In a recent series, the New England Journal of Medicine (NEJM) set out to answer the question whether wearable digital health technology (DHT) was going to improve patient outcomes and clinical practice or is it just a trend that will pass, like so many consumer devices and their well-being claims? The editors noted that “wearable DHT is at an inflection point between fanciful descriptions and practical applications that are being woven into health monitoring, clinical diagnoses, and administrative approvals for new therapies”, which gives more than a hint about the answer they think the series gives to their question.
Case studies
Several papers in the series provide real world examples of examples of how wearable DHT is increasingly being applied in clinical situations today.
One paper (Hughes, Addala, Buckingham) described the advances in wearable technology for people living with type 1 and type 2 diabetes. Automated insulin delivery (AID) systems which can be worn on the body have been around for some time, but the early AID systems had many alarms and safeguards that created additional burdens for the patient. New ‘smart’ AID systems integrate communication between a wearable glucose sensor and wearable insulin pump and use an algorithm to automatically decrease or suspend insulin delivery for predicted hypoglycemia, increase basal insulin for predicted hyperglycemia, and, most crucially, the use of improved adaptive algorithms will deliver corrective doses for higher glucose values while accounting for previously delivered insulin. These automatic real-time decisions are made every 5 minutes.
The AID system also communicates with a cell phone to send data to the cloud, and the health care provider can remotely visualize integrated glucose and insulin delivery data, allowing identification of patterns that can be used to modify settings and behaviors.
These wearable algorithm-driven AID systems allow diabetes sufferers to live a more practical, flexible life, with improved sleep because the wearable system ticks away doing the monitoring and infusion work during sleep and by providing “a degree of forgiveness for late or missed meal boluses” [a bolus is a dose of insulin taken to handle a rise in blood glucose, like the one that happens during eating]. Wearable AID technology also has become straightforward enough that a recent study found that 81% of parents could be trained remotely to fit and supervise young children with diabetes wearing an AID.
But the authors also point to challenges with this wearable technology, including “unexpected insulin infusion-site failures, times when the accuracy of the glucose sensor is inadequate, requirements for frequent user input, issues with commercial smartphone compatibility, connectivity between devices, challenges in transmitting data to the cloud, and limitations in equitable access to diabetes technology.”
Another paper (Donner, Devinsky, Friedman) describes the breakthroughs in wearable technology for people living with epilepsy . One third of people with epilepsy have seizures despite medical treatment, and the risk of sudden, unexpected death from seizures is higher for a person living alone or sharing a household but not a bedroom. The US Food and Drug Administration has approved several mobile device applications for seizure detection. The user downloads and subscribes to the application on a smartwatch and pairs it with a mobile phone or tablet.
Much like fall detectors for the elderly, these applications use the movement and pulse rate sensors of the smartwatch to identify potential convulsive seizures and the linked mobile device to alert pre-identified caregivers. But some applications are much more sophisticated in identifying seizures by using electrocardiography and photoplethysmography to measure heart rate and pulse rate, electrodermal activity to measure sympathetic nervous system activity, and an audio recording device to detect and record seizure-associated sounds.
The success of these applications in detecting convulsive seizures ranges from 76 to 95%. However, a more inconvenient problem is that the rate of false alarms ranges, depending on the application, from 0.1 to 2.5 false alarms per day.
Current devices work also only for convulsive seizures and have not been shown to reliably detect other seizure types. The applications only detect convulsive seizures once they have gripped the person. The ‘holy grail’ for people living with epilepsy would be a wearable device which has the ability to predict that a seizure is imminent or is likely to occur within a number of hours, which then could allow for safety planning and even intervention with rescue medications. However, the authors note that the data collected from patients may “play a part in the development of prediction algorithms by identifying environmental and health factors associated with seizures that can be combined with biologic signal data from seizure-detection devices.”
Who's liable?
The million dollar question (quite literally) is who is liable when a medical professional’s treatment of a patient is “augmented” by reliance on DHT and something goes wrong.
In another paper in the NEJM series, the authors (Mello and Guhain) tracked down cases in the US courts in which software-related errors were alleged to have caused physical injury - they could find only 51 cases. These clustered into three broad situations:
harms to patients caused by defects in software that is used to manage care or resources. Typically, plaintiffs bring product-liability claims against the developer. For example, in one case, the court held that the plaintiffs had made a viable claim that a defective user interface in drug-management software led doctors to mistakenly believe that they had scheduled medication.
harms to patients when physicians adhere to erroneous software recommendations. Patients bring malpractice (i.e. negligence) claims alleging that the doctor should have ignored the recommendation or independently reached the correct decision. For example, in one case, doctors followed the output of a software program for cardiac health screening, which classified a young adult patient with a family history of congenital heart defects as “normal” on the basis of clinical test results. When the man died weeks later of a congenital heart condition, the family sued the physicians, alleging that they should have scrutinized the output of the software more closely and relied on their own interpretation of the tests. The court denied the defendant’s motion for summary judgment, based on the evidence that other specialists acting reasonably would have done the same thing.
harms to patients from apparent malfunctions of software embedded within devices, such as implantables, surgical robots, or monitoring tools. Plaintiffs typically bring product-liability claims against the developer, but these cases tend to fail because “[c]ourts are skeptical when plaintiffs frame the failure of the device as its defect and instead demand that they identify specific design flaws".
