The UK’s Competition and Markets Authority (CMA) has recently released one of the world’s first detailed analysis of competition in generative AI models (termed Foundational Models or FM).
The helicopter view
The CMA’s mud map of the FM supply chain is as follows:
An Economist article from early this year, Big Tech and the pursuit of AI dominance , reflects a commonly held concern amongst policy makers:
The tech giants have all they need—data, computing power, billions of users—to thrive in the age of ai. They also recall the fate of one-time Goliaths, from Kodak to BlackBerry, that missed earlier platform shifts, only to sink into bankruptcy or irrelevance. Their response is a deluge of investments. In 2022, amid a tech-led stock market rout, the big five poured $223bn into research and development,, up from $109bn in 2019... All told, this was equal to 26% of their combined sales last year, up from 16% in 2015.
The CMA, while acknowledging that potential outcome, also considers that there is potential for a positive market outcome for consumers, businesses and the wider economy in which:
..there were multiple independent firms competing with one another to produce leading FM models, with innovative firms able to access the inputs they need to enter, expand and compete effectively. In that scenario, firms would be able to experiment with different business models and forms of monetisation, including the supply of FMs on both an open-source and closed-source basis so others can continue to build on existing FM capabilities.
Could data be the ultimate source of AI dominance?
As depicted above, data comes into the development of AI at two levels:
Pre-training : At this level, hundreds or thousands of gigabytes of data are used to build the baseline knowledge of the model, commonly harvested from publicly available sources, although proprietary data increasingly is used to improve quality.
Fine tuning : an optional additional process that can be applied to pre-trained models with two objectives:
Alignment can ‘iron out’ potential bad behaviour, such as biases which are almost inevitably embedded or inferred by the FM from the vast lakes of public data used for pre-training. An FM also needs to be taught to how to better communicate with human users in ways we would expect (‘speak like a machine’).
Domain or task specific fine-tuning essentially trains a FM, pre-trained as general purpose AI, to specialise in a particular domain or task: for example, training a model to provide legal advice.
Pre-training data
The CMA considered that the sheer scale of the data needed to pre-train large FMs could militate against dominance because data at that scale is only available on the public internet.
But some argue that search engine firms have an advantage harvesting public data for two reasons: first, they have already have sorted and organised Internet data into web indexes to search engines to provide rapid results relating to user queries; and second, their search engines are more likely to circumvent anti-scraping technologies deployed by content providers which block or limit of activity of users, bots or applications that are deemed to be over-using or abusing a web page (called rate-limiting).
However, the CMA appears to be mildly sceptical of these arguments:
the alternative internet ‘harvesting’ technologies used by start-ups, such as the web crawler C4, “have similar utility for pre-training, particularly given that many high performing models have been developed without access to a web index.
considerable research and development effort is now being focused on finding efficiencies in new training methods and model architectures, which could “[a]ccess to large volumes of high-quality training data may confer less of an advantage if new methods to achieve increased performance, using fewer resources, emerge and become accessible to a range of competitors in the near term.”
It also has been argued that some firms, particularly social media and video content platform providers, may own or have easier access to, repositories of photos, videos, digital books, audiobooks, music, and podcasts. The CMA again appears mildly sceptical:
proprietary data is likely to be the smaller component of the data input compared to web-scrapped data. Lifting the overall quality of pre-training data is more likely to be achieved through better “filtering web scraped data to find high quality data..than adding data from proprietary sources”.
there are also many sources of proprietary data outside the digital content providers, such as academic institutions and traditional media and publishing companies, and “[i]t is not clear whether certain companies will necessarily have advantages in buying proprietary data from third party data owners, given that there are a range of FM start-ups that have been able to raise significant capital.”
Fine-tuning data
While fine-tuning data was more likely to be mainly proprietorial, the CMA considered that market power over this data at this level of the supply chain was less likely because:
the much lesser volume of data required for fine-tuning compared to pre-training is well within the reach of intermediaries and users: for example, using employees to generate example conversations user feedback data.
there is an emerging market of specialist data providers providing high quality labelled data for alignment purposes: estimated to be in the range of tens of millions of US dollars, might not be cost prohibitive to VC-backed start-ups.
