Just as the UK Government publishes its AI governance implementation guide and the Australian Government announces its expert group on ‘safe and responsible AI’ , a paper from Harvard’s Kennedy School argues that the “reigning paradigm” of AI governance is too “reactive, punitive, status-quo-defending” and calls for “an expansive, proactive vision for technology—to advance human flourishing”.
What’s wrong with current AI governance frameworks?
In the policy equivalent of “if I was going to Dublin I would not start here”, the Kennedy School paper argues that many of the emerging global regulatory approaches to AI governance come from an individual harms and rights perspective, except China (as would be expected):
Risk frameworks like the ones in the EU AI Act rely heavily on frameworks developed for governing human subjects research in the biomedical and behavioural sciences. Those frameworks have, historically, focused much more heavily on individual harms and benefits, rather than on harms and benefits to groups, societies, or humanity. The EU, UK, and Japan introduced liberal, rights-protective frameworks, with varying degrees of emphasis on individual rights and societal goals. The U.S. also embraces a liberal, rights-protective framework, but with added attention to marginalized populations and equity, as well as a high priority on national security and economic competitiveness. China explicitly frames its policy around socialist values, giving no attention to the protection of rights.
The result, the paper goes on to argue, is that principles around which AI governance models are framed are not moored to any clear ideology (our word, not theirs):
The goals of protecting privacy, accountability, and transparency are insufficient as guides for the present moment because they do not in themselves include a governance vision. They give us a framework for thinking about how to protect individual rights but little guidance for how society should steer through organizational, systemic, and/or existential risks and opportunities. They support reactive and punitive approaches to governance but no vision for how to construe the risks and opportunities much more broadly, and to make correspondingly large investments in public goods and personnel.
Looking inside the ‘black box’ of AI model itself for ‘the good and the bad’ misses the point. Rather, in defining safe and responsible AI, the paper argues you need to look at the AI model’s impact on the outside world “because AI systems are deployed in use cases that are themselves connected to other social and biological phenomena, the effects of AI systems may emerge that flow not from the specific technical capabilities of the system but rather from how that capability interacts with other phenomena.
The paper also argues that development of effective AI governance frameworks has been paralysed by a false debate over whether short term or long term risks are the more urgent to address:
Two conceptual frames have come to divide and dominate literature on AI governance. On one hand, philosophers, computer scientists, and industry executives sympathetic to long-term predictions and utility mathematics have taken cues from science fiction to spin out possibilities of extreme and even existential risk from AI systemsOn the other hand, social scientists and students of the history and ethics of artificial intelligence often focus on near-term risks and already present harms, identifying problems such as bias and unfairness in algorithmic design or deployment, or potential violations of privacy.Policymakers are then left to balance what are presented as two fundamentally different worldviews: the present harm approach and the future risk approach.
Instead, the paper says that this is “not a fundamental philosophical problem..[but].. an organizational problem — that is, a challenge of proper task delegation and governance design.” The solution is to assign responsibilities for different regulatory tasks to different public sector agencies: the near term harms mostly should be handled by upskilling existing agencies and a new agency or coordinating task force should be established to manage emergent capabilities, including potentially a new international agency - the AI equivalent of the International Atomic Energy Agency.
An alternative set of principles of AI governance
The Kennedy School paper proposes its own set of 4 propositions for AI governance:
Proposition 1: Technology, properly conceived, ought to advance human flourishing. “Society can’t steer the technology that shapes our lives if we don’t first declare its purpose.”
Proposition 2: Human flourishing requires individual autonomy. The paper argues that autonomy is a two-sided coin of ‘negative liberties’ (‘freedom from..’) and ‘positive liberties’ (‘freedom to..’): current AI governance frameworks focus on the negative liberties, “where we are protected in our person, our property, our conscience, our expression, and our associations”, but because they are confided by their individual rights approach, they fail to adequately address ‘positive liberties’, where “we govern ourselves in our private lives and share in the governance of our public lives”.
Proposition 3: Autonomy requires the values of democratic governance. While requiring steps to safeguard democracy (e.g. acting on misinformation), the paper argues that AI governance “needs to go beyond the surface features of democracy (elections, checks and balances, etc.) to get at the conditions that allow for autonomy and therefore are essential for human flourishing.”\
Proposition 4: Autonomy requires the material bases of empowerment. This goes to emerging concerns that AI will deepen the digital divide and disadvantage gaps. The paper urges “[w]e need technology thatexpands human capacities rather than supplanting the place of human beings in the productive structure of the economy”, and is very deliberately steered towards social connectedness, trust and human physical and mental well-being.
Is this just all arm waving?
The Kennedy School paper seeks to flesh out how these 4 AI propositions should be applied in a more tangible agenda for government agencies and private enterprise:
Blocking and mitigating harms from both the production and use of AI tools
The paper identifies the following three harms that need to be addressed:
harms to individual and community flourishing: this includes the societal impact of rapid economic transformation; the societal impact of potentially shrunken trust and verifiability; and the digital divide.
harms to democratic/political stability: this includes proliferation of fraud and impacts on the administration of justice and democratic processes; expanding power differentials between the public and technology executives and investors; and overreliance on AI tools as arbiters of truth and originators of sound decision-making.
harms to economic empowerment: this includes labour dislocation; exploitative practices related to mining and data-scraping harming local economies and violating human rights abroad; uncertainty about the future of independent and creative art and cultural products as technologies generate content recombining the work of past artists; and exploitative labour conditions in mines for metals valued for use in AI and new technologies.
