COP 26 Glasgow is expected to agree an intergovernmental roadmap on how AI can be used to address climate change (a discussion session will be held Monday 8 November).
Other efforts to find innovative AI solutions to tackle climate change include the A.I. Moonshot Challenge, a €500,000 prize offered by a consortium of scientific bodies lead by the European Space Agency.
So, what can AI do on climate change? How much can it help limit temperature rises to 1.5 degrees?
First, the bad news
ICT is a major contributor to greenhouse gas emissions. A recent paper co-authored by the ‘father of the Internet’, Mike Berners-Lee, found that the ICT’s carbon footprint, when taking account of the full life cycle from development, manufacture, operation and disposal, was 2.1%–3.9% of global greenhouse gas (GHG) emissions in 2020, compared with the ‘bad boy’ of climate change, aviation, at 2%. About 30% of ICT’s emissions come from embodied emissions (i.e. the manufacturing process) and 70% from use. While computing devices have become much more energy efficient, the paper expressed concern that we may have reached the outer limits of what could be achieved:
“efficiency improvements might be coming to an end…As transistors have shrunk in size and increased in speed, they have begun to heat up; this led to manufacturers putting a speed limit on processing in 2004. The problem now is ‘‘quantum entanglement’’ where transistor layers become so thin that electrons jump between them, making transistors increasingly unreliable.”
AI is an ‘energy glutton’ given the complexity of training and inferencing on big data, and especially deep learning. Researchers have estimated that 284,019 kg of CO2e are emitted from training just one machine learning algorithm for natural language processing, an impact that is five times the lifetime emissions of a car.
AI as a positive force the climate
But AI also has been described as the ‘Swiss army knife’ of climate change solutions because of its potential multifunctional roles, including in measuring and reducing emissions; enabling innovative business models to help the climate; and improving resilience to climate hazards.
A seminal paper by prominent scientists and engineers from leading organizations and universities, including MIT, Cornell, Stanford, DeepMind, Google AI and Microsoft Research, sets out tangible ideas on how machine learning can be used to tackle climate change, and some proposals are as follows:
In energy:
- AI can be used to optimise demand forecasting of electricity and related predictions of sources like sunlight, wind, etc. This can be achieved by using past power consumption datasets and weather information. Hyperlocal weather modelling by AI can be used to monitor and adjust the positioning of solar panels and wind turbines to maximize power generation.
- AI can better manage an electricity grid which is becoming hugely complex with different forms of generation (solar, wide, total, geothermal, stored hydro and remnant fossil fuels). At the other end of the scale, AI can better manage small microgrids connecting a cluster of houses in a local area which are even harder to balance than national grids.
- AI provides consumers with their own management tools. Google, by utilising electricityMap– an AI-powered platform that shows in real-time how clean electricity is around the world and provides past, current and forecasted carbon footprint data for electricity by country –manages to align computing tasks with times of low-carbon electricity supply in the grid and, thus, reduces CO2e emissions from electricity consumption.
In logistics:
- In transport, which accounts 25% of GHGs, AI can be used to reduce the use of transport vehicles by optimizing the flow of vehicles, provide more efficient navigation, and facilitate shared transport.
- Supply chains are complex and generate a lot of data – even more so now as they become heavily populated with IoT devices. Much of this data is unstructured or in many different formats, and AI can sort through the data, find the relationships, predict before repair events happen and ensure a more efficient – and less energy intensive – supply chain.
In materials innovation, AI can dramatically cut development time. A new range of high performance, high quality materials will be needed to capture, store and transmit energy more efficiently and to replace materials we currently use in everyday life that generate a high level of GHGs in manufacture. The time frame for discovering new materials is remarkably long, typically approximately 10–20 years from initial research to first use because “significant progress tends to require a combination of chemical intuition and serendipity.” ML techniques can automate this process by combining existing heuristics with experimental data, physics, and reasoning to apply and even extend existing physical knowledge. For instance, ML and AI are being used to figure out a proposed material’s crystal structure, with the goal of accelerating materials discovery for solar fuels.
