MIT recently conducted in-depth interviews on the future direction of ‘enterprise AI’ with chief information officers (CIOs) at some of the world’s largest and best-known companies, including Dupont, Adobe and Shell, as well as experts from the public, private, and academic sectors.
The big gear change
The most striking outcome from the MIT survey is the dramatic shift in CIO perceptions of the importance of AI to the future of their enterprises:
Although AI was recognized as strategically important before generative AI became prominent, our 2022 survey found CIOs’ ambitions limited: while 94% of organizations were using AI in some way, only 14% were aiming to achieve enterprise-wide AI by 2025. By contrast, the power of generative AI tools to democratize AI—to spread it through every function of the enterprise, to support every employee, and to engage every customer —heralds an inflection point where AI can grow from a technology employed for particular use cases to one that truly defines the modern enterprise.
As a result, CIOs are left scrambling (or in MIT’s more polite language, needing to ‘act decisively’) across a range of technology, operational, legal and governance issues:
..embracing generative AI to seize its opportunities and avoid ceding competitive ground, while also making strategic decisions about data infrastructure, model ownership, workforce structure, and AI governance that will have long-term consequences for organizational success.
So what does that look like?
Start here: recognise that AI is everywhere, all at once
The CIOs interviewed by MIT recognised that generative AI differs from how they have previously thought about AI/ML - and for that matter ‘traditional’ technology projects - in three ways.
First, back in 2022, CIO’s thought that AI adoption would be uneven across industries and between functions within enterprises. While 94% of organizations were already using AI in 2022 in some way, only 14% of them aimed to achieve enterprise-wide AI, and only in finance and in IT functions did more than half of the surveyed CIOs say AI would be critical.
But in this most recent MIT survey, the surveyed CIOs uniformly acknowledged that, “thanks to its flexibility and range, and user-friendly, natural language-based interface”, generative AI needed to be deployed enterprise-wide in order for the enterprise to seize and maintain competitive advantage, including in traditional, non-tech businesses.
Second, uniquely with generative AI, some of the most automation vulnerable sectors are in high-end technical and creative fields, including in technology teams themselves. But the CIOs did not consider that AI would result in job losses. The MIT-surveyed CIOs largely framed AI as “a co-pilot rather than a rival to human employees”. Adobe’s CIO remarked that “[g]enerative AI lets creators at all levels use their own words to generate content[b]ut I don’t think it will ever replace humans. Instead, it’s going to be an assistant to humans.”
Third, generative AI will mean “a trove of unstructured and buried data is now legible, unlocking business value.” Many businesses struggle with incompatible IT systems, often stretching back decades: earlier generations of IT that have not yet been retired; an odd assortment of IT systems have been inherited from acquired or merged businesses; proprietary technologies are not designed with interface with other third party systems. As a result, “important documents, from research and development intelligence to design instructions for plants, have been lost to view, locked in offline proprietary file types.”
One of the revolutionary things about generative AI is its ability to use unstructured data. Earlier version of AI/ML had to focus on use cases where structured data was ready and abundant - which meant that because of the complexity of collecting, annotating, and synthesizing heterogeneous datasets, wider AI initiatives were unviable. MIT observes that:
[b]y contrast, generative AI’s new ability to surface and utilize once-hidden data will power extraordinary new advances across the organization. LLMs, because they have the ability to ingest and process unstructured data, have the ability to browse in response to real time inquiries across these diverse databases.
Next go here: recognise that AI changes the relationship between users and technology
The MIT-surveyed CIOs recognised that generative AI, because it fundamentally transforms how ordinary users interact with technology, also will transform how technology teams plan, deploy and support technology with their businesses.
Generative AI will democratize access to technical capabilities once confined to a slice of the workforce. Dupont’s CIO reported that:
We have created communities of practitioners internally who do not necessarily have mainstream IT backgroundsWe want to empower our business users to craft their own dashboards and drive their own insights from data.
In this re-engineered framework between user and technology department, ideas for AI should come from the workforce, marking the start of a more self-service and entrepreneurial era within organizations. The Dupont CIO went so far as to predict that, with the emerging capability of generative AI to write code from plain English instructions, his job will be to ‘polish up’ code for new apps directly developed by non-technical users in the business.
Two key enablers
The MIT-surveyed CIOs identified two major changes needed to support enterprise-wide AI: a new approach to data infrastructure and a new approach to IT governance.
New approach to data
The new data approach is a called a ‘data lakehouse’, which MIT describes as follows:
They are a hybrid of two historical approaches— data warehouses and data lakes. Data warehouses came to prominence in the 1980s to systematize business intelligence and enterprise reporting. However, warehouses do not offer real-time services, operating on a batch processing basis, and cannot accommodate emerging and non-traditional data formats. Data lakes, favored for their ability to support more AI and data science tasks, emerged more recently. But they are complex to construct, slow, and suffer from inferior data quality controls. The lakehouse combines the best of both, offering an open architecture that combines the flexibility and scale of data lakes with the management and data quality of warehouses.
Because data warehouses do not cope well with unstructured data, such as log analytics, streaming, and social media data, they are not good sources from which to feed generative AI. By contrast, data lakes, with their looser ‘all in’ character, can store large amounts of structured and unstructured data in its raw, original, and unformatted form, and therefore are better suited to machine learning and predictive analytics. But, because the data is not ‘cleaned’ and organised, this can come at the cost of sub-optimal query performance when required for reporting and analytics use cases.
A data lakehouse attempts to get the best of both worlds. It allows the storage of raw data like a data lake, while also facilitating the option for pre-processed, structured data like a warehouse. This allows generative AI to feed on the whole of an enterprise’s data ‘to do its thing’, while specific pre-designed data analytics are still available to the enterprise.