Alternatively, fine-tuning data also can be crowd sourced: for example, Open Assistant Conversations is a human generated and annotated assistant-style conversation corpus.
fine-tuning of later FMs can utilise conversations between humans and existing models that have been shared online.
The CMA was fairly dismissive of artificially generated, called synthetic data, as a ‘silver bullet’ because of the risks of ‘model collapse’: existing models can contain defects which pollute future models.
Could control of computational power be the source of AI dominance?
The CMA was more concerned about control of the substantial computing power required to pre-train FMs than was over the data itself. OpenAI reportedly spent over $100 million to develop GPT-4.
The CMA considered that “most FM developers would not build the necessary computational infrastructure for pre-training due to the large upfront cost” and that if FMs continue to enlarge to stay competitive, then market concentration driven by control of computing power could tighten.
But even so, the CMA noted that there were commercial alternatives for access to large scale computing resources for FM development, mainly from the cloud service providers such Amazon Web Services (AWS), Microsoft Azure, and Google Cloud Platform (GCP). These CSPs had their own powerful incentives to enter into partnership arrangements with FM developers:
[t]he CSP can improve their offering by using the latest hardware as well as mitigate potential supply shortages, while the developer can get access to cutting-edge technology that can help them create more innovative applications.
Right on cue, Anthropic, an AI developer, has announced a US$4 billion partnership with Amazon .
Even so, the CMA cautions that things may not always play out evenly for smaller FM developers with the CSPs because larger FM developers “are more likely to get ‘first in line’ and make deals to hold larger compute clusters.”
The CMA discounted the availability of large scale computing resources, such as in universities, as an alternative because, outside the US (and probably the PRC), they were generally underpowered compared to privately own computing infrastructure and also dependent on uncertain grant funding from Government.
The CMA noted that the much smaller volume of data required for fine-tuning means that compute is unlikely to be a competition issue at that downstream level of the supply chain.
During their operating life, FMs may need to be re-trained a make a prediction based on new data, called inference. While a single inference requires very little compute, as the model size and/or number of users increases, inference at scale can require large amounts of compute.
However, the market seemed to be delivering FM users options for access to computing power for inferences. FM developers which directly deploy FMs models make available the computing infrastructure (through an API) to facilitate the inference process, as well as pay for the cost of computing it. Alternatively, some CSPs can provide FM inference (and fine-tuning) through their platforms and APIs: for example, the Amazon Bedrock API allows access to models from AI21, Anthropic, and Stability AI.
Questions around the other levers of dominance attributed to Big Tech
The CMA also considered that there were uncertainties around the applicability to FM of other levers of dominance typically attributed to Big Tech in earlier digital markets:
talent : the CMA noted that there had been a big shift from academia to industry: 65% of new AI PhDs were hired by industry in 2021, compared to 41% in 2011. But in its consultations, the CMA reported no concerns about non-compete clauses or publication restrictions imposed on employees during this review.
Financial clout : The cost of training and deploying FMs is significant, especially for pre-training. However, the CMA said that “[t]he evidence we have seen shows that currently smaller players are able to secure funding from investors.”
First mover advantage : the CMS commented that “[b]eing early to release major FMs does not guarantee success or the ability to capitalise on this advantage” because pre-trained open-source FMs may allow for new entrants to catch up quickly by benefitting from early movers’ work without having to invest the time and resources that they did.
Network effects : the CMA noted that stakeholder feedback that “once a FM completes its pre-training or fine-tuning, its performance level is essentially fixed, with the number of users having no immediate direct impact on user experience.” A large user bases may benefit from data feedback loops in fine-tuning and developing future models, but even then the size of the advantage is unclear “given that this data is currently not automatically ‘fed’ back into the model, but instead requires a rigorous manual review to ensure quality and safety.”
Potential sources of competitive constraints
The CMA noted that a large number of FMs have been deployed as open-source models, which means that the code and parameters of open-source models are publicly available:
The greater transparency of open-source models has several benefits. Users can have a better understanding of how the models work, which can help them to assess their accuracy and reliability. They can also modify the code of open-source models to improve them or add new features.