Addressing these harms will require some bureaucratic replumbing:
Because..standards for evaluation, audit, and security will need to be put in use across multiple domains and sectors, already subject to existing regulatory bodies, governments will want to form cross-agency learning teams to try to steer toward alignments of conceptualization and vocabulary across context. Such cross-agency learning will in all probability be best advanced by a free-standing AI regulatory body, charged with integrating AI regulation within the procedures of all existing agencies.
Seeing and mastering emergent capabilities (i.e. the sci-fi scenario)
The paper describes the challenge across AI models as follows:
While closed-source models try to get ahead of..use cases [of potential catastrophic or existential risks] by creating boundaries for off-limit topics and capability testing through red-teaming, they have not completely succeeded. The rise in powerful open-source models, and whether public access trumps increased accessibility for bad actors, has been heavily debated. Open-source models make the source code of these AI systems accessible and available to anyone on the internet, therefore bypassing the requirements or accountability of companies who might be held responsible.
Hence the need for a standalone agency (and possibly the ‘AI IAEA’) to provide oversight of frontier labs with gating requirements for new AI models, including red teaming for emergent capabilities to identify and address emergence.
Steering toward public goods, including funding R&D
The paper says “we need to see the opportunities new technologies offer to solve collective action problems, and provide public investment for research into and development of those solutions” by focusing on the flip-side of the three harms outlined above:
benefits to individual and community flourishing: this would involve investment in personalization of learning; improved access to expert advice and internet literacy; and contextualization engines to help protect against fraud, misinformation, and disinformation.
benefits to democratic/political stability: this would involve investment to increase opportunities to engage in democratic process, including cross-jurisdictional possibilities.
benefits to innovation, creation and economic integration: this would involve investment to promote entrepreneurial opportunities; and creating new jobs; and facilitating. “task diversity”—one person can complete many more different kinds of tasks than they could before.
Human Capital Strategy
The paper makes the obvious but important point that none of the above will work unless governments urgently upskill agencies across their bureaucracy. But the paper acknowledges the challenge the public service faces in attracting AI talent from the private sector and suggests, perhaps a little wistfully, that “[t]here is an urgent need for colleges and universities with high levels of graduates in technical fields to build an expectation for graduates of national service at some point over the career life course.”
Investing in the sustainability of democratic steering capacity
While the paper recognises the innovative role of the private sector, it also says that:
the ambition of tech companies is matched by their proven ability to alter every facet of life Among the most significant challenges presented by the current trend of private, venture-funded technological development is the immense market, platform, and political power placed in the hands of private, profit-motivated individuals.
The paper argues that, by taking a bigger picture view of safe and responsible AI that reaches beyond individual harms and protections, its recommended governance framework stands a better chance than the existing AI regulatory frameworks in relocating “authority over technological futures from private individuals to public institutions”.
Conclusion
EU Commissioner Margrethe Vestager has warned that the “window of opportunity” to maximise the benefits of artificial intelligence is closing - that’s more of a political ‘call to arms’ than being entirely accurate given that AI regulatory frameworks will need to continually evolve as they scramble to keep pace with the technological developments. However, she is right in the sense that now is the time to resolve the normative framework within which to define safe and responsible AI going forward. While the Kennedy School paper can be as vague and elusive in its formulation of AI governance principles as the existing regulatory models it critiques, the paper adds the following important perspective to the discussion of this normative framework:
how we should govern this emerging technology is a chance not merely to categorize and manage narrow risk but also to construe the risks and opportunities much more broadly, and to make correspondingly large investments in public goods, personnel, and democracy itself.
The Kennedy School paper is authored by Danielle Allen, Sarah Hubbard, Woojin Lim, Allison Stanger, Shlomit Wagman, and Kinney Zalesne, of the Allen Lab for Democracy Renovation, Ash Center for Democratic Governance and Innovation.
Read more here: A Roadmap for Governing AI: Technology Governance and Power Sharing Liberalism
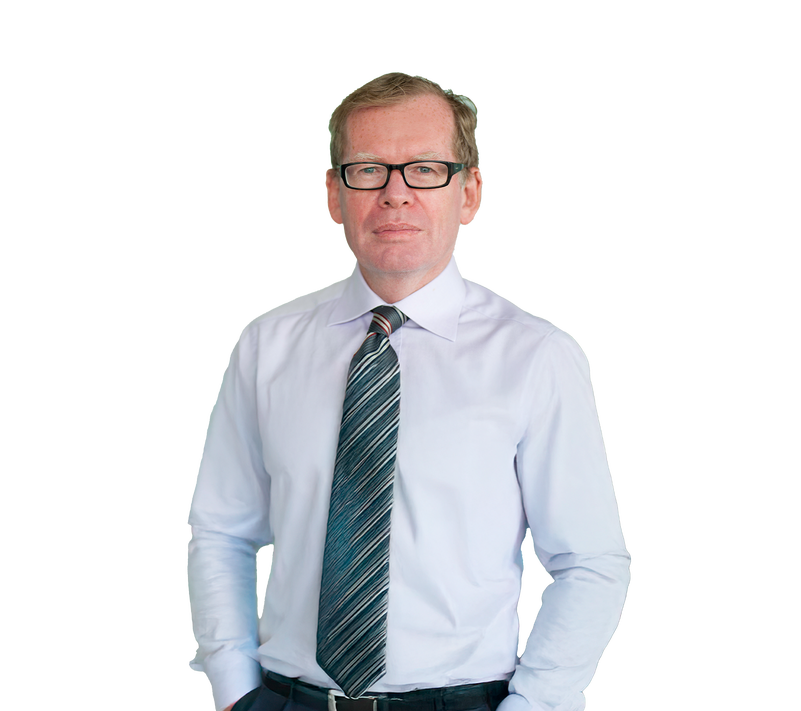
Peter Waters
Consultant