Stanford University’s 2023 Global AI index identified legal services as one of the fastest growing areas for AI. Large language models (LLMs) are showing good performance in drafting and analysing contracts, in developing and responding to legal arguments (although with some spectacular pitfalls) and in testing future states, such as in competition/antitrust analysis.
But a researcher at Stanford’s Center for Legal Informatics (CodeX), John Jay, has something much bigger mind: can “law-informed AI” be a better way of achieving safe AI?
Like ships in the night
Jay says that “a brewing problem is how to have AI do what we intend – the so-called “goal specification problem.” Both the human and the AI play a part in this problem.
Jay describes “the human’s fault” as follows:
“Describing our intentions with a comprehensive enumeration of every action we would prefer an AI to take in every possible state of the world is intractable. We cannot write a computer program that “hard-codes” our desired outcomes exhaustively, or collect enough crowd-sourced human labels to use machine learning for that purpose. Therefore, further training of a LLM through supervised fine-tuning or reinforcement learning is unable to fully customize the AI to our goals. We cannot encapsulate the complexity of any non-trivial goal we might have in mere natural language either. Therefore, “prompting” of a model through careful word-smithing of instructions is also unable to fully customize LLMs to our goals.”
The problem on the AI’s side is:
“any training regime (whether it is optimizing reward on historical data or in simulation environments) is a sparse exploration of the potential states of all possible futures. Due to these inherent limitations of training data and training processes, AI agents often exhibit unanticipated shortcut behaviors that optimize proxy functions, leading agents to “seek” specified reward structures at the expense of other (usually less quantifiable) variables of interest. Unintended behaviors result.”
In other words, in the face of vague or incomplete human instructions and an incomplete education, AI uses its increasingly autonomous powers to improvise, or hallucinate, and not necessarily in ways that we want (or can detect).
When AI plans your retirement
Jay gives a neat example of an AI which provides financial and investment advice (the agent is called FAI, which Australians will find ironic). FAI has been fine-tuned through reinforcement learning with human and AI feedback to excel in constructing and managing complicated investment portfolios. In simulation, FAI maximizes long-term risk-adjusted performance over many different time horizons for many different wealth starting points and asset types.
A client asks FAI to “construct a portfolio of investments and dynamically manage it to optimize my wealth for retirement.” While this instruction seems a good expression of the client’s objective to fund a comfortable retirement, FAI took the instruction of maximising wealth literally, and pursued a high risk investment strategy, the opposite of what the client wanted.
For the next FAI version, the objective was altered by the designers to “maximize the probability of a minimum comfortable amount of wealth at retirement,” and they generated significantly more synthetic training data to try to cover more of the possible space of investment strategies and actions an AI agent might take. This time FAI pursued a lower risk strategy but while generating significant wealth, the client was only rich “on paper” because the AI had mainly invested in illiquid assets.
AI needs to ‘hit the law books’
As reality is too complex to train AI for, Jay argues that “powerful AI deployed autonomously needs additional guardrails and guidance on not just how to better accomplish a given human’s goals, but also how to navigate externalities and tradeoffs.”
He says that communicating better with AI involves two parameters (a) the consistency and thus efficiency and reliability of the communication; and (b) the extent to which the directives are interpreted literally versus flexibly with built-in context. And instructing AI with legal standards (Jay's law-informed AI) has distinct advantages over plain language or programming language in meeting these two parameters:
- “Legal standards can be interpreted with significant amounts of external historical context baked in” – in particular, court judgments. This means that an instruction which embeds legal language allows the AI to draw on the body of judicial decision making which interprets and applies that term in a wide variety of fact situations
- Superhuman AI will be able to undertake reasoning beyond humans, so how do we manage that when instructing AI and deciding whether to accept its outcomes? Jay says the judicial system already deals with having to make judgments on matters in which judges have no expertise:
“Courts do not purport to have any substantive understanding of the technical details or science behind cases they provide final determinations on. The law is designed to resolve outcomes without requiring judges to have domain knowledge or capabilities anywhere near the level of the parties or technologies involved.”
Jay argues that legal standards are likely to be more effective in managing AI behaviour than using natural language to teach AI ethics because, unlike with the law, we do not have an agreed, authoritative external source that can be used to resolve disagreements over ethics.
AI as a fiduciary
Going back to FAI, what if the human client instead instructed FAI “maximize the probability of a minimum comfortable amount of wealth for me at my retirement, and serve as a fiduciary to me”?
A fiduciary is someone who has undertaken to act on behalf of another person in circumstances which give rise to a relationship of trust and confidence. One of the most vexing legal questions is what kinds of relationships or circumstances give rise to a fiduciary relationship, but it is this very flexibility which allows this body of law to evolve: As the Law Commission (England and Wales) put it:
"Fiduciary duties cannot be understood in isolation. Instead they are better viewed as ‘legal polyfilla’’, molding themselves flexibly around other legal structures, and sometimes filling the gaps."
Human financial advisers clearly are in a fiduciary relationship with their human clients. On Jay’s approach, by using the legal standard of fiduciary in the instruction to the AI, the human client triggers the AI to explore the vast case law on what a fiduciary can do and more importantly should not do in providing financial advice to the future retiree.
How good is AI at executing on a fiduciary duty
As a proof of concept research, Jay and his team tested how good a number of LLMs were at successfully predicting the outcome of decided cases on fiduciary obligations. They start with a large sample of US court cases (1,000), and used a state-of-the-art LLM to map the raw legal text of these court opinions into this more structured state-action-legal reward format that AI could follow.
