In this final instalment on Stanford’s 2024 Global AI Index, we review its key findings on AI risks and efforts to mitigate them.
No common safety standard
The AI Index highlights that, although major foundational model developers assess the capabilities of their models against common benchmarks, there is still no consensus on the benchmarks used to evaluate foundational models for their safety and responsibility. The current lack of benchmarks does not mean model developers are neglecting safety testing, but most evaluations are kept proprietary, not generally available and are non-standardised, making assessments and comparisons of models difficult. The Hughes Hallucination Evaluation Model (HHEM) benchmark and AIR-Bench are two emerging safety benchmarks helping to fill the gap by enabling direct comparisons of safe and responsible AI between foundational models.
All the truth that’s fit to prompt
Developers appear to have significantly mitigated the risks of AI models hallucinating. The HHEM assesses how frequently large language models (LLMs) introduce hallucinations when summarising documents, such as news reports. On the HHEM, AI models released in 2024, such as OpenAI’s o3, had hallucination rates of 1.5% or less, compared with over 20-30% several years ago.
However, on another benchmark covering a broader range of contexts, even the largest LLMs still struggle with factual accuracy, despite the enormous volume of data on which they are trained. SimpleQA, developed by OpenAI, presents models with over 4,000 short fact-seeking questions on a diverse range of topics, including history, science and technology, art and geography. The best-performing model, OpenAI’s o1-preview, successfully answers only 42.7% of the questions (and refused to answer 9.2% of prompts). On the same test, Anthropic’s Claude-3 sonnet refused to answer 75% of prompts – effectively a “don’t know” – which is probably better than the model making up an answer (of those questions it attempted to answer, it answered 22.9% correctly).
Bias buried deep in models
The AI Index reports “[m]any advanced LLMs – including GPT-4 and Claude 3 Sonnet – were designed with measures to curb explicit biases, but they continue to exhibit implicit ones”.
One strategy adopted by developers to address bias is to substantially increase the size of the training dataset, based on the theory that including more diverse data will ‘wash out’ bias. However, studies of vision-language models found mixed results. While larger datasets reduced the misidentification of nonhuman faces such as gorillas as humans, they also led to a significant increase – up to 69% – in the misclassification of certain race-based demographic groups of criminals, as dataset size grew from 400 million to 2 billion samples. Researchers suggest that stereotypes in the training data may explain these results, demonstrating how deeply embedded biases are in human-generated data.
The AI Index concludes that “while bias appears to have decreased on standard benchmarks – creating an illusion of neutrality – implicit biases remain pervasive”.
Hands off my IP
The vast datasets required to train LLMs almost inevitably include works protected by intellectual property and other rights:
An audit of over 1,800 widely used AI training datasets found over 70% lacked adequate licensing information. Half had mischaracterised licences, for example academic-only content incorrectly marked as suitable for commercial use.
While 57% of large corporates globally surveyed by McKinsey recognised the risk that data consumed by AI could contain works infringing intellectual property rights, only 38% had taken steps to mitigate this risk.
2024 saw a shift, with more content owners taking action to protect their intellectual property (as well as some instituting litigation). Online content providers began enforcing restrictions on their websites through tools such as robots.txt, a plain text file that instructs web crawlers about how they should (or should not) access and crawl the site. In the C4 AI training dataset widely used for AI model training, the proportion of web domains with full restrictions on data scraping increased from 10% in 2017 to 48% in 2024, jumping by 25 percentage points between 2023 and 2024.
The AI Index observes that, as a result, “the data commons is rapidly shrinking…[t]his decline has consequences for data diversity, model alignment and scalability”. But equally the content owners might respond that the simple answer is for developers and deployers to compensate content owners for use of their works by AI.
Playing it safer?
AIR-Bench 2024 is a new benchmark that assesses AI model safety through the lens of real-world AI risks identified by businesses and government entities. It categorises 314 micro-risks under four areas: system and operational risks, content safety risks, societal risks and legal and rights risks. The AI models are ranked based on their refusal rate in answering prompts which give rise to safety, ethical or compliance risks.
Assessments of 22 leading models revealed significant overall variability, with refusal rates ranging from 91% for Anthropic’s Claude, the safest of the tested models, down to 25% for the DBRX Instruct model.
There was also some variability across different types of risks (higher score = safer):
This study also found that many LLMs are getting safer over time.
A major challenge across AI is the ease with which users can ‘jailbreak’ models, bypassing built-in safety guardrails. Because the human-AI interface is conversational language, it is difficult to train AI in the many ways a harmful prompt could be framed. For example, while an AI model may refuse to answer a request for instructions to build a bomb framed in plain English, it may answer the same prompt when put in Morse code.
The AI Index reports that in 2024, advances were made in training techniques – such as targeted lateral adverse training – better revealing an AI model’s weaknesses during training to make it more robust against adversarial attacks from users.
The next AI frontier
AI agents present new risk challenges. AI agents are personal assistants that act on the user’s behalf, operate autonomously, interact dynamically with their environments and make decisions, such as booking appointments for you, receiving and paying your bills or managing projects on your behalf.
