The Robodebt scheme did such damage because it used a simplistic data-matching algorithm with no human oversight. While the Robodebt Royal Commission acknowledged the increasing role of automated decision making in government, it also warned of the potential consequences of more sophisticated programs:
“While the fallout from the Robodebt scheme was described as a “massive failure of public administration,” the prospect of future programs, using increasingly complex and more sophisticated AI and automaton, having even more disastrous effects will be magnified by the “speed and scale at which AI can be deployed” and the increased difficulty of understanding where and how the failures have arisen. It is not all doom and gloom: when done well, AI and automaton can enable government to provide services in a way that is “faster, cheaper, quicker and more accessible.” Automated systems can provide improved consistency, accuracy and transparency of administrative decision-making. The concept of “when done well” is what government must grapple with as increasingly powerful technology becomes more ubiquitous.”
Given the scathing findings of the Robodebt Royal Commission, Australia needs a new ‘settlement’ between its governments and citizens on the use of data by government.
Some guidance on what this might look like comes from a recent report from the UK’s Alan Turing Institute.
Back to the Future for Government
The Turing paper argues that recent crises, such as the global financial crisis and the COVID pandemic, demonstrate that government decision-making processes are ‘inherently fragile’ but that:
“[m]odern data-intensive technologies, such as data science and artificial intelligence (AI), hold tremendous potential to rebuild resilience in government. However, changes are needed in order to realise this potential.”
The Turing report lays much of the blame for the current fragility of government institutions at the feet of neo-liberal politics (although it does not use this term).
A leading public policy thinker, Christopher Hood, identified three overlapping values that underly public or administrative decision-making:
- Economy and leanness of purpose: where the standard of success is frugality of resource use and where “the central concern is to ‘trim fat’ and avoid ‘slack’”.
- Resilience and robustness: where the standards of success are reliability and adaptability, and where “the central concern is to avoid system failure, ‘downtime’, [and] paralysis in the face of threat or challenge”.
- Fairness and honesty: where the standards of success are public trust and confidence and “the central concern is to ensure honesty, prevent ‘capture’ of public bodies by unrepresentative groups, and avoid all arbitrary proceeding”.
The level of control governments take of the economy during war (and potentially in response to climate change) is example of government intervention that addresses resilience and robustness. The emergence of the welfare state in countries like the UK and Australia after WWII is an example of government action to address unfairness and inequity, which often can flow from the events that required action to avoid ‘system failure’.
However, the Turning report argues that over the last few decades:
“..many western governments changed focus, prioritising economy and leanness of purpose over all other administrative values. The dominant paradigm for administrative reform at this time was New Public Management (NPM), which aimed to transfer management practices developed for business to the public sector. In countries that pursued NPM enthusiastically, organisational capacity was deliberately reduced: ‘slack in the system’ was diminished or eliminated, which, in turn, eroded resilience. .. the disaggregation of government departments reduced their ability to address problems spanning multiple sectors or to make coherent policy in a globally connected world.”
Hence, the twin crisis in the Australia polity of the cruelly flawed welfare entitlements decision-making identified by the Robodebt Royal Commission and concerns some have expressed about overuse of consultants as revealed by the PwC affair.
The Turing paper argues that the time has come for governments to return to the refocus on the values of resilience and fairness which characterised government decision making in the post-war period, but now with the benefit of harassing the radically different technology available today.
Private and public data analysis solves different problems
The political fixation of governments on efficiency was reflected in how they used technology. The Turing paper cautions that any attempt at the macro policy level to refocus government on resilience and fairness will be undermined without “a new and distinctly public sector approach to data science”.
This is because the problem advanced technology is being used to solve in the private sector is very different to the problem to be solved in the public sector:
“the private sector has made rapid progress in using data science to solve hard problems. This work has typically focused on using tools such as deep learning to complete well specified, yet difficult and time-consuming tasks that traditionally required human intelligence.”
whereas, the wicked problems in the public sector involve:
“trade-offs for which there may be no “correct” answer. For instance, how to allocate resources for climate change adaptation in an uncertain future, or how to reduce hospitalisation rates in an epidemic while protecting the economy.”
which means that:
“..the primary aim of public sector data science should not be to replicate human capabilities or to reduce costs but to boost governments’ expertise by collecting, linking and modelling heterogeneous data, and mediating between different areas of human expertise.”
