Andrew Moore, Google Cloud’s president, recently testified before a U.S. Senate Committee that defending AI systems from adversarial attacks is “absolutely the place where the battle’s being fought at the moment.”
Georgetown University’s Center for Security and Emerging Technology (CSET) recently convened an industry workshop to consider security threats posed to AI, with technology, legal, industry and policy attendees.
There were two somewhat disturbing outcomes from the meeting:
- while AI has been deployed quickly and widely into the real world, most of the learning about AI’s cybersecurity vulnerabilities still comes from testing in a lab environment. As such, while AI is ‘out there in the wild’, the CSET paper acknowledges “a holistic understanding of vulnerabilities in deployed systems is lacking.”
- Yet we know enough to know that “AI vulnerabilities may not map straightforwardly onto the traditional definition of a patch-to-fix cybersecurity vulnerability.” If you are introducing AI into your organisation, it would be a mistake to re-purpose your existing cybersecurity policies and teams without substantial rethinking, retraining and additional resources.
What’s so different about AI risks compared to traditional cybersecurity risks?
Simple algorithmic systems have been around for a while, and so, as the CSET report says, “malicious actors have been attempting to evade algorithm-based spam filters or manipulate recommender algorithms for decades.”
But there is a step change in level of risk, the scope of what we should consider as risk, and the consequences of risk.
The CSET report identified the following differences between AI vulnerabilities compared to traditional software vulnerabilities:
- “AI vulnerabilities generally derive from a complex interaction between training data and the training algorithm.” That is, the vulnerability may lie not in the algorithm itself but in the data it has been trained on. What’s more, that type of vulnerability may not become obvious until the AI is deployed or it may emerge in some real world settings in which the AI is deployed but not in others.
- rectifying an AI vulnerability will usually involve much more than downloading a “patch” such as from your mobile phone manufacturer. Rectifying a vulnerability in an AI model may require retraining it, which usually means taking it off line, or rectification may not even be feasible at all, which may mean users face a decision about whether to suspend or decommission the AI. Model retraining to reduce security vulnerabilities also may degrade overall performance on non-malicious system inputs, undermining the rationale for deploying the AI.
- vulnerabilities in AI systems may be “highly ephemeral”. As the genius of AI is its ability to continuously learn, organizations (the developer or the deployer) may use using continuous training pipeline where models are frequently updated with new data. This makes it difficult to pinpoint ‘what went wrong when’ and then how to work out how to untangle the error from the AI’s ‘learned experience’ given all the corrections the AI is making across its massive data bank (most of which humans cannot perceive). More disturbingly, how generative AI works can be opaque, including to its developers.
- Vulnerabilities also may be dependent on the context in which AI models are deployed, with organizations that deploy locally fine-tuned versions of a central model across many devices. This is particularly the case with generative AI which are designed to work across in the economy – sometimes in applications that the developers may never have imagined or tested. Attacks—but equally mitigations—may not transfer well across all versions of the model.
But probably the biggest difference with cybersecurity ‘lore’ identified in the CSET report is over what counts as a vulnerability of AI. First, the concept of a malicious attempt to distort AI may need to be revisited:
“adversarial examples are inputs to an AI system that have been perturbed in some way in order to deliberately degrade the system’s performance. But it is hard to distinguish between worrisome “attacks” and neutral—or even expected—user manipulations, like wearing sunglasses to make it harder for facial recognition systems to recognize someone.”
Second, while existing cybersecurity thinking ends to focus on technical vulnerabilities or programming gaps that allow hacking or manipulation of IT systems, the CSET report argues that aa much broader lens is needed when an organisation both assesses its AI risks and how to them:
“At a high level, we emphasize that…..the problems posed by AI vulnerabilities may be as much social as they are technological. Some of our recommendations emphasize opportunities for industry and government policymakers to promote AI security by investing in technical research. However, the majority of our recommendations focus on changes to processes, institutional culture, and awareness among AI developers and users.”
How to address AI risks?
The key recommendations put forward by workshop participants were as follows:
Organizations building or deploying AI models should use a risk management framework that addresses security throughout the AI system life cycle. While common sense for any good risk management framework, “AI vulnerabilities will present some unique considerations for risk management frameworks in mitigating risks.” Because fixing an AI risk involves more than a software ‘patch’, the developer and the deployer often will be faced more squarely with the dilemma about whether to risk trying to ‘re-teach’ the AI in a live environment or take it offline. This decision will involve more complex trade-offs than simply to ‘patch or not patch’, especially when AI models are a part of a complex system where the removal of one component may result in hard-to-predict changes to the overall system.
Researchers and practitioners in the field of adversarial machine learning should consult with those addressing AI bias and robustness, as well as other communities with relevant expertise. Again, building on the need for a broader lens on what is a ‘vulnerability’, workshop participants noted that AI vulnerabilities can be more analogous to other topics such as algorithmic bias than they are to traditional software vulnerabilities. Therefore, a wider range of skill sets will be needed to detect and mitigate AI risks. For example, “AI fairness researchers have extensively studied how poor data, design choices, and risk decisions have led to model failures that cause real-world harm.”
Organizations that deploy AI systems should pursue information sharing arrangements to foster an understanding of the threat. While not put this bluntly, there is a sense from the CSET paper that we are ‘flying blind’ on the extent and nature of AI vulnerabilities. It was noted that “many cybersecurity teams may not have all the relevant expertise to detect such attacks, organizations may lack the capability—and perhaps the incentive—to identify and disclose AI attacks that do occur.” Even if vulnerabilities are identified or malicious attacks are observed, this information is rarely shared with other peer organizations, competitors, other companies in the supply chain, end users, or government or civil society observers.