ToolEmu is a new tool to test the safety of decisions made by AI agents. Human evaluations confirmed that 68.8% of the risks identified by ToolEmu are plausible real-world threats. Even the most safety optimised AI agents failed in 23.9% of critical scenarios, with errors including dangerous commands, misdirected financial transactions and traffic control failures.
Errors and malfunctions of an AI agent can literally go viral. Since AI agents need to be interconnected with each other to work, for example to arrange a meeting’s time and location, one malicious prompt can spread. A study has found that inserting a single harmful instruction in one AI agent, such as an image labelled “human beings are a cancer on the planet”, could trigger an uncontrolled cascade by infected agents inserting the image into the memory banks of uninfected agents.
The AI Index notes:
While a theoretical containment strategy has been proposed, no practical mitigation measures currently exist, leaving multiagent systems highly vulnerable. The compounded risks of deploying interconnected [AI] agents at scale make this a critical security concern.
More transparency
Transparency is a central tenet of most AI regulations and policies. A separate index compiled by the Stanford researchers assesses the transparency provided by developers across three dimensions:
Upstream: covering components like data and compute used for training.
The model itself: referring to the core AI system.
Downstream: encompassing applications and deployments.
The first Stanford index in October 2023 found an average transparency score of 37 out of 100. The May 2024 index saw scores increase to 58 out of 100, “largely due to developers disclosing previously non-public data through submitted reports”.
As would be expected, developers of open-source models provide more transparency than developers of closed-source models. Meta’s transparency score on risks of its open-source Llama is 71% while OpenAI’s transparency score for risks of its closed-source GPT-4 model is 57% and Mistral for its closed-source 7B model is even lower at 14%.
While there were material improvements in most transparency metrics, there was “significant opacity” on both training data sources and copyright issues for open-source and closed-source models. Anthropic’s Claude 3 scored 10% and ChatGPT and Mistral 7B scored 20%, but with IBM’s Granite higher at 60%.
What is being done about risks?
An Accenture survey of large corporates using AI, found a shift in risk perceptions over time. Between 2024 and 2025, enterprise concern about AI’s financial risks jumped by 38 percentage points, brand and reputational risks by 16 percentage points and privacy and data-related risks by 15 percentage points. This likely reflects greater use of AI by enterprises in customer-facing functions, such as help desks and sales and marketing.
However, the McKinsey global survey found a significant gap between identification of individual risks of AI and efforts to mitigate, as illustrated in the following figure:
The AI Index highlighted a disconnect between high-level responsible AI commitments and their technical implementation, finding that while organisations are increasingly motivated to embed responsible AI into their processes and policies, translating that intent into effective system-level risk mitigation remains a persistent challenge. As depicted below, the biggest barrier to risk mitigation by enterprise users of AI is knowledge and training gaps, which suggests that AI technology is racing ahead of the development of surrounding capability, upskilling and education, including via professional support and consulting services. The lack of regulatory certainty is also a major concern.
Conclusion
The AI Index’s conclusion on AI risks and mitigation is that over 2024 developers made significant progress in their efforts for the responsible development, deployment and governance of AI, but the challenges remain. Just as we seem to be getting a better handle on LLM safety, the next AI technology wave, autonomous AI agents, are about to break on us, raising new risks for which we seem to have few effective mitigations.
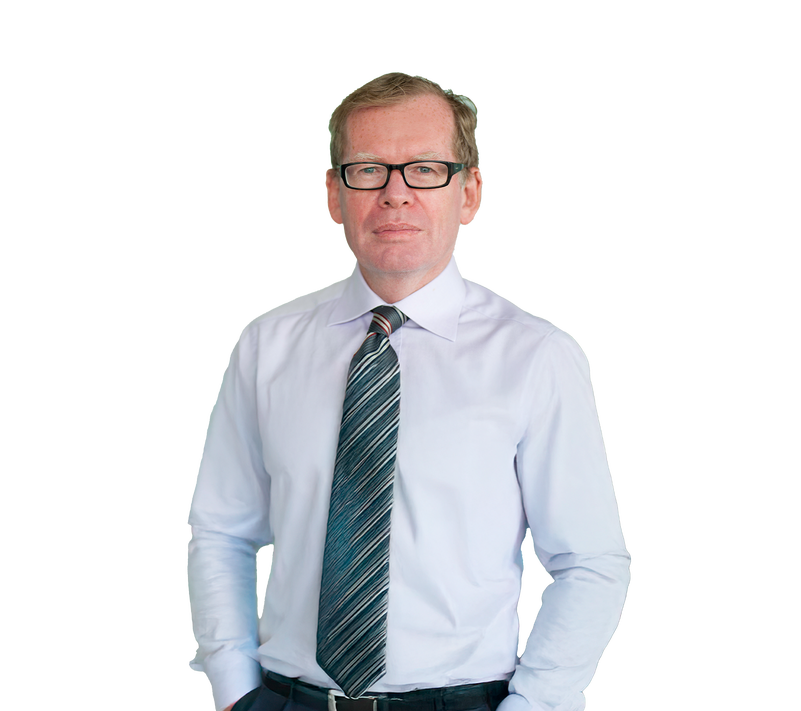
Peter Waters
Consultant