Generative AI, aptly named, has the ability to generate new content, be it text, images, music or even videos. Could generative AI be used to generate rules to regulate itself? As interesting as that may be, it would probably be better left to humans. Various strides have been taken, including in Australia ( Supporting responsible AI: discussion paper ); the USA ( ABC News: Several major tech companies agree to AI safeguards ); and the EU ( AI Act).
There is no doubt that generative AI is powerful tool. Even the AI industry itself says that regulation has an important role in keeping generative AI in check. A recent Netflix Black Mirror episode, Joan is Awful has provided a terrifying glimpse into the future of streaming involving AI (Spoiler: AI being used by ‘Streamberry’ to generate instant video content, tailored for each individual Netflix subscriber based on any of its subscribers’ lives).
The EU has attempted to lead the charge in regulating AI with the enactment of the AI Act. However, in a recent paper, three EU academics (Philipp Hacker, Andreas Engel and Marco Mauer) argue that the AI Act has been overtaken by generative AI even before comes into legal effect. This may serve as a cautionary tale in Australia’s own consideration of AI regulation Supporting responsible AI: discussion paper .
The Generative AI Value Chain
Hacker, Engel and Mauer set out a clear description of the participants in the AI value chain as the basis for their discussion of the allocation of responsibilities in any AI regulatory framework:
Developers : A developer is the entity that creates the AI, which involves pre-training on volumes of data and setting parameters so that the AI can recognise patterns and relationships in order to generate its output. Examples of this include OpenAI (creating ChatGPT) and Stability AI (creating Stable Diffusion).
Deployers : A deployer is the entity that substantially modifies the AI model for a particular use case and puts the AI model into service. An AI system may have multiple deployers, modifying it for different use cases.
Users : The user is the entity generating output by providing the AI model with prompts. Users may be using the AI model for personal or business purposes.
Recipient : The entity consuming the output prompted by the user.
The Fundamental Problem at the core of the EU AI Act
The EU AI Act in its current form is designed to place heavier regulatory burdens on AI systems the riskier they become. It identifies ‘high risk’ systems to include those that affect the health and safety or fundamental rights of persons. Before being introduced to the EU market, high risk AI systems are subjected to regulatory obligations such as human oversight, testing and registration in an EU-wide database, with the lion’s share of the regulatory obligations falling on the developer.
While that sounds a sensible calibration of AI risk, the problem posed by generative AI is how to capture a technology that is general in nature that can be used by deployers and users for almost any task, in any sector of the economy, often without the knowing, intending or having any reasonable foresight of its use. How does one then regulate for high risk applications without overregulating low risk applications? Or, more bluntly, should developers be subject to the requirements for ‘high risk’ AI because downstream deployers or users have used, or even could adapt, the AI for high risk activities?
During the legislative process, ‘patches’ were proposed in an attempt to update the EU AI Act for generative AI. The European Council AI proposed to capture generative AI with a new definition of ‘general purpose AI systems’, which means ‘an AI system that can be used in and adapted to a wide range of applications for which it was not intentionally and specifically designed’. Hacker, Engel and Mauer strongly criticise this definition as far too broad:
[Generative AI], in our view, must necessarily display significant generality in ability, tasks, or outputs, beyond the mere fact that they might be integrated into various use cases (which also holds true for extremely simple algorithms)According to [the proposed new definition], every simple image or speech recognition system seems to qualify, irrespective of the breadth of its capabilities.
The EU Parliamentary process produced a different definition and a proposed reframing of the obligations in the risk matrix for generative AI. The Parliamentary version defines foundation models as an AI system “that is trained on broad data at scale, is designed for generality of output, and can be adapted to a wide range of distinctive tasks”. Hacker, Engel and Mauer say that this is closer to the mark than the EU Council approach because the definition focuses on the generality of outputs and tasks. The Parliamentary version then applies a baseline of governance and transparency measures to all generative AI.
While welcoming the Parliamentary version as steps in the right direction, Hacker, Engel, and Mauer argue that the Parliamentary version ends up in much the same place as the Council proposal by, in practice, treating all generative AI models as high risk. This is because:
all foundation models also need to implement risk assessments, risk mitigation measures, and risk management strategies with a view to reasonably foreseeable risks to health, safety, fundamental rights, the environment, democracy and the rule of law, again with the involvement of independent expertswhile containing steps in the right direction, this proposal would be ultimately unconvincing for as it effectively treats foundation models as high-risk applications.
From a competition law perspective , Hacker, Engel and Mauer argue this potential for overregulation may stifle innovation and represents a barrier for new entities entering the AI industry, as only larger established players will have the resources to overcome the regulatory barriers:
Given the difficulty to comply with the AI Act’s [generative AI] rules, it can be expected that only large, deep-pocketed players (such as Google, Meta, Microsoft/Open AI) may field the costs to release an approximately AI Act-compliant [generative AI model]. For open source developers and many SMEs, compliance will likely be prohibitively costly. Hence, the AI Act may have the unintended consequence of spurring further anti-competitive concentration in the [generative AI] development market.
A Solution?
Hacker, Engel and Mauer propose that different regulations should apply to the different actors in the generative AI value chain, and that instead of regulating generative AI itself, there should be a shift towards regulating based on use cases of generative AI.
Compared to the ‘patched’ AI Act solution for generative AI, the regulatory burden should shift away from the developer to deployers and users. Where a deployer puts into service, and user puts to use, generative AI for a high-risk use case , it would be more practical to increase the regulatory burden on those actors rather than the developer who, at best, would have to predict the potential high risk use cases their generative AI could be used for. The increased regulatory burden includes comprehensive risk assessments and audits of datasets before the AI is put into service, increased reporting requirements and appropriate human oversight. It is not a ‘set and forget’ regulatory burden.
