By 2024, it is predicted that 60% of data used to train artificial intelligence systems globally will be synthetic: i.e. artificially-generated data created using generative AI.
In a recent article, two legal academics, Michal S Gal and Orla Lynskey, predict that “synthetic data has the potential to do to data what synthetic threads did to cotton.”
How is synthetic data made?
Gal/Lynskey identify three categories of synthetic data based on how far removed the data is from real world data (which they call ‘collected data’):
synthetic data can be generated based on a transformation of collected data. Synthetic data can be used to fill gaps in the collected data; or conversely, synthetic data can strip out personal or group characteristics to reduce bias effects. Synthetic data can even take the statistical characteristics of the original dataset and creating a wholly synthetic one with quite similar characteristics, a thorough ‘deidentification’ approach to break the link back to individuals.
synthetic data can reduce the need for collected data. One methodology involves two neural networks pitted against each other in a zero-sum game. The first network, the Generator, generates synthetic data without directly using the collected data. The generated data is then sent to the second neural network - the Discriminator - which was trained on collected data. The Discriminator compares the synthetic data with the collected data, creating a propensity score and determining which parts of the data give away its “fakeness”. The process keeps repeating until the Generator is successful in creating a synthetic dataset which the Discriminator accepts is real. For example, where data in a HR AI is too male-dominated, this approach could be used to generate equally compelling data about fictional female candidates (called upsampling).
a simulator generates an entire set of synthetic data based on a set of rules which determine the relationships between the relevant data attributes. For example, the AI simulator DeepMind was taught to play the board game Go by first being fed the rules of the game and then trained by creating numerous simulations of games playing against other instances of itself. Not only did the AI replicate a complete dataset of games with no collected data, but it developed new strategies never seen among human players.
The benefits of synthetic data
According to a recent OpenAI report, the cost of training large AI models will rise from $100 million to $500 million by 2030.
Much of the cost of assembling large training data sets is in the process of cleaning and preparing the data, including labelling the data which is often done manually. Synthetic data essentially combines the process of data collecting, labelling and organising the data automatically during the generation process, creating data that is fit for purpose from the start. Gal/Lynskey quote one entrepreneur estimating that “a single image that could cost $6 from a labelling service can be artificially generated for six cents”.
But synthetic data has other important advantages over real world data. AI can learn faster on synthetic data, for example:
the AI developer Nvidia uses synthetic data to train robots in warehouses to recognise objects of different shapes and sizes in different conditions. Synthetic images can be generated covering a huge variety of shapes of boxes under a myriad of light/shadow conditions which might only rarely occur in a collected dataset.
In medical research, “digital twins” replicate a real medical profile of an individual. Synthetic data can go even further and generate a virtual patient which combines a wider range of human conditions. Gal/Lynskey quote a medical researcher:
we should not limit ourselves by how the real world limits us. We can’t create a person that represents more than that person, but we can create a model that represents more than one person. Why not take advantage of that? Once you understand the diversity [of patients], you can build that into the [future, virtual] patient population.
Should synthetic data upend antitrust assumptions about Big Tech?
Control of vast lakes of collected data is consistently identified by antitrust/competition law agencies as the cornerstone of the market power of Big Tech. As the OECD has observed:
[D]ata can give rise to self-perpetuating feedback loops, network effects and economies of scale that enhance the first-mover advantage of incumbent firms. Further, data access can be leveraged across multiple markets Evidence suggests that market power may be on the rise, and that it may more durable, particularly in digital-intensive sectors.
Gal/Lynskey argue that synthetic data can help change such market dynamics. Given that synthetic data can be used to augment collected datasets which are otherwise too small to be useful, firms with small datasets could compete with firms that possess much more collected data. In turn, if collected data no longer confers a significant comparative advantage on Big Tech, there will be greater incentives to share data, with the price capped at the (relatively low) cost of generating synthetic data.
Therefore, Gal/Lynskey think that the growing prevalence of synthetic data should lead to a less interventionist approach by antitrust/competition agencies:
Mergers : “in industries where firms will be able to compete with smaller quantities of collected data, and where the collection of such data does not involve insurmountable barriers, more mergers would be benign.”
Mandatory data access : many have argued, applying the philosophy of the Essential Facilities Doctrine, that some types of data should be recognised as “essential data” and subject to an access regime. For example, the EU Digital Markets Act mandates the gatekeeper platform to provide competing providers of online search engines with access on fair, reasonable, and non-discriminatory terms to ranking, query, click, and view data generated by searches on its engines, subject to anonymisation of personal data. Gal/Lynskey argue “data may challenge such essentiality in some markets: if collected data can be replaced by synthetic data, then the justifications for requiring firms to share it are weakened.”
Gal/Lynskey also consider whether synthetic data might cut the other way and suggest the need for a tightening in antitrust/competition laws in some scenarios:
“To illustrate, consider the prohibition of cartels, which is based on the existence of an “agreement in restraint of trade”. Synthetic data on market conditions and rival's actions can help train algorithms to reach a coordinated equilibrium without such an agreement, and the algorithm can then be applied to real-world conditions. In other words, synthetic data can be used to circumvent the existing law, in a way that can only be addressed by reformulating the content of the prohibition .”
In mergers, they write that synthetic data could exacerbate the competitive harms of some mergers. ObamaCare prohibits health insurance companies discriminating against clients based on pre-existing conditions. A way to circumvent that prohibition would be to strike insurance rates on a geographically de-averaged basis where consumers are more prone to suffer from certain medical conditions. Privacy laws would prevent use of patient records for that purpose, but it might be possible to generate synthetic deidentified data. Gal/Lynskey note that this is a potential risk of the recent merger between a major US health insurer and the largest electronic data interchange clearing house.