Cases studies - from opposite ends of the scale
At the micro-end of the scale is Trashbot. The efficiency and value of recycling is undermined by poor – or lazy – decisions we make when choosing the coloured bin into which to put our garbage. Paying human workers to dig through and re-sort mountains of garbage once collected is expensive, time consuming, messy and dangerous.
The efficiency and value of recycling is undermined by poor – or lazy – decisions we make when choosing which coloured bin to put our garbage in. Paying human workers to dig through and re-sort garbage is expensive, time consuming, messy and dangerous.
Instead of multiple openings, the Trashbot bin presents people with a single opening into which to deposit their garbage, whatever it is. Once you place garbage into the Trashbot single opening, cameras and sensors quickly analyse the type of discarded item. The item is weighted on a Teflon-coated plastic shelf and liquids are drained if any. The AI decides whether the item is destined for the landfill or recycling facility and sends it into the appropriate internal bin within Trashbot.
At the macro end of the scale is IceNet, an AI which predicts changes in the Artic ice pack. Near-surface air temperatures in the Arctic have increased at two to three times the rate of the global average. The September sea ice is now around half that of 1979. Climate simulations project the Arctic will have no sea ice in the summer of 2050, some say as early as 2030.
There is evidence that the extent of the Artic ice pack impacts temperatures well beyond the Artic, even down to mid-latitudes. Better prediction of Artic ice patterns across seasons could improve weather forecasting in other areas.
But predicting sea ice fluctuations is very difficult because sea ice is dynamically and thermodynamically coupled both to the atmosphere above and the ocean below: “current operational sea ice forecasting systems, based on deterministic coupled atmosphere-ice-ocean models, are often no better than simple statistical forecasts at seasonal lead times of two months and beyond.”
Deep learning systems like IceNet are adept at learning nonlinear statistical relationships between input and output data. IceNet extends the range of accurate forecasts, exceeding current state-of-the-art performance at 2- to 4-month lead time forecasts for August, September and October. Once trained, IceNet runs over 2000 times faster on a laptop than the current statistical models running on a supercomputer, taking less than ten seconds on a single graphics processing unit. IceNet is particularly adept at predicting the ice edge, which could reduce shipping hazards.
IceNet also has a potential role in conservation. One of the tragic outcomes of melting ice is ‘mega haul-outs’ of Pacific walrus, occurring when tens of thousands of walrus are forced to congregate on land due to a lack of sea ice to rest on. Human disturbances can cause stampedes at haul-out sites and lead to high walrus mortality. Information from IceNet’s forecasts, combined with known haul-out locations, could anticipate these events and help to prevent stampedes by managing human access.
How much of a difference can AI make?
A PwC study commissioned by Microsoft concluded that using AI for environmental applications has the potential to boost global GDP by 3.1 – 4.4% while also reducing global GHG emissions by around 1.5 – 4.0% by 2030 relative to business as usual. AI applications in energy (up to -2.2%) and transport (up to -1.7%) have the largest impact.
Those figures sound modest, but applied globally, the savings in GHGs are equivalent to the 2030 annual emissions of Australia, Canada and Japan combined.
However, as significant as AI could be in addressing climate change, the ML experts paper referred to above cautions:
“We emphasize that machine learning is not a silver bullet. The applications we highlight are impactful, but no one solution will “fix” climate change. There are also many areas of action where ML is inapplicable….. Furthermore, technology alone is not enough – technologies that would reduce climate change have been available for years, but have largely not been adopted at scale by society. While we hope that ML will be useful in reducing the costs associated with climate action, humanity also must decide to act.”