The authors found that, while the US has a reputation as ‘plaintiff friendly’ (our term, not theirs), patients face significant challenges in suing over DHT errors:
because software is intangible, courts have been reluctant to apply doctrines of product liability to it. This impacts the standard of care which has to be shown - product liability is much stricter than the negligence standard which applies to professional services like medicine.
the challenge of specifying the design flaw is even greater with AI than with software generally because, as many developers candidly acknowledge, we don’t quite know how generative AI actually works (euphemistically called ‘model opacity’).
plaintiffs alleging that complex products were defectively designed often are required to show that there is a reasonable alternative design that would be safer. But this is difficult to apply to AI:
AI models are essentially mathematical equations that encode statistical patterns learned automatically from data. Plaintiffs must show that some such patterns were ‘defective’ and that their injury was foreseeable from the patterns learned. However, because such patterns are represented with the use of up to billions of variables, identifying the patterns on which a system relies is technically challenging. Plaintiffs can suggest better training data or validation processes but may struggle to prove that these would have changed the patterns enough to eliminate the ‘defect. ’
Finally, and probably most tellingly, the authors posit that “[c]ourts also may have conceptual difficulty in deeming physicians and hospitals to be negligent for relying on models that, on average, deliver better results than humans alone".
A framework for assessing risk when using medical AI
The authors of the NEJM legal paper proposed a framework to support health care organizations and clinicians in assessing AI liability risk, depicted below:
While a little US-centric, it provides a useful guide in other jurisdictions.
source of the error: the health care organisation needs to look ‘upstream’ to understand how the developer has trained the AI, including to determine whether there might be biases (e.g. race) in the training data compared to its patient profile. The health care organisation also needs to look ‘downstream’ and think about how the AI will integrate into their clinicians' practices:
..will some clinicians be inclined to dismiss model recommendations because of engrained habits or distaste for AI? Research suggests that users’ trust in machine learning models predicts their willingness to adjust their judgments on the basis of model output.
opportunity for catching errors: The authors note that:
Research in human-computer interactions shows that decision makers who are assisted by computer models frequently overrely on model output, fail to recognize when it is incorrect, and do not intervene when they should, a phenomenon known as automation bias.
The authors observe that ‘the situational opportunity’ can be improved when AI tools are highly visible (i.e. when they and their warnings and guidelines are not easily forgotten), with clinicians having information about the model to help focus their vigilance such as the rates of false negative and false positive rates and patient-specific probability scores. But the authors also recognise the realities of the time-pressured environments in overburdened hospital systems, where the ‘situational opportunity’ is likely to be pretty limited.
how serious is the potential harm: technological advances in DHT seem to mean it is increasingly being pushed into dealing with the most critical, most challenging medical issues.
how likely it would be for injured patients to find legal redress: this is the most speculative part of the analysis because of, as the authors’ review of the US case law found, “the awkward adolescence of software-related liability”. They make the point that when the traditional requirements of showing causation collide with the opaqueness of many AI models there could be some paradoxical results:
Models with the highest risk of error may not pose the greatest liability risk because of the problems that plaintiffs may encounter in proving design defect. Higher-performing models in which errors are more easily identifiable to plaintiffs may involve greater liability risk than poorer-performing models in which the operation is more opaque.
The big, as yet unresolved issue is the allocation of liability between developers and medical users. The authors posit that “[h]ealth care organizations may face greater liability for situations in which errors are more likely to have resulted from human conduct or clinical integration than from erroneous output.”
However, that might understate the diligence which medical users should display in evaluating how AI models were trained before incorporating them into their health care environments.
Read more: Digital Technology for Diabetes
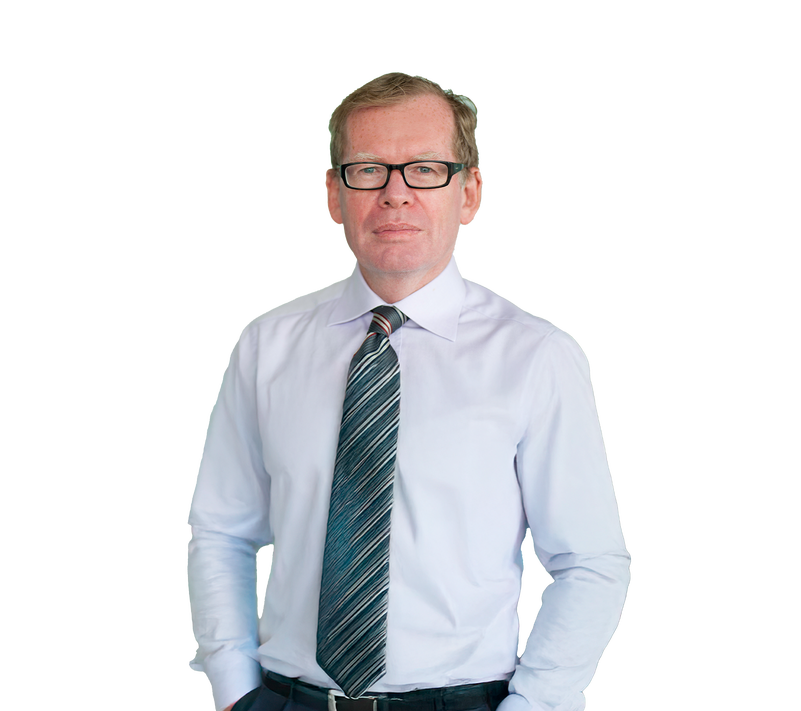
Peter Waters
Consultant