal, Monty Raper, Peter Waters
KNOWLEDGE ARTICLES YOU MAY BE INTERESTED IN:
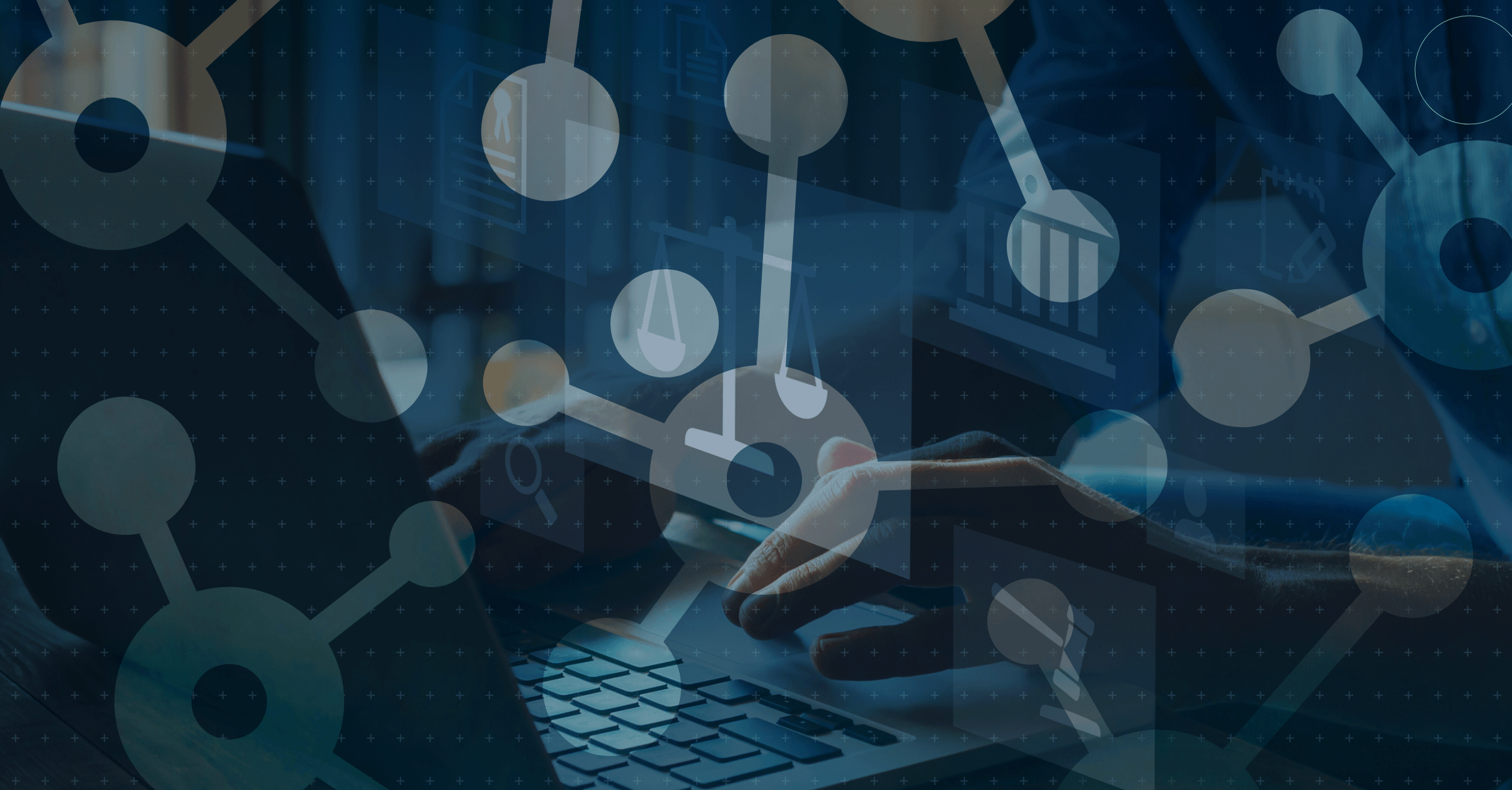
Visit Smart Counsel