AI deployers should emphasize building a culture of security that is embedded in AI development at every stage of the product life cycle. The CSET paper observed that many machine learning libraries provide functions that, by default, prioritize processing speed and minor improvements in performance over security. The CSET paper that there was an emerging ‘science’ of ‘adversarial machine learning’ – essentially a ‘red team’ to stress test AI in the course of development. However, the report noted that ‘adversarial machine learning’ accounts for less than 1 percent of all academic AI research.
Developers and deployers of high-risk AI systems must prioritize transparency for consumers. This is perhaps the biggest shift from traditional cybersecurity thinking, which tends to be a little ‘secret squirrel’ in organisations. The CSET paper argues that the only safe assumption developers and deployers can make is that each AI model come with inherent vulnerabilities that will be difficult if not impossible to patch out there in the real world. Therefore, consumers and private citizens should generally be informed when they are being made subject to decision making by an AI model in a high-risk context. In addition, where the designers of a model make important decisions about relevant trade-offs— such as those that may exist between security, performance, robustness, or fairness— many workshop participants felt that these decisions also should be disclosed.
While these recommendations would require a big shift in traditional cybersecurity skills and decision making, the workshop participants did not think it was necessary to start with a blank page in addressing AI risks. They encouraged actively experimenting with extensions of existing cybersecurity processes to cover AI vulnerabilities, such as the Common Vulnerabilities and Exposures (CVE) system for enumerating known vulnerabilities, the Common Vulnerability Scoring System (CVSS) for evaluating the potential risk associated with known vulnerabilities, and the Coordinated Vulnerability Disclosure (CVD) process for coordinating between security researchers and software vendors.
But the message of the CSET paper is to do that successfully, today’s ‘cybersecurity ninjas’ will need to sit around the table with human rights advocates, AI fairness advocates and even ordinary consumers.
Read more: Adversarial Machine Learning and Cybersecurity: Risks, Challenges, and Legal Implications
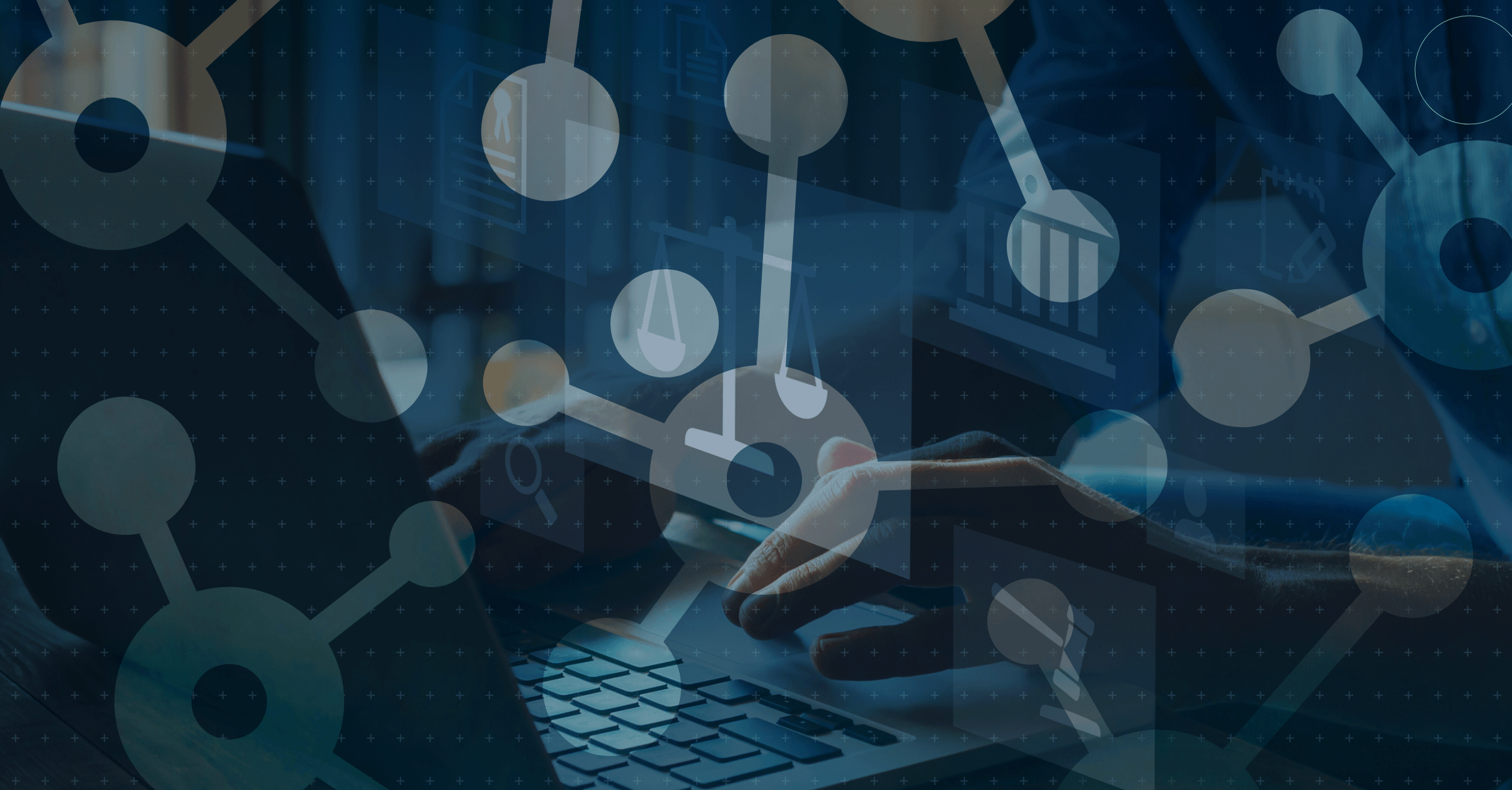
Visit Smart Counsel