KNOWLEDGE ARTICLES YOU MAY BE INTERESTED IN:
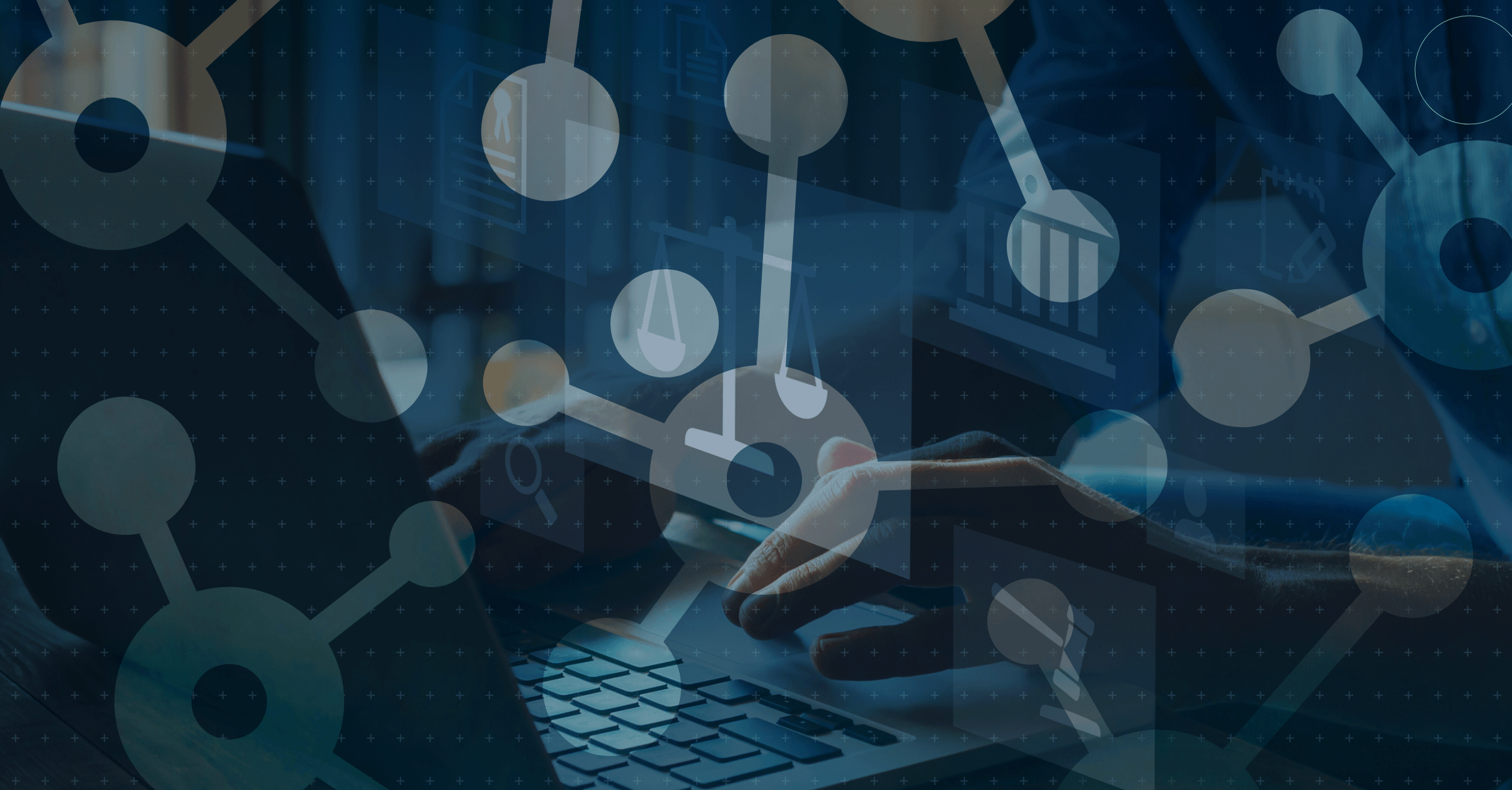
Visit Smart Counsel