How to build a better data science framework for governments
The Turing report identifies two starting points for the design of a more effective data approach for governments.
First, while post pandemic ‘resilience’ is at the top of the list for policy makers, you cannot divorce it from ‘fairness’:
“..the values of fairness and honesty (or transparency) are crucial to avoiding a situation where inequality threatens government stability and resilience. So, as we build the foundations for more resilient government using data science, we must provide the ethical guardrails to ensure that data science is used in safe and ethical ways.”
Second, these ethical guidelines cannot be developed in isolation from the citizens who will be affected by decisions made using the data analysis:
“..a citizen-focused approach to data science [is needed], which directly involves the people affected by use of data science technologies in the process of shaping standards to guide their safe use.”
Similar principles are proposed by the Robodebt Royal Commission.
What effective government data science tools would look like?
First and foremost, as problems in the public sector will involve trade-offs for which there may be no “correct” answer, the data analysis models should be more focused on better understanding causality than trying to predict the likelihood of a particular outcome. While the Turing paper acknowledges that “[t]his is a challenge at the forefront of modern statistics and machine learning”, it would have two benefits for public policy making:
- better enables ‘what if’ scenarios which juggle competing policy considerations in different configurations; and
- policy makers are more likely to be able to follow the logical chains and interpret the outputs than is the case with a ‘black box’ architecture for an algorithmic model, such as with generative AI.
Second, as shocks are unpredictable in both timing and what they will be about, governments need to be able to access an in-place set of “tools, methods and technologies that will allow them to intervene efficiently to promote stability.”
The Turning paper makes the following suggestions on what these pre-build tools might look like:
- agent computing: this approach creates ‘agents’, representing individuals, who explore a network or ‘world’. For example, agent-based models are capable of simulating people’s movements across entire labour markets in granular detail. While useful simulation tools were built during the pandemic, the Turing paper notes that they were typically developed in haste, were fraught with uncertainties and were often narrowly focused on particular issues, rather than being adaptable across government.
- digital twins: is a virtual representation of a physical system or process that is continually updated from data collected via monitoring of the real system. While currently used in emergency events planning, the Turing paper notes that new tools and approaches would be needed to enable digital twins to capture more complex socio-economic environments and to operate at scale.
- collective or ‘ensemble’ modelling: where different models, each of which may have its limitations, are brought together to solve a complex problem (i.e. the sum is more powerful than each individually). This approach has been used for predicting climate change.
- transfer learning: a model build from a data abundant source is applied to in a data scarce environment: for example, a health-related model built using data in one country with good centralised health records is used in another country which collects much less data.
Third, in probably the biggest challenge for a bureaucracy, the Turing paper underlined the importance for resilient policymaking of working across traditional boundaries and sharing insights between domains. In a none-too-subtle plug for institutions such as itself, the Turning paper called for:
“The design, development and implementation of effective tools and models for resilient policy-making must be guided by strong, interdisciplinary collaborations between decision makers and experts from across multiple different domains – from mathematicians and data scientists to ethicists and social scientists. Such interdisciplinary working can be incentivised by investing in collaborative spaces that sit outside the bounds of traditional academia.”
Conclusion
The punchline of Turing report is that, in applying advanced data science techniques, governments must stop mimicking private sector management practices. We should recognise that there is a fork in the road between private and public sector use of data:
“..rather than focusing on reducing costs through automation of what humans can already do well, data science reforms [for government] should focus on doing what humans cannot do well: addressing difficult, interconnected problems with many possible solutions through the harmonisation of data, knowledge, and expertise from different domains.”