The Shell CIO commented that the ability of the lakehouse model to support structured and unstructured data was crucial to his company: for predictive maintenance alone, Shell has 17,000 models and 4 trillion rows of data—adding another 20 billion per day from 3 million sensors across facilities around the world.
New approach to governance
The MIT survey comments that while unified governance was always a need, generative AI has raised the stakes and a renewed commitment to its importance was a common theme amongst the surveyed CIOs. The following comments by Abode CIO’s were representative of the views about the importance of including a wide range of voices throughout the AI oversight process at Adobe:
It’s important to have the element of diverse oversight through the whole process and to make sure that we have diversity not only of things like ethnicity, gender, and sexual orientation, but also diversity of thought and professional experience mixed into the process and the AI impact assessment.
This is not a plaintiff plea from ESG executives, but from CIOs trying to ensure successful implementation of AI within their enterprises.
Build or buy AI
While CIOs have often led the rush to cloud-based services, the MIT survey noted that “[t]oday’s CIOs and leadership teams are re-evaluating their assumptions on ownership, partnership, and control as they consider how to build on the capabilities of third-party generative AI platforms.”
While accessing “AI in the cloud”, given the size and complexity of generative AI, is highly attractive, the MIT-surveyed CIOs identified the following concerns with buy over build your own:
over-leveraging a general-purpose AI platform is unlikely to confer competitive advantage - because yours competitor are getting the same or similar answers from a common AI.
the risk of leakage of IP and competitive advantage to others. Says Michael Carbin, an MIT professor and adviser to MosaicML “[y]ou don’t necessarily want to build off an existing model where the data that you’re putting in could be used by that company to compete against your own core products.” Shell’s CIO said “[w]e can’t allow things like Shell’s corporate strategy to be flowing through ChatGPT.”
loss of control or agency over R&D. The MIT survey noted that users lack visibility into the data, weightings, and algorithms that power closed AI models, and the product and its training data can change at any time. This is particularly a concern in scientific R&D where reproducibility is critical.
inaccurate and unreliable outputs. The largest LLMs are, by dint of their size, tainted by false information online. Beyond that, DuPont’s CIO commented that “[i]t’s an interesting thing with ChatGPT: you ask it the same question twice and you get two subtly different answers; this does make science and innovation company people raise their eyebrows.”
Building LLMs at the scale of ChatGPT is beyond the resources and skills of most non-tech enterprises, even the global companies included in the MIT surveys. However, as we discussed last week , recent developments in generative AI has produced and successfully trained much smaller models: MIT professor Carbin says:
The reduction in complexity comes by narrowing your focus from an all-purpose model that knows all of human knowledge to very high-quality knowledge just for you, because this is what individuals in businesses actually really need. Much of this technology can be within the reach of many more organizations. It’s not just the OpenAIs and the Googles and the Microsofts of the world, but more average-size businesses, even startups.
Databricks, which sponsored the MIT survey, has released Dolly, named after the world’s first cloned mammal, which has just 6 billion parameters— less than 3.5% of the 175 billion used in GPT-3. Dolly is based on the LLaMA tool from Meta, is fine-tuned on “high-quality inputs crowdsourced from Databricks employees”, and can be further trained by a user to its requirements for less than $30.
Conclusion
The MIT survey concludes that, after a cautious, uneven start, “the most forward looking CIOs are moving decisively into this AI era.” The survey rounds off with a personal reflection from Dupont’s CIO:
People who went through the computer and Internet revolution talk about when computers first came online. If you were one of those people who learned how to work with computers, you had a very good career. This is a similar turning point: as long as you embrace the technology, you will benefit from it.
Read more: State of AI Report 2023
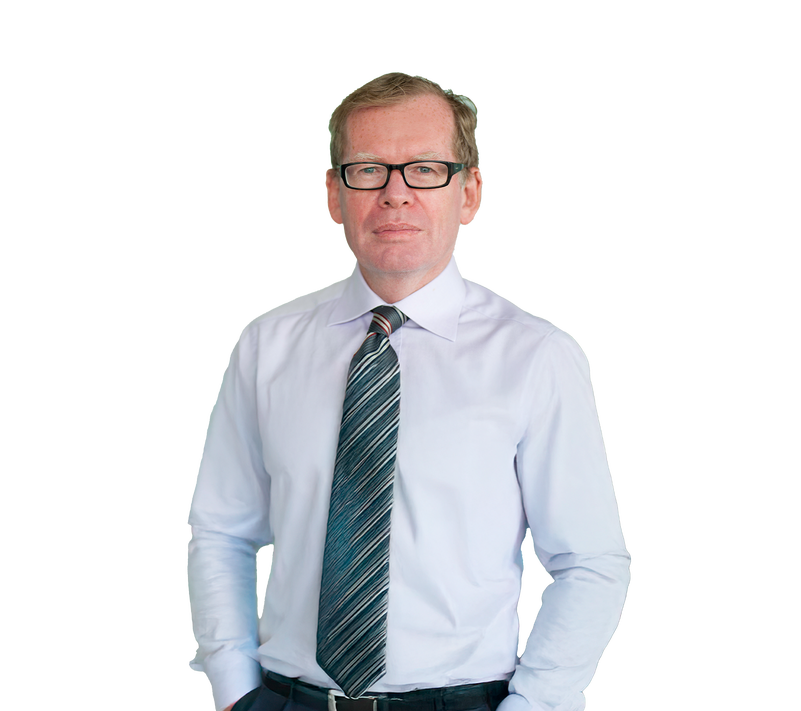
Peter Waters
Consultant