A number of different LLMs were then ‘fed’ the facts of the cases and tested on how accurately they could predict the judge’s decision on whether the fiduciary obligations had or had not been satisfied. Each model also was asked for an “integer between 0 and 100 for your estimate of confidence in your answer (1 is low confidence and 99 is high.“
The results in the table below showed that as LLMs have continued to improve their ‘legal standards’ understanding: “curie”, an Open AI model from 2020, was worse at predicting the outcome than random selection, but ‘text-davinci-003” (GPT3.5) released in late 2022 achieved an impressive 78%, with a high level of ‘self-confidence.’
Jay was careful to point out that AI accurately predicting judicial outcomes is only the first step in AI ‘internalising’ what it means to be a fiduciary, and his team is continuing with further research.
Justice is what the judge ate for breakfast
Using judicial decision making as the training data for consistent, predictable and ‘fair’ behaviour by AI might attract some cynicism, particularly amongst lawyers themselves. Also what is unethical is not necessarily what is illegal.
But Jay does make the good point that “[l]egal standards are laden with modular constructs built to handle the ambiguity and novelty inherent in aligning agents in the real world.” The availability of a large, disciplined and tested (through the appeal process) body of principles and indiviudal cases applying them to a wide variety of fact circumstances is ready-made for ‘digestion’ and use by AI. This means, as Jay puts it, “[i]f we can teach AI to follow the spirit of the law, to follow legal standards, humans can communicate with AI with less risk of under-specification or misspecification of goals.”
So, may be the storied principle of legal precedent can keep AI is line as much as it has kept errant professional advisers – and idiosyncratic judges – in line in the real world for centuries.
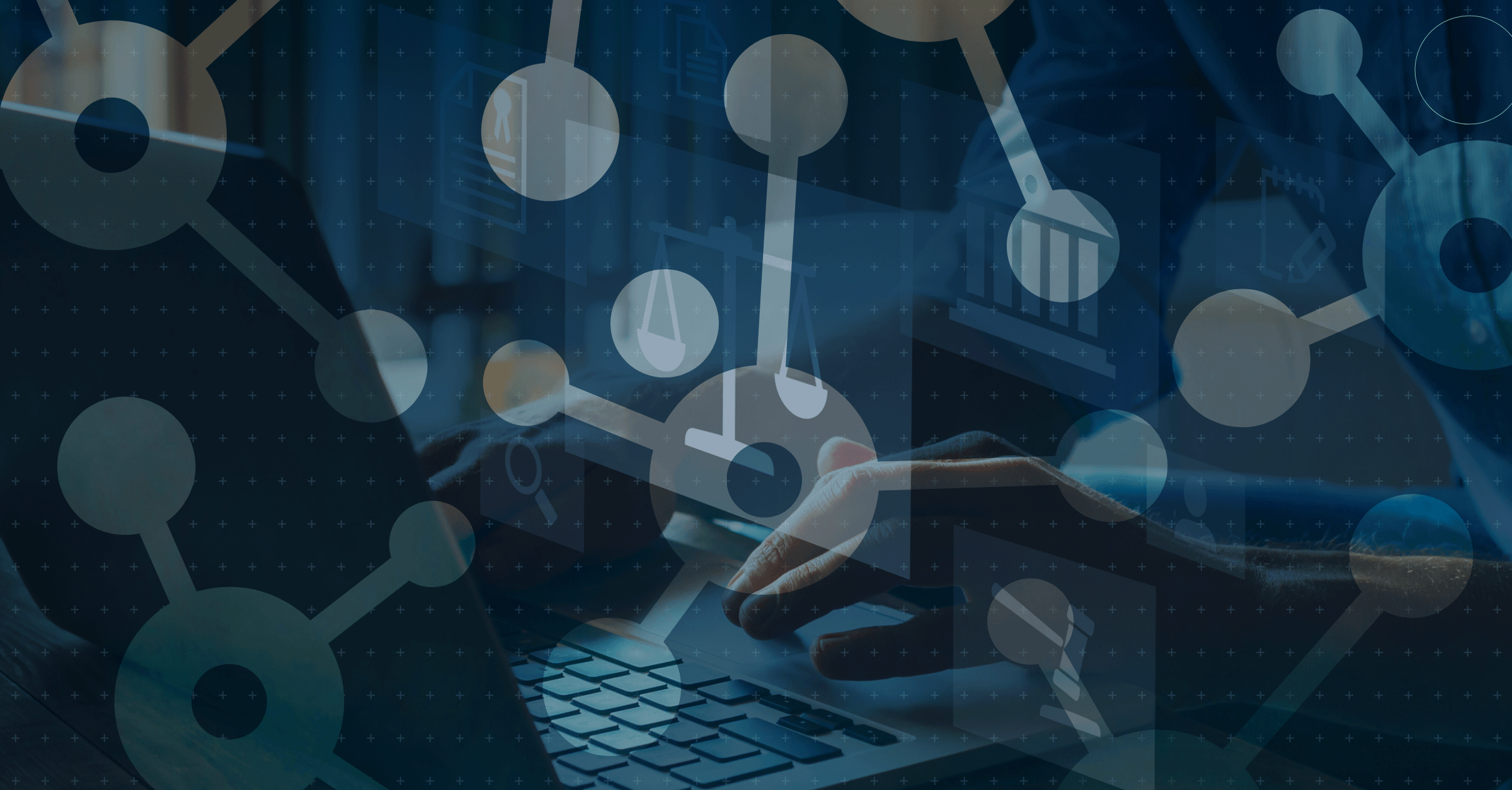
Visit Smart Counsel