Read more: Building resilience in government using data science
KNOWLEDGE ARTICLES YOU MAY BE INTERESTED IN:
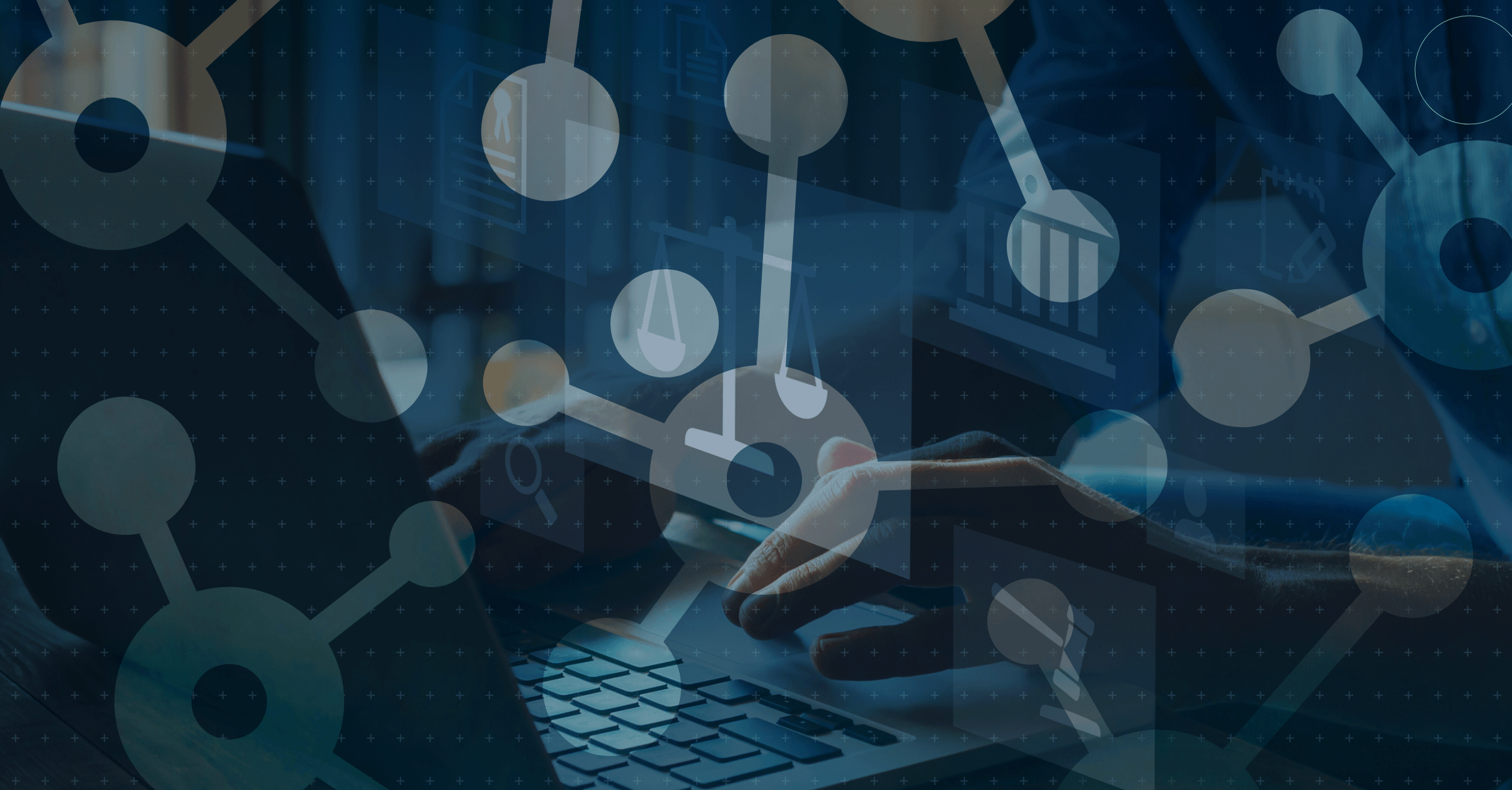
Visit Smart Counsel