Some stakeholders argued that open-source pre-trained models are generally smaller and perform less well than the highest-performing closed-source models. However, the CMA thought that it “remains to be seen to what extent any such gap will be maintained over time.” The LLM leader boards published by Hugging Face shows rapid progression made by open-source models: by March 2023, the 13-billion parameter Vicuna LLM model claimed to deliver 90% of ChatGPT's quality.
But the CMA also noted there was uncertainty around the ability to commercialise open-source FM and therefore the willingness of investors to continue to fund their development.
The CMA queried whether ‘good enough’ FMs may be sufficient to compete in many sectors:
Currently the FMs at the cutting edge in terms of performance are those using vast amounts of inputs. However, it is possible that open-source or closed-source models will not need to achieve a comparable performance level to the highest performing models to act as a competitive constraint. For instance, certain tasks such as classifying customer reviews or generating text descriptions for products, among others, could be effectively accomplished with smaller models or those fine-tuned for the specific purpose.
The ability to compete at different levels of performance could lower barriers to entry because less data and less computer power would be required to develop and pre-train lower end FMs.
Third, the CMA also commented that a concentration in the development of pre-trained models may not necessarily exclude a diverse market for the fine-tuning of FMs. Lower requirements for compute to conduct fine-tuning may encourage developers to take advantage of “off-the-shelf” FMs and fine-tune them to their own, or a client’s needs. But the CMA noted that downstream competition could be undermined if FM development were to advance to the point where the most powerful models are highly effective for a wide range of tasks without requiring extensive customisation.
Conclusion
The CMA is no digital Pollyanna. However, while “[p]revious technology-driven markets have shown that network effects and switching barriers can lead to consolidation, weak competition, and a ‘winner-takes-most’ outcome” , the CMA’s analysis suggests that, given the nascent state of AI competition and the uncertainties around the direction of technology and commercial factors, it remains to be seen the extent to which FM markets follow this pattern.
In the last instalment of our series on the CMA report, we will examine the CMA’s principles to guide the evolution of FM markets.
Read more: AI Foundation Models: Initial Report
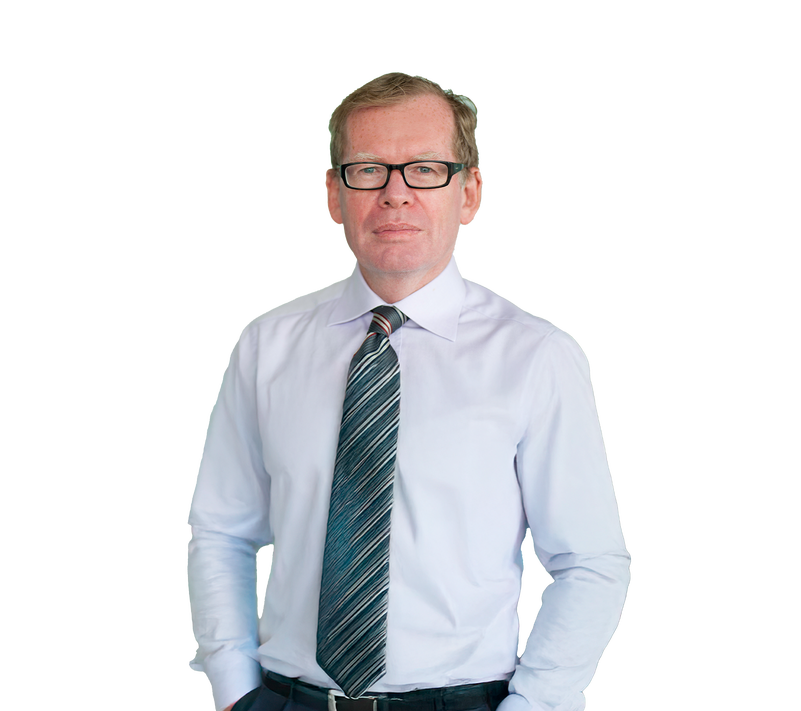
Peter Waters
Consultant