Should privacy law protect you against adverse inferences based on other people’s data?
Use of synthetic data is clearly privacy enhancing. Gal/Lynskey acknowledge that the risk of reidentification cannot be ruled out, but this risk is much less than with other approaches to deidentification in which the final data set is still a version of the real world data.
Gal/Lynskey argue the real challenge to basic rights from synthetic data is the making of inferences about an indiviudal based on data synthesised from other people’s information. They give the following illustration: if we know from data on others that eating a high-fat diet increases the risk of heart conditions, and that Ann is part of a community that eats a high-fat diet, we may infer that Ann is at higher risk of heart disease.
Gal/Lynskey note that to the extent that the law has been attentive to inferences to date, this has been primarily to consider whether inferences about a person deduced from their own personal data constitute personal data. They pose the challenge of synthetic data as follows:
if the synthetic generation process is successful, then the dataset generated will constitute a convincing replica of a dataset about real world people. If this replica dataset can be used to impact individuals, then irrespective of the precise data used to draw this inference, the threat to individuals’ fundamental rights will be the same.
They say that this requires a fundamental rethink of what we are seeking to protect against in privacy laws:
This begs the question of whether the concept of identifiability is sufficient to prevent harm to individuals, and whether it can capture linkages or inferences on which synthetic data might be based. This also casts further doubt on the utility of individual control over one’s privacy. Accordingly, lawmakers and courts face a dilemma: to define or interpret the scope of data privacy laws more broadly, thereby loosening the link between collected data and the individual and capturing more data flows under their scope, or to see some of the values that data privacy laws promote undercut by synthetic data processing .
Gal/Lynskey acknowledge that expanding privacy laws in this way could significantly restrain the utility of data, and that a balance needs to be struck. But they leave open where that new balance point may be.
Can information be too accurate?
Data accuracy should be enhanced with synthetic data and that generally should lead to better decision making.
But Gal/Lynskey also note that it cannot be assumed that more accurate data will always enhance welfare:
Overly accurate information can enable new forms of differentiation and categorization, which might have negative welfare effects on individuals and groups through exploitation or manipulation. Accurate data can also give rise to a loss of manoeuver space for individuals. Likewise, it makes individuals and society more readable, potentially reducing individuals’ capacity for self-development and change, while exacerbating power and information asymmetries between those who process data and those who are subject to this data processing .
Gal/Lynskey point out that we also have laws which dilute data accuracy - or rather the granularity of data that can be used. They go back to the example of restrictions on personal data which can be used to strike individual health insurance rates.
They note that existing laws, particularly consumer protection and fair trading laws might, as well as ensuring synthetic data is not wrong and misleading, also set ‘fairness’ bounds on the accuracy of predictive analytics. They also posit that there may need to be additional legal measures, and give the example of the creation of “throttling metrics,” by which friction in the algorithm might protect important human values.
Conclusion
While recognising the great benefits of synthetic data, Gal/Lynskey stress it is no panacea. But more significantly, they go on to argue that “synthetic data challenges the equilibrium found in existing laws” and that we need to consider (urgently as the synthetic data train has already left the station):
a shift in the focus of data governance models from data collection to its uses and effects; from user consent and control to notions of welfare and well-being; and from private data to inference data and to collective data harms .
But the shifts advocated by Gal/Lynskey involve moving away from current legal concepts which, while they have their blurry edges, are tangible. This is than more an inertia of the known. Rebasing privacy law from the concept of personal identifying information to a new balance around social welfare vs social harm involves a much more qualitative assessment: one which, as Gal/Lynskey acknowledge, could produce different outcomes between different synthetic data-fed AI, and the same AI fed with real-world information. Also, as we will discuss next week, the risks of whether and how algorithms might collude are highly nuanced.
Read more: Synthetic Data: Legal Implications of the Data-Generation Revolution
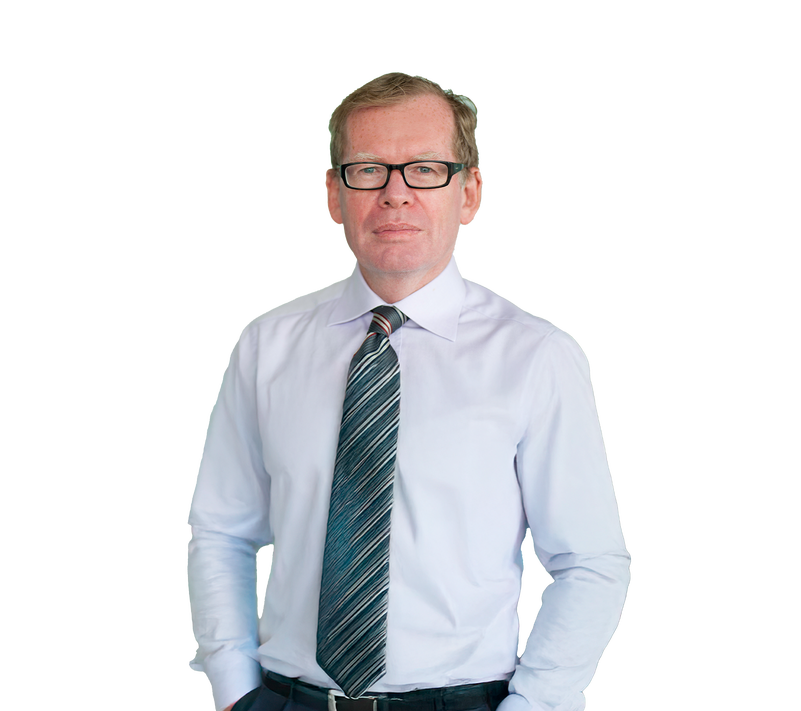
Peter Waters
Consultant