We have depicted the proposed approach as follows:
Specifically, Hacker, Engel, and Mauer propose:
There are minimum standards that all generative AI models should be subjected to. However, the appropriate actor that should be responsible for those minimum standards differs depending on the minimum standard itself. The minimum standards are:
Transparency over the provenance and curation of the training data is reported on. Users should understand how the generative AI was trained, the performance metrics used, and strategies to mitigate harmful content. Both the developers and deployers would be the appropriate actors as they both play a role in building or modifying the generative AI.
Transparency over the use of generative AI. A recipient should know which parts of the output they are receiving were generated using, or adapted from, AI. The user of AI is best place to disclose how they used generative AI in providing the output to the recipient. considers that this obligation should be limited to professional users, perhaps those using generative AI in trade or commerce. This would mean that a news article written using generative AI and published on a website would need to be disclose that generative AI was used. But a birthday invitation that was designed by a generative AI user and sent to the prospective guests would not need to disclose that generative AI was used in the preparation of those invitations.
Non-discrimination obligations. This relates to ensuring that AI models are trained with data that has been curated to be balanced and representative of protected groups. As the developer is responsible for the training, non-discrimination should primarily be their responsibility, although the deployer should play a supporting role.
Content moderation. Generative AI has the potential to be used in creating fake news or harmful speech. Like the content on more traditional social media networks, content produced from generated AI needs to be moderated. The responsibility should belong to developers and deployers to moderate output and audit their models, and assist with notifications such as trusted/authentication markers.
Data governance, cybersecurity, privacy and sustainability. The authors do not elaborate so much on these aspects, but also considers them as too important to be delegated downstream based on use cases. These aspects should be dealt with upstream by developers.
Regulation should allow for mandated collaboration between the different actors in the generative AI value chain. Hacker, Engel and Mauer recognise that as you go downstream in the value chain, the level of expertise decreases. Collaboration would allow actors to leverage expertise further upstream in fulfilling that actor’s regulatory responsibilities. There is a competition law risk in collaboration between these entities, especially if entities are vertically integrated in the value chain. However, this is not an unfamiliar problem for competition lawyers to navigate. The authors also recommend drawing from other areas of law relating to access to information, such as a litigation discovery process.
Conclusion
Self-evidently, AI presents uniquely difficult challenges, but are technology-specific laws and regulators the right tools? As Hacker, Engel and Mauer point out, a true-ism of public policy is that technology-specific laws can quickly become overtaken by the very technology they are designed to address.
But perhaps a greater, less articulated danger of technology-specific laws is that they become the domain of technologists - the laudable public policy goals become wrapped in the opaqueness of the technology itself. Surely AI, of all the technologies with which we have had to come to grips with, requires a broader focus, a more human-centric approach.
This suggests that the place to start in AI regulation is to focus on the output or the content of AI, not the technology. On this approach, we would see how far existing laws which express societal values, such as non-discrimination laws, can be applied; and then consider the extent to which unique features or risks of AI require specific measures. Such AI-specific measures might include the transparency, governance and mandatory collaboration measures proposed by Hacker, Engel and Mauer, or enacting the ‘water-marking’ AI content and other proposals from the recent White House brokered voluntary code.
Read more: ACM Digital Library | Regulating ChatGPT and other Large Generative AI Models
KNOWLEDGE ARTICLES YOU MAY BE INTERESTED IN:
AI Regulation in Australia: A centralised or decentralised approach?
Buy Now Pay Later - Preparing for tougher regulation
The impact of AI is getting very serious, very quickly
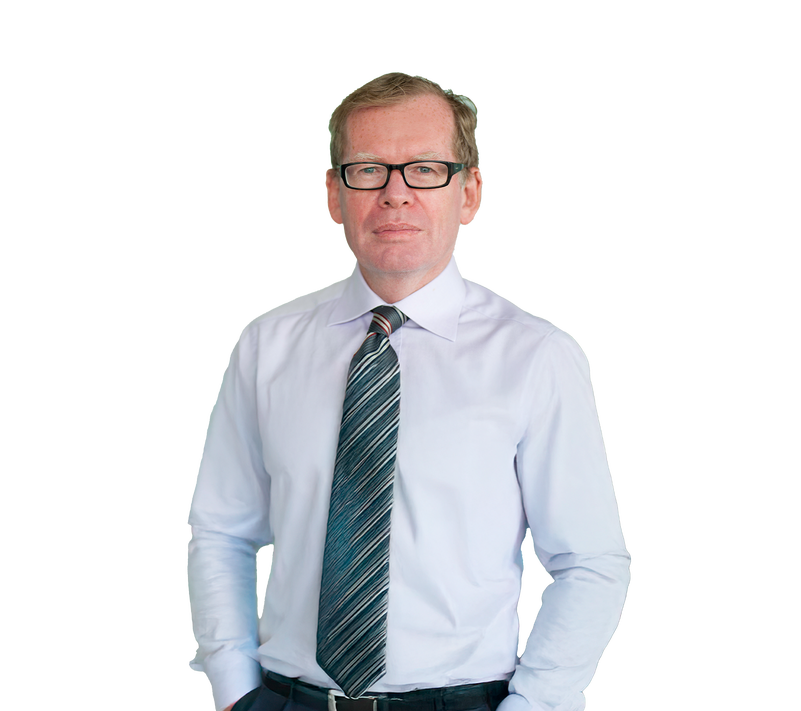
Peter Waters
Consultant